Hydrus: Improving Personalized Quality of Experience in Short-form Video Services
PROCEEDINGS OF THE 46TH INTERNATIONAL ACM SIGIR CONFERENCE ON RESEARCH AND DEVELOPMENT IN INFORMATION RETRIEVAL, SIGIR 2023(2023)
摘要
Traditional approaches to improving users' quality of experience (QoE) focus on minimizing the latency on the server side. Through an analysis of 15 million users, however, we find that for short-form video apps, user experience depends on both response latency and recommendation accuracy. This observation brings a dilemma to service providers since improving recommendation accuracy requires adopting complex strategies that demand heavy computation, which substantially increases response latency. Our motivation is that users' sensitivity to response latency and recommendation accuracy varies greatly. In other words, some users would accept a 20ms increase in latency to enjoy higher-quality videos, while others prioritize minimizing lag above all else. Inspired by this, we present Hydrus, a novel resource allocation system that delivers the best possible personalized QoE by making tradeoffs between response latency and recommendation accuracy. Specifically, we formulate the resource allocation problem as a utility maximization problem, and Hydrus is guaranteed to solve the problem within a few milliseconds. We demonstrate the effectiveness of Hydrus through offline simulation and online experiments in Kuaishou, a massively popular video app with hundreds of millions of users worldwide. The results show that Hydrus can increase QoE by 35.6% with the same latency or reduce the latency by 10.1% with the same QoE. Furthermore, Hydrus can achieve 54.5% higher throughput without a decrease in QoE. In online A/B testing, Hydrus significantly improves click-through rate (CTR) and watch time; it can also reduce system resource costs without sacrificing QoE.
更多查看译文
关键词
personalized quality of experience,resource allocation,short-form video services
AI 理解论文
溯源树
样例
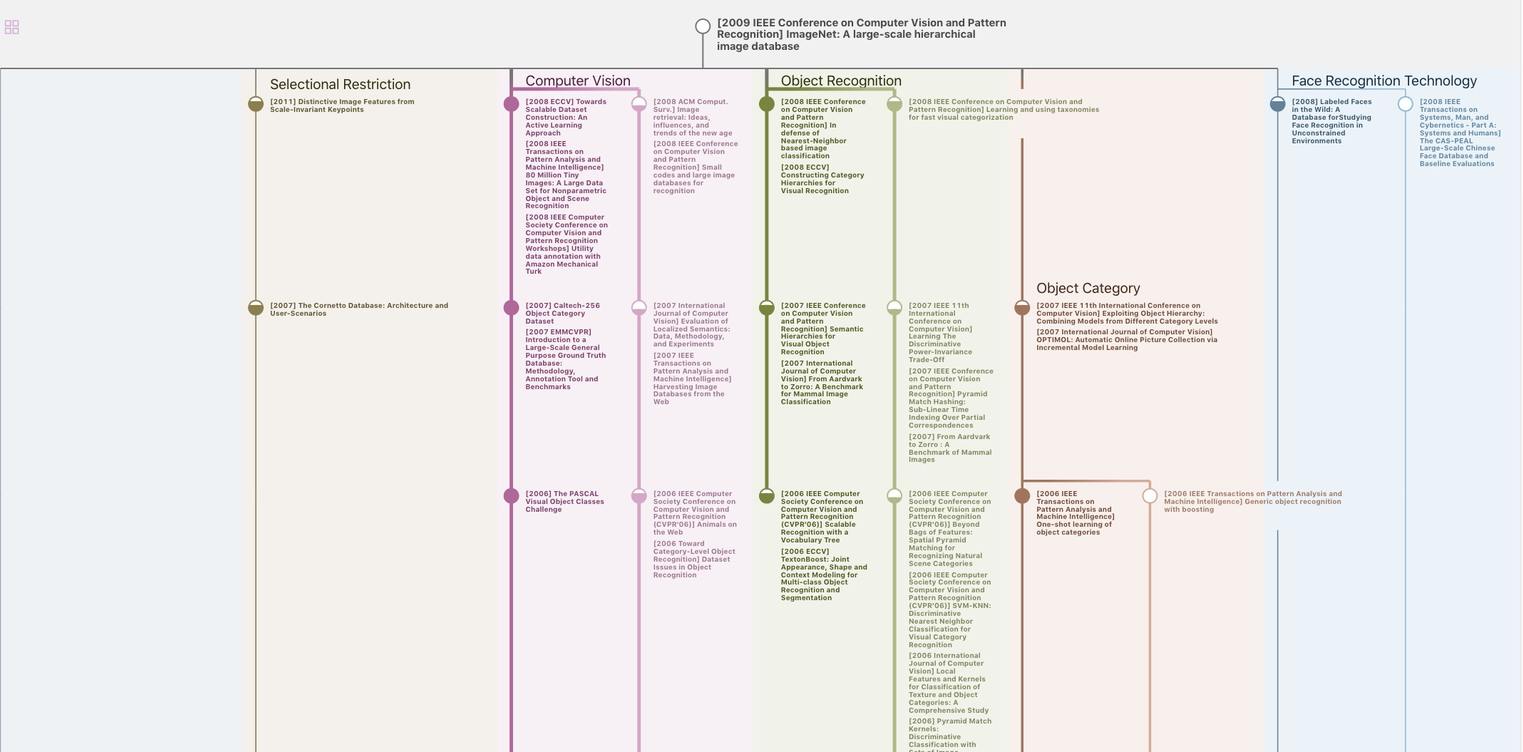
生成溯源树,研究论文发展脉络
Chat Paper
正在生成论文摘要