MEME: Multi-Encoder Multi-Expert Framework with Data Augmentation for Video Retrieval
PROCEEDINGS OF THE 46TH INTERNATIONAL ACM SIGIR CONFERENCE ON RESEARCH AND DEVELOPMENT IN INFORMATION RETRIEVAL, SIGIR 2023(2023)
Abstract
Text-to-video(T2V) retrieval aims to find relevant videos from text queries. The recently introduced Contrastive Language Image Pretraining (CLIP), a pretrained language-vision model trained on large-scale image and caption pairs, has been extensively studied in the literature for this task. Existing studies on T2V task have aimed to transfer the CLIP knowledge and focus on enhancing retrieval performance through fine-grained representation learning. While fine-grained contrast has achieved some remarkable results, less attention has been paid to coarse-grained contrasts. To this end, we propose a method called Graph Patch Spreading (GPS) to aggregate patches across frames at the coarse-grained level. We apply GPS to our proposed framework called Multi-Encoder Multi-Expert (MEME) framework. Our proposed scheme is general enough to be applied to any existing CLIP-based videotext retrieval models. We demonstrate the effectiveness of our method on existing models over the benchmark datasets MSR-VTT, MSVD, and LSMDC datasets. Our code can be found at https://github.com/kang7734/MEME_.
MoreTranslated text
Key words
Text-Video retrieval,CLIP,Multi-grained contrast
AI Read Science
Must-Reading Tree
Example
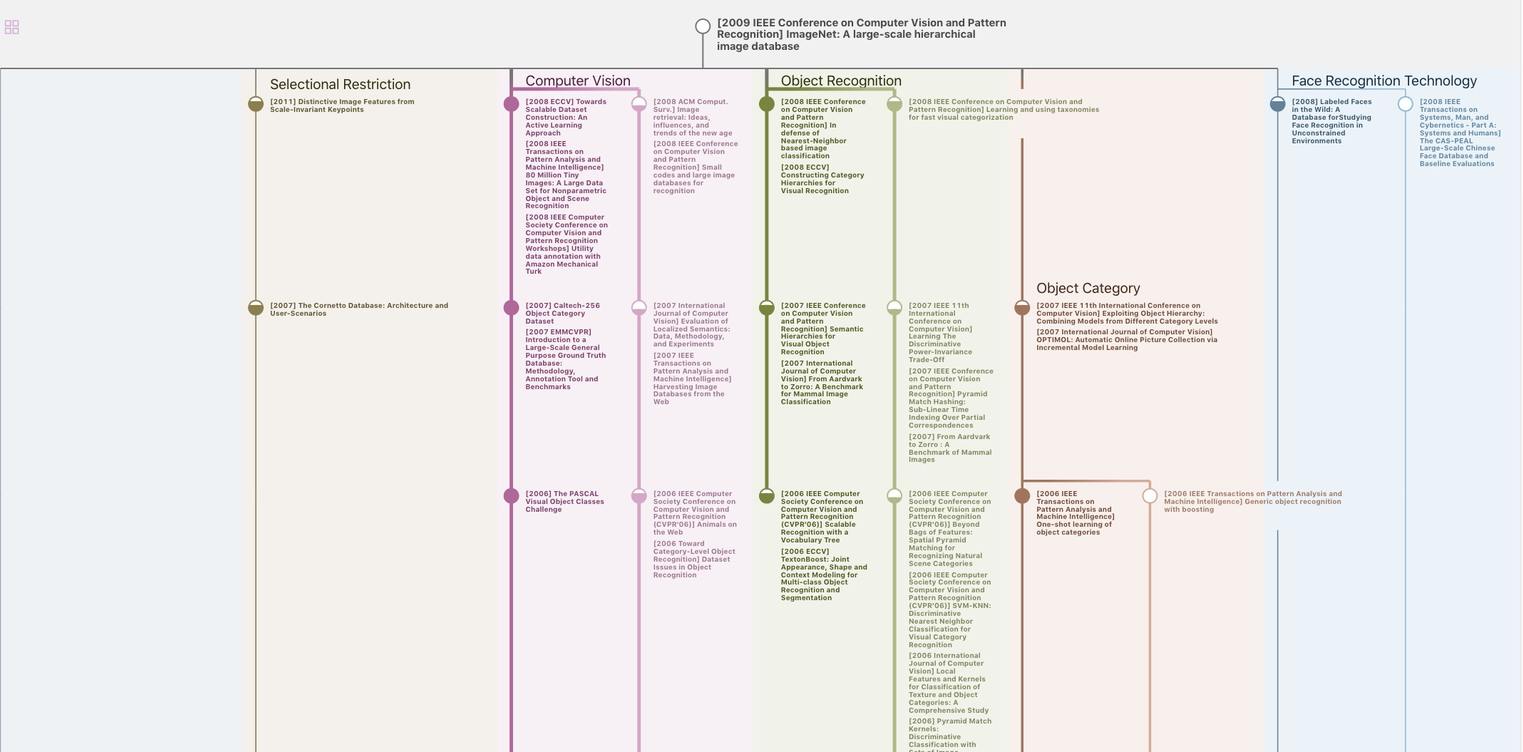
Generate MRT to find the research sequence of this paper
Chat Paper
Summary is being generated by the instructions you defined