Exploring Scenarios of Uncertainty about the Users' Preferences in Interactive Recommendation Systems
PROCEEDINGS OF THE 46TH INTERNATIONAL ACM SIGIR CONFERENCE ON RESEARCH AND DEVELOPMENT IN INFORMATION RETRIEVAL, SIGIR 2023(2023)
摘要
Interactive Recommender Systems have played a crucial role in distinct entertainment domains through a Contextual Bandit model. Despite the current advances, their personalisation level is still directly related to the information previously available about the users. However, there are at least two scenarios of uncertainty about the users' preferences over their journey: (1) when the user joins for the first time and (2) when the system continually makes wrong recommendations because of prior misleading assumptions. In this work, we introduce concepts from the Active Learning theory to mitigate the impact of such scenarios. We modify three traditional bandits to recommend items with a higher potential to get more user information without decreasing the model's accuracy when an uncertain scenario is observed. Our experiments show that the modified models outperform all baselines by increasing the cumulative reward in the long run. Moreover, a counterfactual evaluation validates that such improvements were not simply achieved due to the bias of offline datasets.
更多查看译文
关键词
Contextual Bandits,Active Learning,Counterfactual Evaluation
AI 理解论文
溯源树
样例
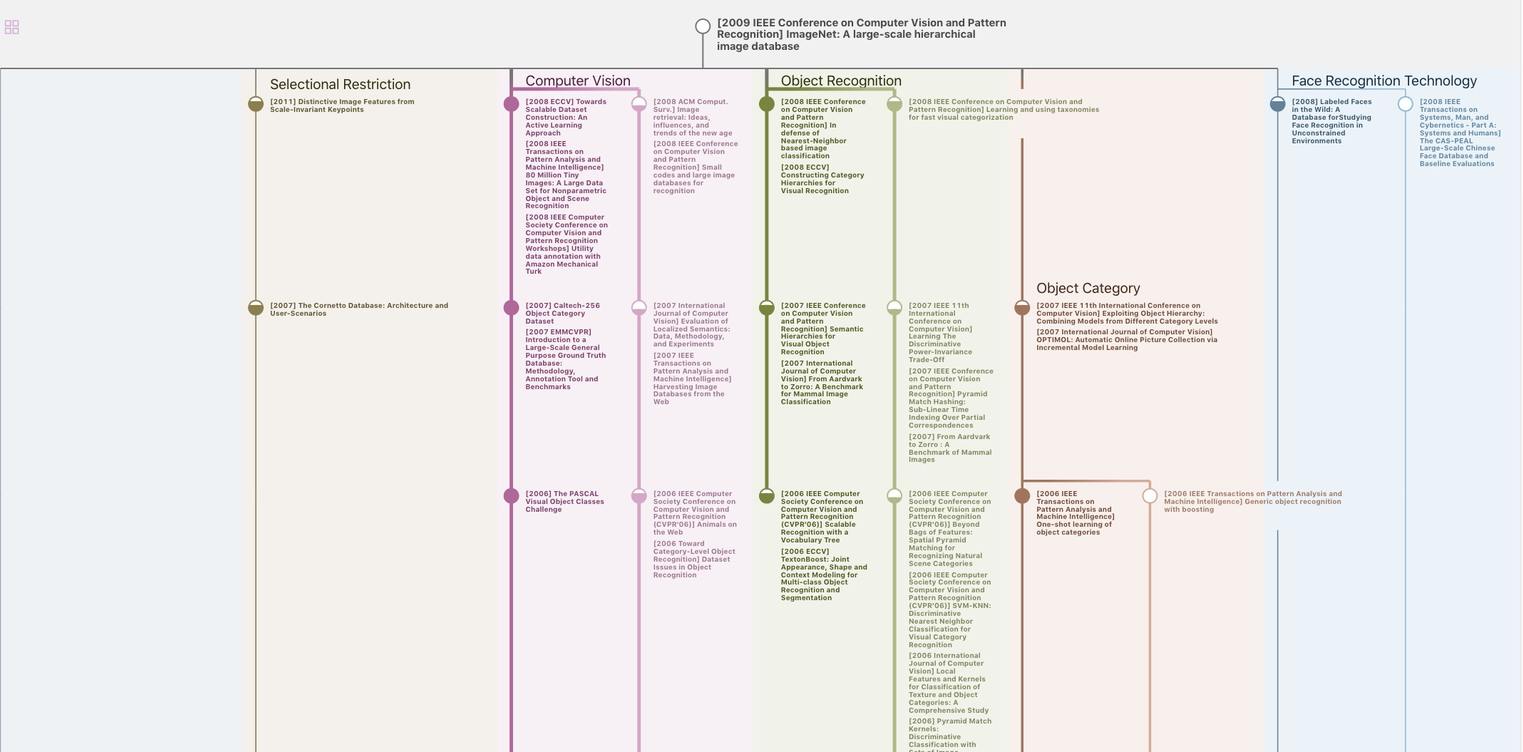
生成溯源树,研究论文发展脉络
Chat Paper
正在生成论文摘要