Beyond the Overlapping Users: Cross-Domain Recommendation via Adaptive Anchor Link Learning
PROCEEDINGS OF THE 46TH INTERNATIONAL ACM SIGIR CONFERENCE ON RESEARCH AND DEVELOPMENT IN INFORMATION RETRIEVAL, SIGIR 2023(2023)
摘要
Cross-Domain Recommendation (CDR) is capable of incorporating auxiliary information from multiple domains to advance recommendation performance. Conventional CDR methods primarily rely on overlapping users, whereby knowledge is conveyed between the source and target identities belonging to the same natural person. However, such a heuristic assumption is not universally applicable due to an individual may exhibit distinct or even conflicting preferences in different domains, leading to potential noises. In this paper, we view the anchor links between users of various domains as the learnable parameters to learn the task-relevant cross-domain correlations. A novel optimal transport based model ALCDR is further proposed to precisely infer the anchor links and deeply aggregate collaborative signals from the perspectives of intra-domain and inter-domain. Our proposal is extensively evaluated over real-world datasets, and experimental results demonstrate its superiority.
更多查看译文
关键词
Cross-domain recommendation,Optimal transportation,Graph neural networks
AI 理解论文
溯源树
样例
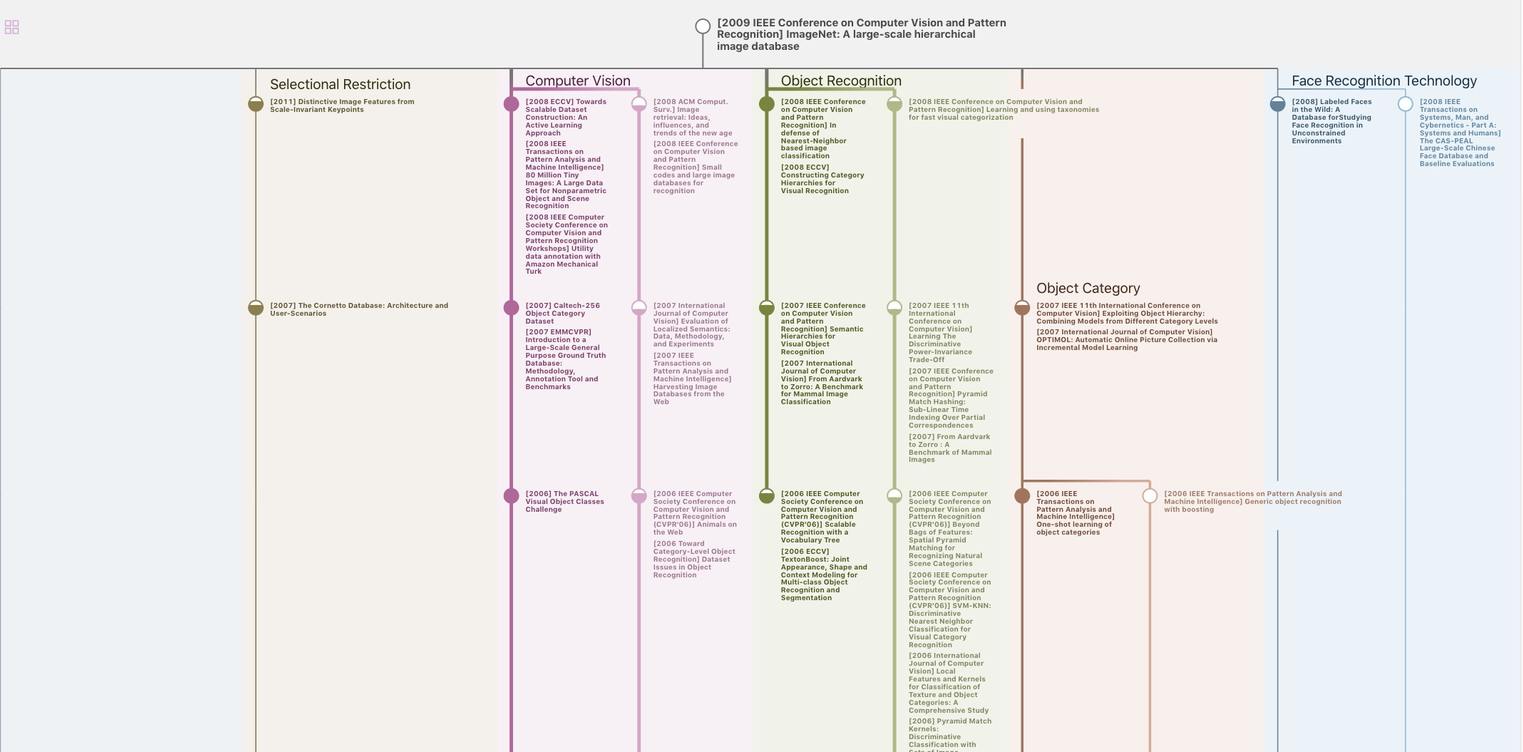
生成溯源树,研究论文发展脉络
Chat Paper
正在生成论文摘要