Knowledge-enhanced Prompt-tuning for Stance Detection.
ACM Trans. Asian Low Resour. Lang. Inf. Process.(2023)
摘要
Investigating public attitudes on social media is important in opinion mining systems. Stance detection aims to analyze the attitude of an opinionated text (e.g., favor, neutral, or against) toward a given target. Existing methods mainly address this problem from the perspective of fine-tuning. Recently, prompt-tuning has achieved success in natural language processing tasks. However, conducting prompt-tuning methods for stance detection in real-world remains a challenge for several reasons: (1) The text form of stance detection is usually short and informal, which makes it difficult to design label words for the verbalizer. (2) The tweet text may not explicitly give the attitude. Instead, users may use various hashtags or background knowledge to express stance-aware perspectives. In this article, we first propose a prompt-tuning-based framework that performs stance detection in a cloze question manner. Specifically, a knowledge-enhanced prompt-tuning framework (KEprompt) method is designed, which consists of an automatic verbalizer (AutoV) and background knowledge injection (BKI). Specifically, in AutoV, we introduce a semantic graph to build a better mapping from the predicted word of the pretrained language model and detection labels. In BKI, we first propose a topic model for learning hashtag representation and introduce ConceptGraph as the supplement of the target. At last, we present a challenging dataset for stance detection, where all stance categories are expressed in an implicit manner. Extensive experiments on a large real-world dataset demonstrate the superiority of KEprompt over state-of-the-art methods.
更多查看译文
关键词
Stance detection, deep learning, prompt-tuning framework
AI 理解论文
溯源树
样例
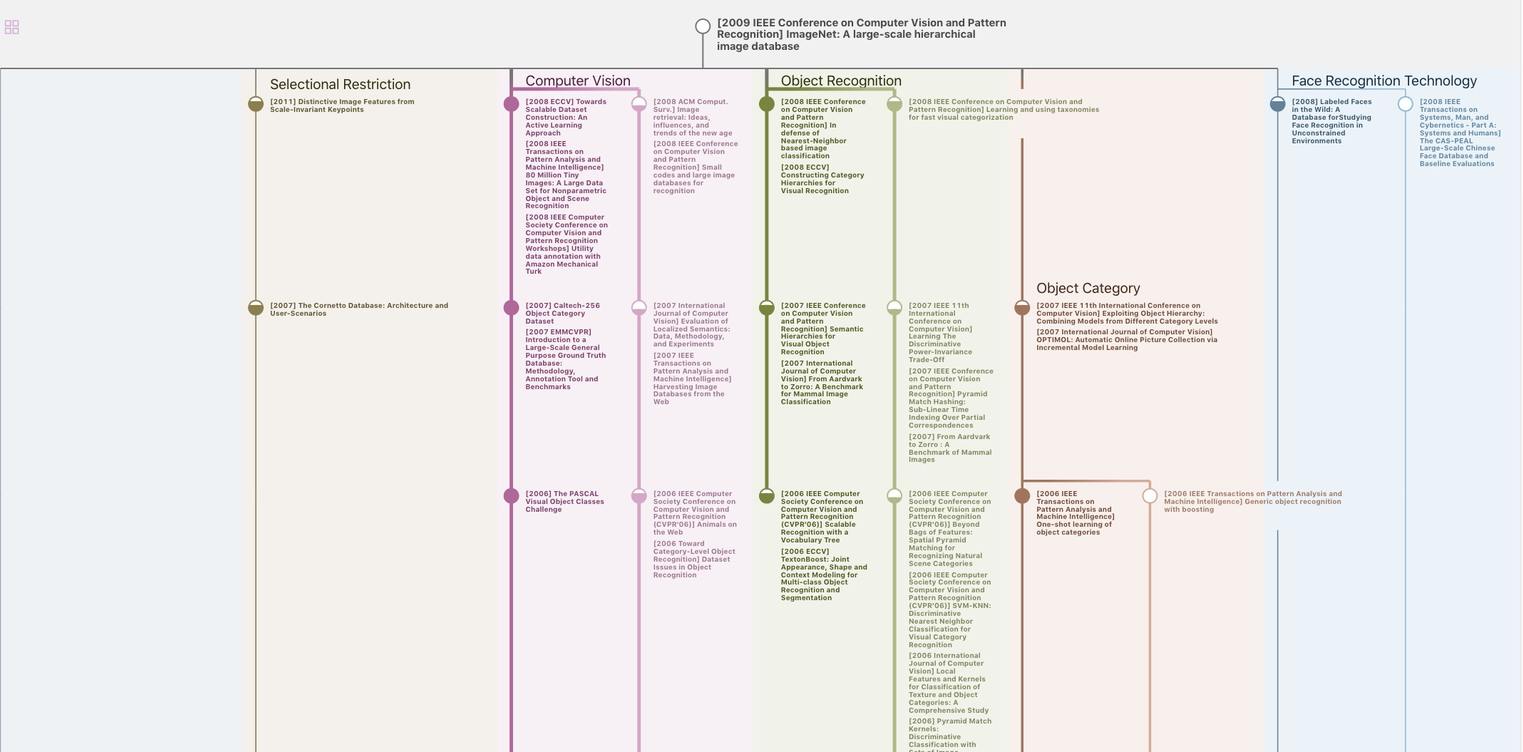
生成溯源树,研究论文发展脉络
Chat Paper
正在生成论文摘要