Sharp Performance Bounds for PASTA.
IEEE Control. Syst. Lett.(2023)
摘要
LiDAR is a standard sensor choice for self-localization and SLAM on indoor autonomous robots. While there are many methods to estimate a robot's location using LiDAR measurements, most rely on algorithms that solve a generic LiDAR scan matching problem. When safety is a concern, these algorithms must provide a bound on the localization error to enable safety enforcing controllers, such as those based on Control Barrier Functions. Unfortunately, most existing scan matching algorithms offer no formal guarantees and are tailored to structured, high-resolution 3D point clouds. In this letter, we present an improved theoretical analysis for a low-cost alternative to these methods named PASTA (Provably Accurate Simple Transformation Alignment), originally introduced in (Marchi et al., 2022). We provide a formal worst-case guarantee on the localization error and show, experimentally, that it is tight. This characterization of the localization error simplifies the interface between high-dimensional perception data and safety-critical control.
更多查看译文
关键词
Estimation, autonomous systems, control applications
AI 理解论文
溯源树
样例
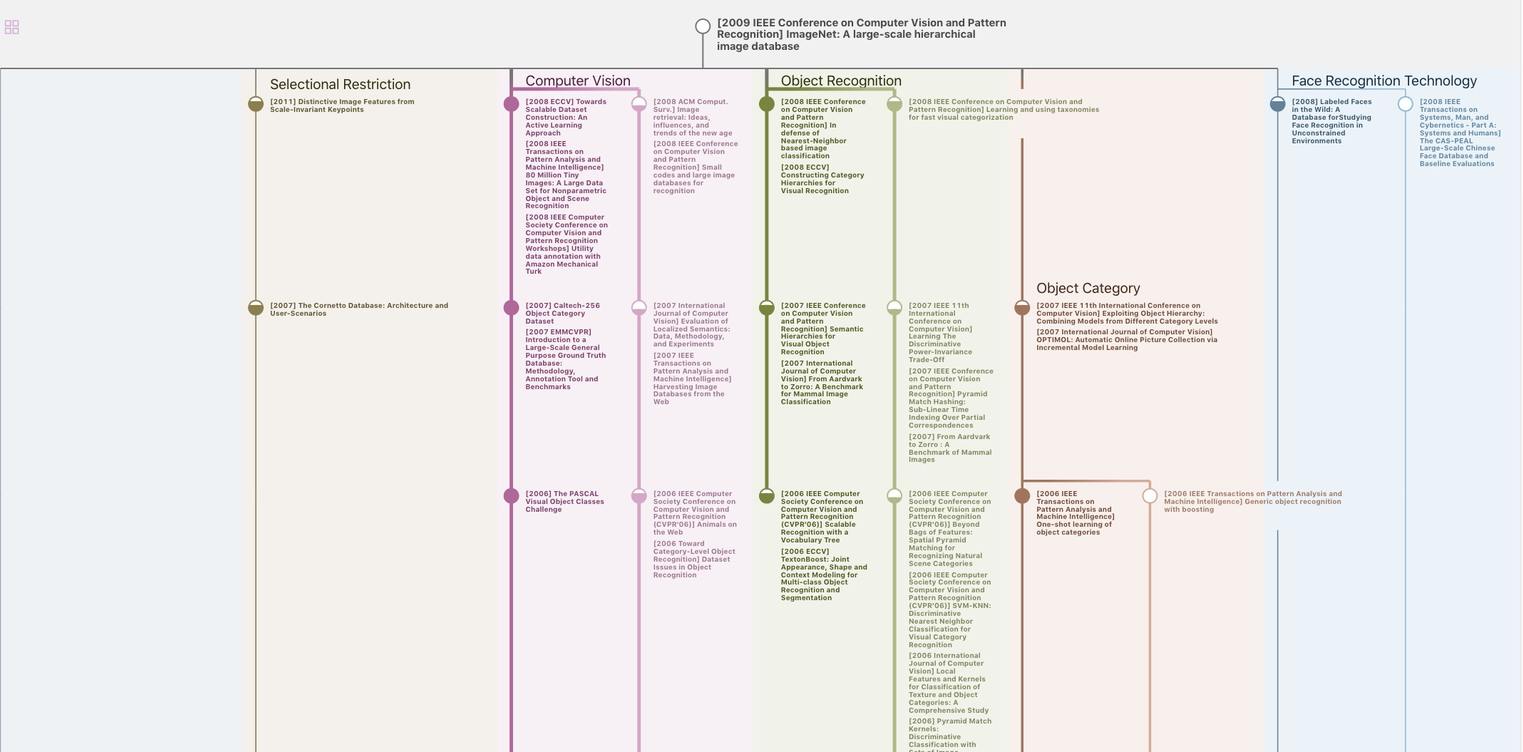
生成溯源树,研究论文发展脉络
Chat Paper
正在生成论文摘要