A Coupled Temporal-Spectral-Spatial Multidimensional Information Change Detection Framework Method: A Case of the 1990-2020 Tianjin, China.
IEEE J. Sel. Top. Appl. Earth Obs. Remote. Sens.(2023)
Abstract
Satellite image time-series change detection methods have become an effective means of obtaining information on land cover change. However, the temporal, spectral, and spatial features and their derived features of land cover objects are of great importance for time-series change detection. The existing studies have made insufficient use of these features, which may affect the results of land cover change detection. In order to fully integrate the above features to portray and represent change information, this study proposes a coupled temporal–spectral–spatial multidimensional information change detection framework (TSSF) method. First, the derived index features are calculated to construct intra-annual temporal–spectral information to reduce the underutilization of spectral features. Second, the intra-annual temporal–spectral information is extended to the spatiotemporal domain by the simple noniterative clustering method and the SG filtering method to increase the exploitation of spatial features. Then, the value and shape-based dynamic time warping method and the change vector analysis in the posterior probability space method are employed to obtain change information from the spectral, index, and class probability perspectives. Finally, the change type in the change region is obtained from the class probability information of the change magnitude according to the Bayesian criterion. Tianjin City was used as the study area to explore the land cover change from 1990 to 2020. The results show that the TSSF method is feasible in expressing temporal–spectral–spatial change information compared with the existing methods and is conducive to the efficient acquisition and identification of change areas and change types.
MoreTranslated text
Key words
Change detection,change vector analysis in posterior probability space (CVAPS),dynamic time warping,Tianjin,time series
AI Read Science
Must-Reading Tree
Example
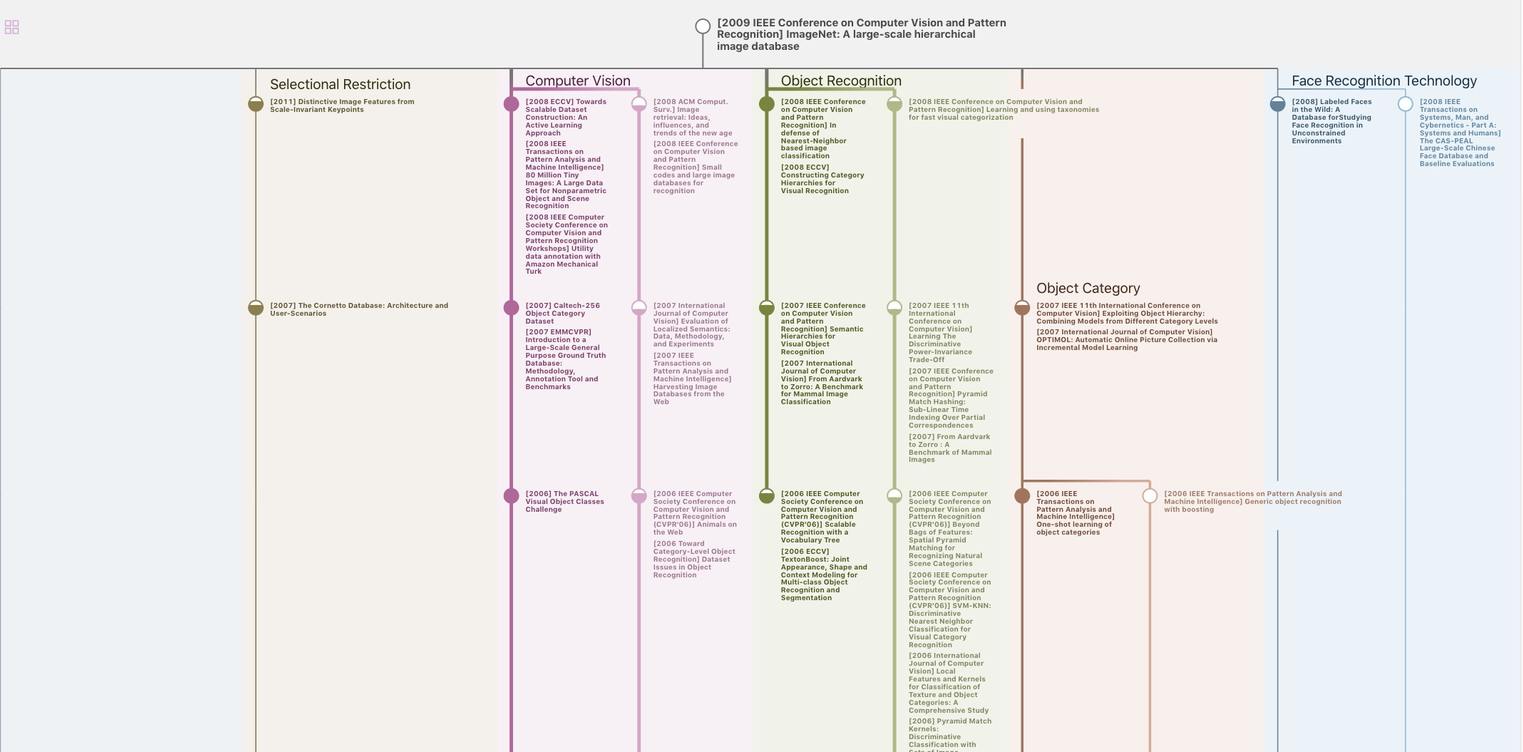
Generate MRT to find the research sequence of this paper
Chat Paper
Summary is being generated by the instructions you defined