Context Conditioning via Surrounding Predictions for Non-Recurrent CTC Models.
IEEE Access(2023)
摘要
Connectionist Temporal Classification (CTC) loss has become widely used in sequence modeling tasks such as Automatic Speech Recognition (ASR) and Handwritten Text Recognition (HTR) due to its ease of use. Recent sequence models that incorporate CTC loss have been focusing on speed by removing recurrent structures, hence losing important context information. This paper presents extensive studies of Contextualized Connectionist Temporal Classification (CCTC) framework, which induces prediction dependencies in non-recurrent and non-autoregressive neural networks for sequence modeling. CCTC allows the model to implicitly learn the language model by predicting neighboring labels via multi-task learning. Experiments on ASR and HTR tasks in two different languages show that CCTC models offer improvements over CTC models by 2.2-8.4% relative without incurring extra inference costs. We have also found that higher order of context information can potentially help the model produce better predictions.
更多查看译文
关键词
CTC, contextualized CTC, non-recurrent, non-autoregressive, automatic speech recognition (ASR), handwritten text recognition (HTR)
AI 理解论文
溯源树
样例
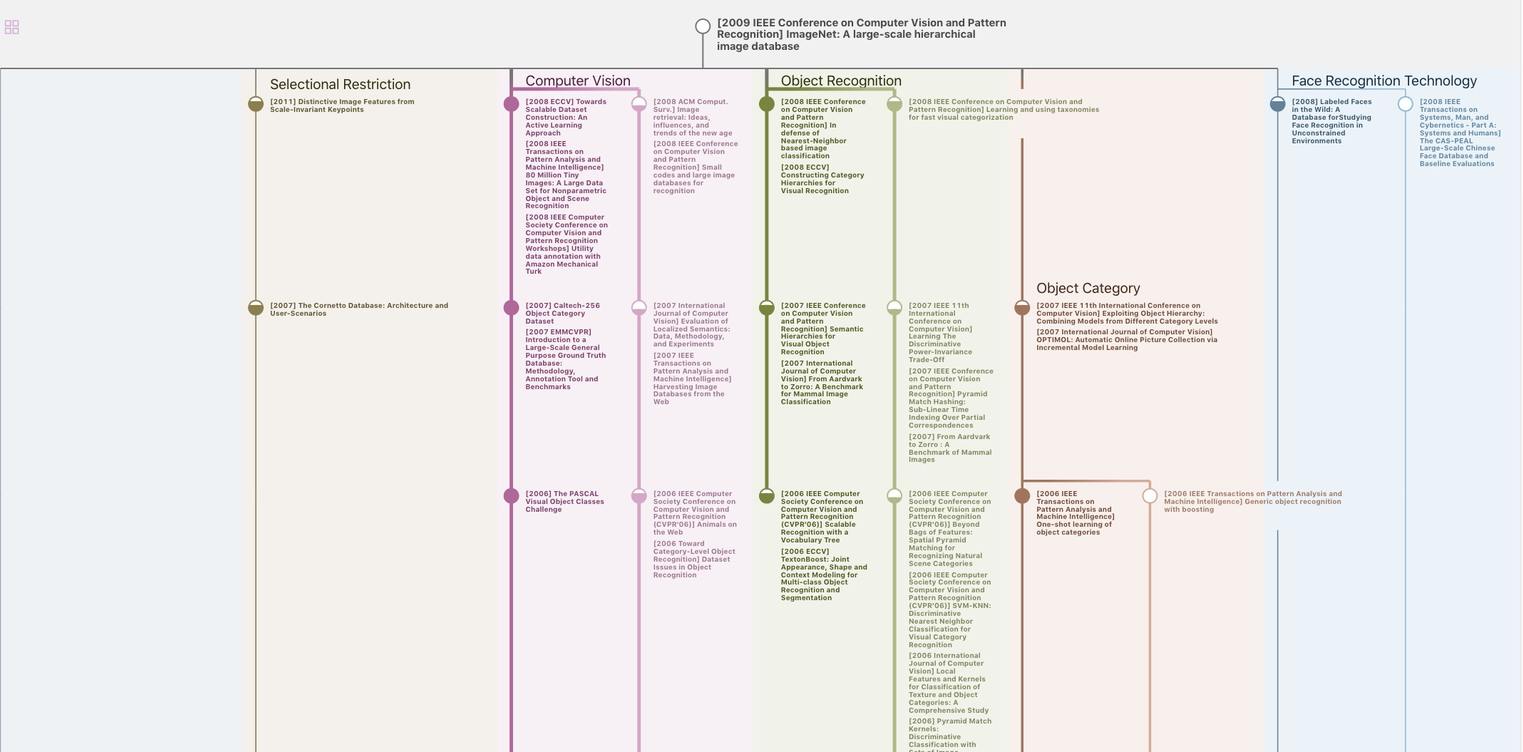
生成溯源树,研究论文发展脉络
Chat Paper
正在生成论文摘要