Autoregressive Decoder With Extracted Gap Sessions for Sequential/Session-Based Recommendation.
IEEE Access(2023)
摘要
Learning the complex relationships between items in a sequential recommendation system (SRS) and session-based recommendation system (SBRS) is critical for obtaining higher prediction scores. In recent studies, to capture item-item information, items have been represented as the nodes of graph neural networks (GNNs) and the relevance of items with self-/soft attention layers has been calculated. GNNs have been used because standalone attention-based methods focus only on the relative significance of items within a single session, neglecting high-order item-item relationships that change through sessions. The relational summarization task is a natural language processing task that extracts the relationship between two tokens from a related corpus; however, its adaptation to SRS and SBRS is unknown. To fill this lacuna, in this study, the relationships between items from related sessions are extracted using the transformer-based abstractive summarization model PEGASUS. To improve session embedding, the proposed model, named "gap-session transformer" utilizes gap-session masking to learn the relationships between items within different sessions. In addition, a group of sessions are divided into multiple corpus sets based on the theme of each corpus, and the autoregressive beam-search decoder is connected to a transformer decoder for the generation of the next session while auxiliary tasks are performed to enhance the recommendation task. Extensive experiments conducted on the MovieLens1M dataset and Yoochoose dataset verify that our model significantly outperforms the state-of-the-art (SOTA) methods, and the results demonstrate the efficacy of the relational summarization task in recommendation systems.
更多查看译文
关键词
Recommender systems,Pegasus,transformer
AI 理解论文
溯源树
样例
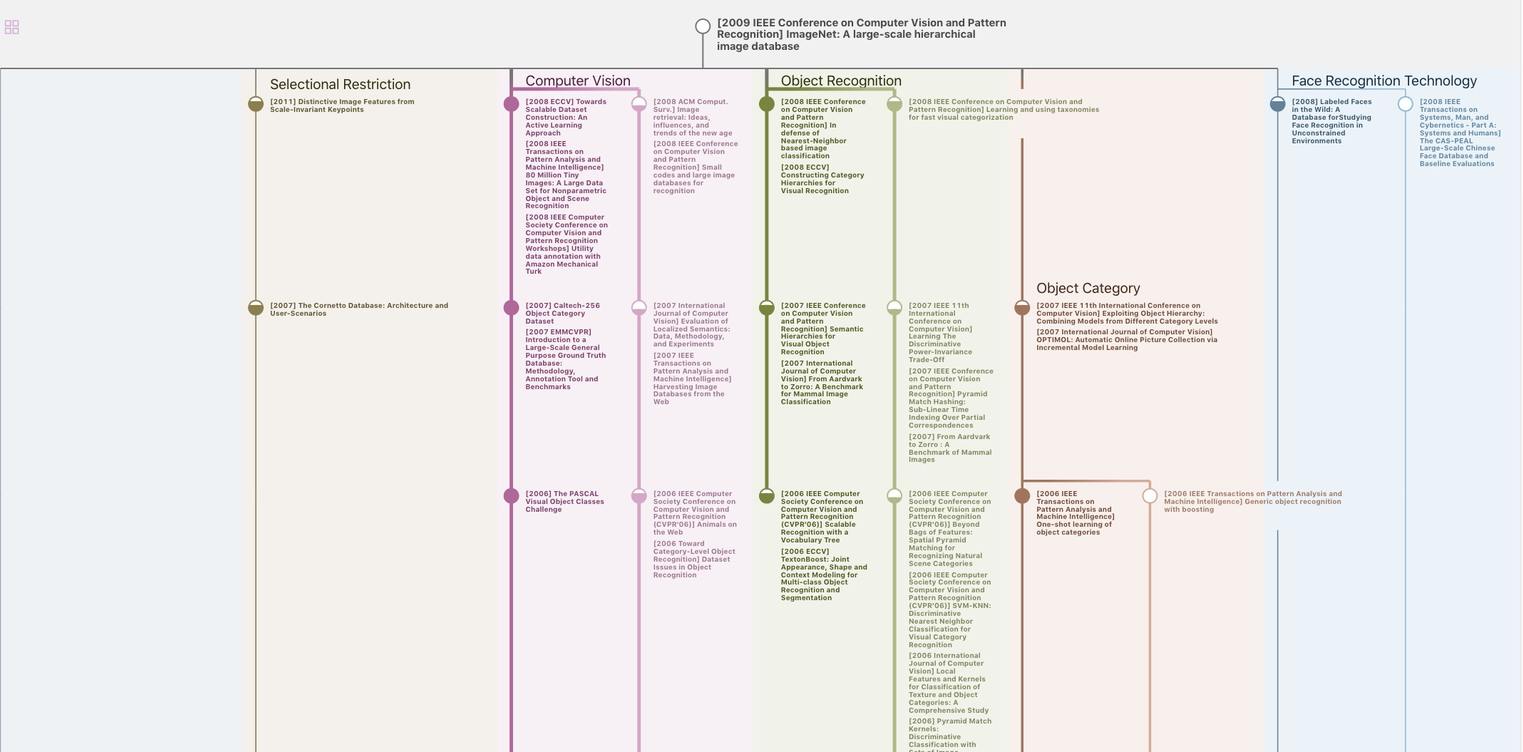
生成溯源树,研究论文发展脉络
Chat Paper
正在生成论文摘要