Semantic Segmentation With Multiple Contradictory Annotations Using a Dynamic Score Function.
IEEE Access(2023)
摘要
Semantic Segmentation aims to partition an image into separate regions where each region conveys certain valuable information. In recent years, deep learning models have achieved high performance in this task. However, when several ground truth segmentations are available, aggregating the information of these segmentations into a single ground truth becomes a crucial pre-processing step. This task becomes challenging when the segmentations are contradictory and the existing classes in the segmentations are imbalanced. An elegant example is the grading of Prostate Cancer in the Gleason 2019 Challenge dataset. This dataset provides six annotations of relatively high contrast from expert pathologists for each image. Additionally, the low number of images for Gleason grade 5 has also resulted in an imbalanced dataset. The Majority Voting and the Simultaneous Truth And Performance Level Estimation (STAPLE) algorithm are the most popular algorithms for combining annotations. In this paper, we visually show that the outputs of these algorithms discard the semantics of the image in regions of high inconsistency and point out that they demote low-frequency patterns, making the final segmentations even more imbalanced. We claim these drawbacks highly decrease the performance of deep learning models trained on these ground truths. To solve this problem, we propose a dynamic score function that selects one of the six annotations for each image while balancing the Gleason grading among the annotations in terms of variability and quantity. Finally, we train and evaluate a Pyramid Scene Parsing network on the final ground truths to validate our claims.
更多查看译文
关键词
INDEX TERMS Convolutional neural networks, Gleason 2019 challenge, Gleason grading, Multi-expert annotations, Prostate cancer, Semantic segmentation
AI 理解论文
溯源树
样例
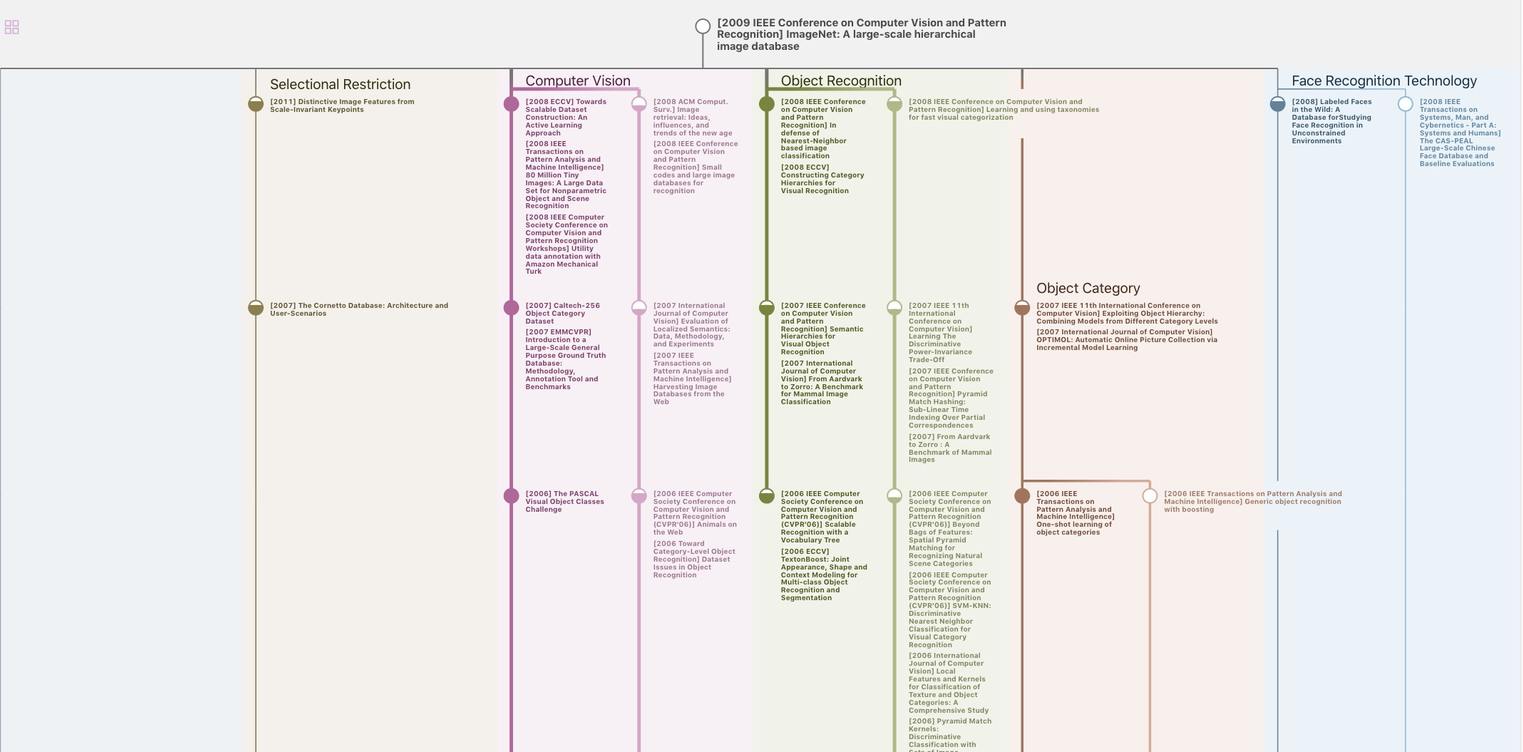
生成溯源树,研究论文发展脉络
Chat Paper
正在生成论文摘要