Avoiding Shortcut-Learning by Mutual Information Minimization in Deep Learning-Based Image Processing.
IEEE Access(2023)
摘要
Deep learning models are increasingly being used in detecting patterns and correlations in medical imaging data such as magnetic resonance imaging. However, conventional methods are incapable of considering the real underlying causal relationships. In the presence of confounders, spurious correlations between data, imaging process, content, and output can occur that allow the network to learn shortcuts instead of the desired causal relationship. This effect is even more prominent in new environments or when using out-of-distribution data since the learning process is primarily focused on correlations and patterns within the data. Hence, wrong conclusions or false diagnoses can be obtained from such confounded models. In this paper, we propose a novel framework, denoted as Mutual Information Minimization Model (MIMM), that predicts the desired causal outcome while simultaneously reducing the influence of present spurious correlations. The input imaging data is encoded into a feature vector that is split into two components to predict the primary task and the presumed spuriously correlated factor separately. We hypothesize that learned mutual information between both feature vector components can be reduced to achieve independence, i.e., confounder-free task prediction. The proposed approach is investigated on five databases: two non-medical benchmark databases (Morpho-MNIST and Fashion-MNIST) to verify the hypothesis and three medical databases (German National Cohort, UK Biobank, and ADNI). The results show that our proposed framework serves as a solution to address the limitations of conventional deep learning models in medical image analysis. By explicitly considering and minimizing spurious correlations, it learns causal relationships which result in more accurate and reliable predictions. The novel contributions in this work are: 1) the separation of features into the prediction of the primary task and the spuriously correlated factor; 2) MIMM targets the preservation of invariance to counterfactuals, prevents shortcut learning, and enables confounder-free network training; and 3) the mutual information minimization addresses heterogeneous data cohorts as usually encountered in the medical domain.
更多查看译文
关键词
Causality, deep learning, medical image analysis, mutual information, shortcut learning
AI 理解论文
溯源树
样例
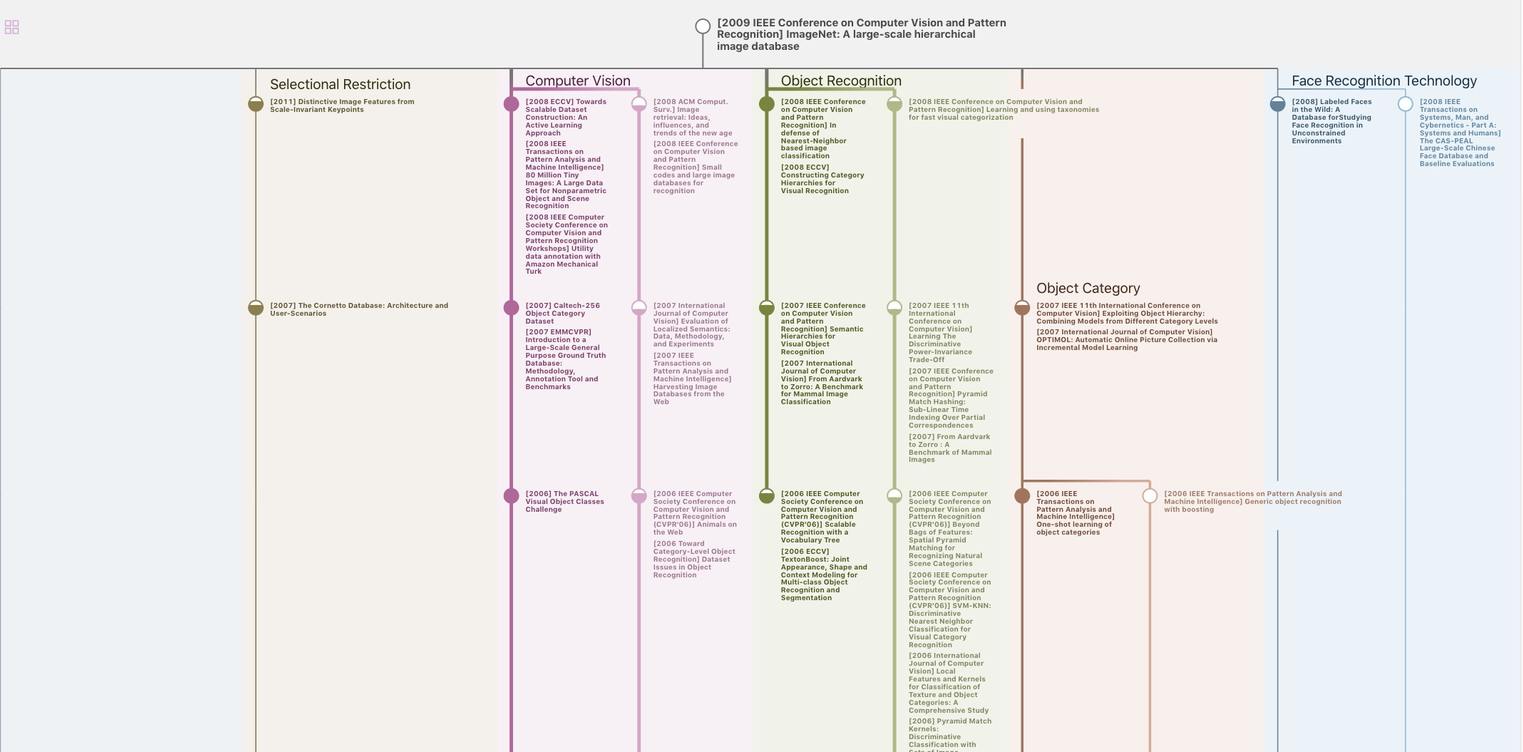
生成溯源树,研究论文发展脉络
Chat Paper
正在生成论文摘要