Tucker Decomposition Based on a Tensor Train of Coupled and Constrained CP Cores.
IEEE Signal Process. Lett.(2023)
摘要
Many real-life signal-based applications use the Tucker decomposition of a high dimensional/order tensor. A well-known problem with the Tucker model is that its number of entries increases exponentially with its order, a phenomenon known as the "curse of the dimensionality". The Higher-Order Orthogonal Iteration (HOOI) and Higher-Order Singular Value Decomposition (HOSVD) are known as the gold standard for computing the range span of the factor matrices of a Tucker Decomposition but also suffer from the curse. In this letter, we propose a new methodology with a similar estimation accuracy as the HOSVD with non-exploding computational and storage costs. If the noise-free data follows a Tucker decomposition, the corresponding Tensor Train (TT) decomposition takes a remarkable specific structure. More precisely, we prove that for a Q-order Tucker tensor, the corresponding TT decomposition is constituted by Q - 3 3-order TT-core tensors that follow a Constrained Canonical Polyadic Decomposition. Using this new formulation and the coupling property between neighboring TT-cores, we propose a JIRAFE-type scheme for the Tucker decomposition, called TRIDENT. Our numerical simulations show that the proposed method offers a drastically reduced complexity compared to the HOSVD and HOOI while outperforming the Fast Multilinear Projection (FMP) method in terms of estimation accuracy.
更多查看译文
关键词
Tensor, tucker decomposition, constrained CPD, tensor train, multilinear algebra
AI 理解论文
溯源树
样例
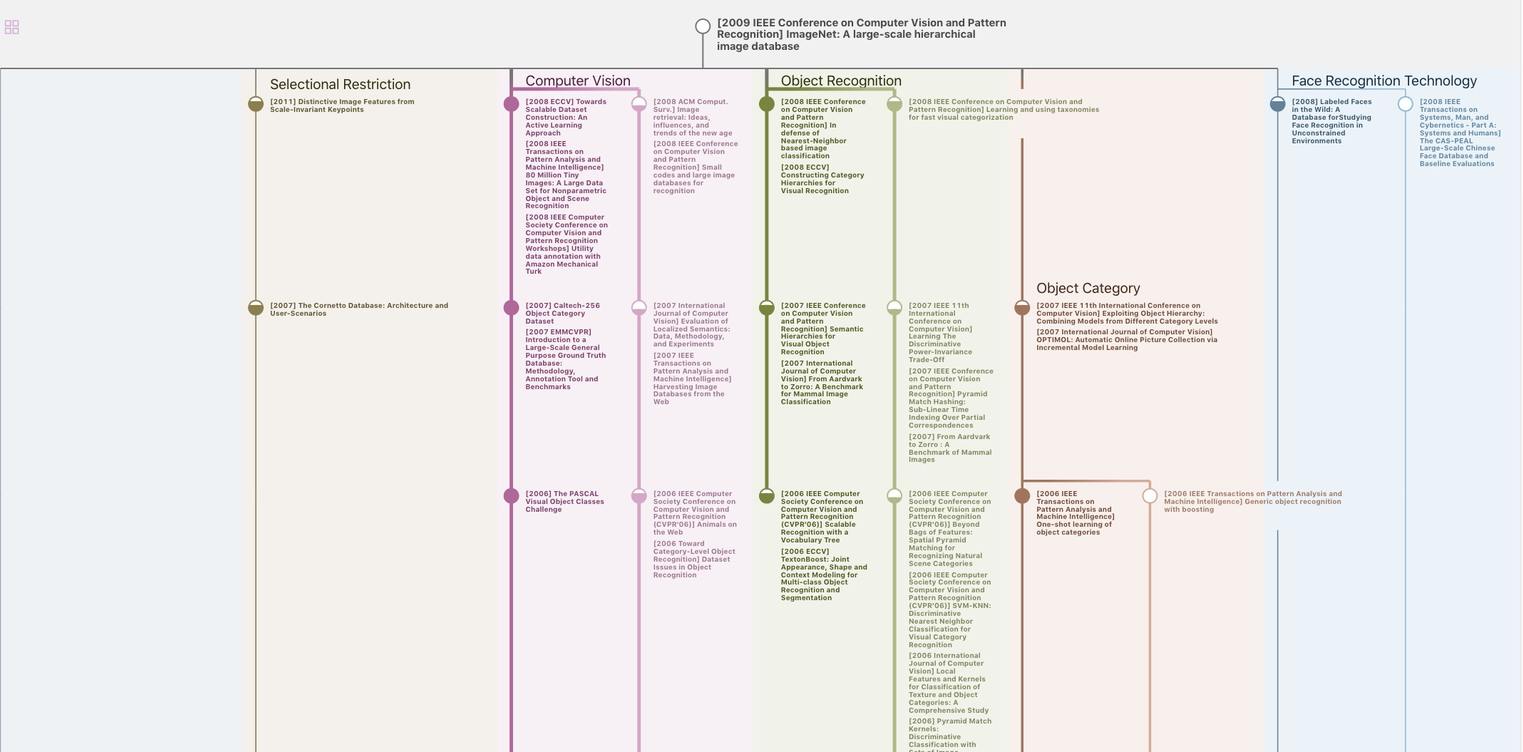
生成溯源树,研究论文发展脉络
Chat Paper
正在生成论文摘要