Deepfake Fingerprint Detection Model Intellectual Property Protection via Ridge Texture Enhancement.
IEEE Signal Process. Lett.(2023)
摘要
In addition to relying on super computing power and professional domain knowledge, training a high-precision deepfake fingerprint detection model (DFDM) to authenticate the fingerprints also requires the support of massive private fingerprint data. To sum up, the DFDM should be deemed as intellectual property (IP) of the trainers, so it is crucial to protect IP. Currently, most watermarking-based IP protection schemes are implemented by introducing additional tasks, such as constructing trigger sets, fine-tuning model weights, etc., which severely impair the performance of the original task and increase the training cost. Inspired by the feature knowledge learned by the model, this letter proposes an IP protection (IPP) scheme for DFDM by verifying whether the suspect model contains the fingerprint ridge features learned by the victim model from another perspective based on the verifier. Firstly, the local binary pattern (LBP) is used to enhance the ridge texture on the fingerprint samples, so that DFDM can better learn the ridge features. Then, a DFDM lacking texture augmentation is employed as the adversarial model for training the meta-verifier without any alteration to the model parameters. Finally, the trained meta-verifier is used to determine whether the suspected model contains the ridge features in the victim model. The public fingerprint dataset (LivDet2017) was leveraged in the DFDM training process to validate our approach. Experimental results show that the proposed scheme can verify the IP of DFDM and is robust to some common attacks.
更多查看译文
关键词
Deepfake Fingerprint Detection,Intellectual Property Protection,Feature Knowledge,Model Watermarking,Model Stealing
AI 理解论文
溯源树
样例
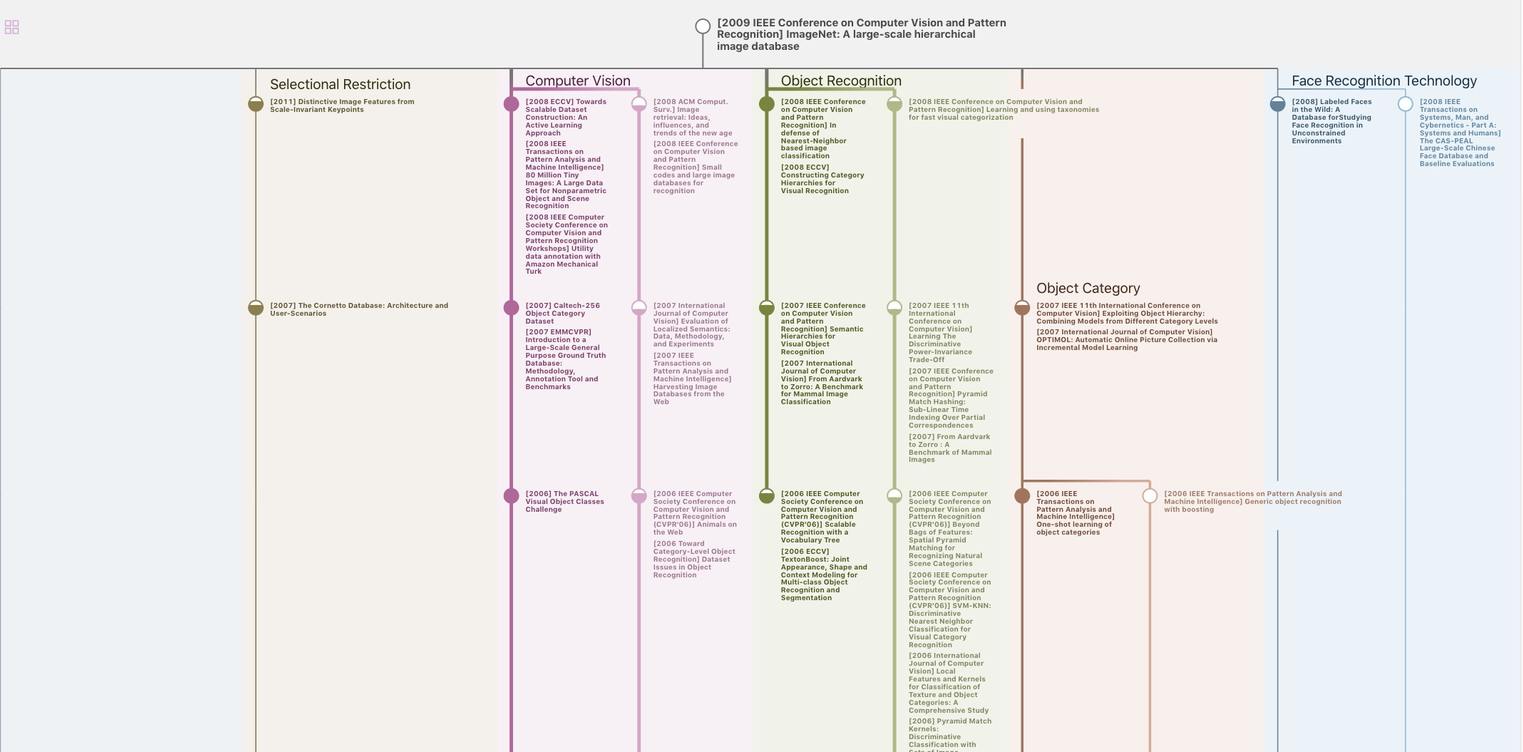
生成溯源树,研究论文发展脉络
Chat Paper
正在生成论文摘要