Automatic Pylon Extraction Using Color-Aided Classification From UAV LiDAR Point Cloud Data.
IEEE Trans. Instrum. Meas.(2023)
摘要
Extracting pylons from point clouds gathered by unmanned aerial vehicle LiDAR systems (UAVLS) is challenging, particularly in complex environments. The difficulty arises due to the presence of nearby objects and vegetation, which are intertwined around the pylon legs and structures. In such situations, it is hard to identify pylon points from vegetation points using spatial point-level features. To overcome this issue, we propose a classification approach using the random forest (RF) classifier incorporating smoothed color features. The procedure involves the following steps: first, a set of local geometry and distribution features are generated by combining spherical and cylindrical neighborhoods. The optimal neighborhood radius is then determined by experimenting with radii ranging from 1 to 8 m. To further enhance the method's accuracy, we introduce smoothed color features within a neighborhood to eliminate the misclassification points of the vegetation close to the pylon leg. The results indicate that smoothed color features outperform intensity and single-color features, achieving a high extraction rate of 98.65% and 99.99% for the two datasets, respectively. Furthermore, our method is robust against varying levels of noise and density, and it significantly improves pylon classification accuracy when coupled with other classifiers such as support vector machine (SVM) and AdaBoost.
更多查看译文
关键词
Color features, feature set, point cloud, pylon extraction, random forest (RF)
AI 理解论文
溯源树
样例
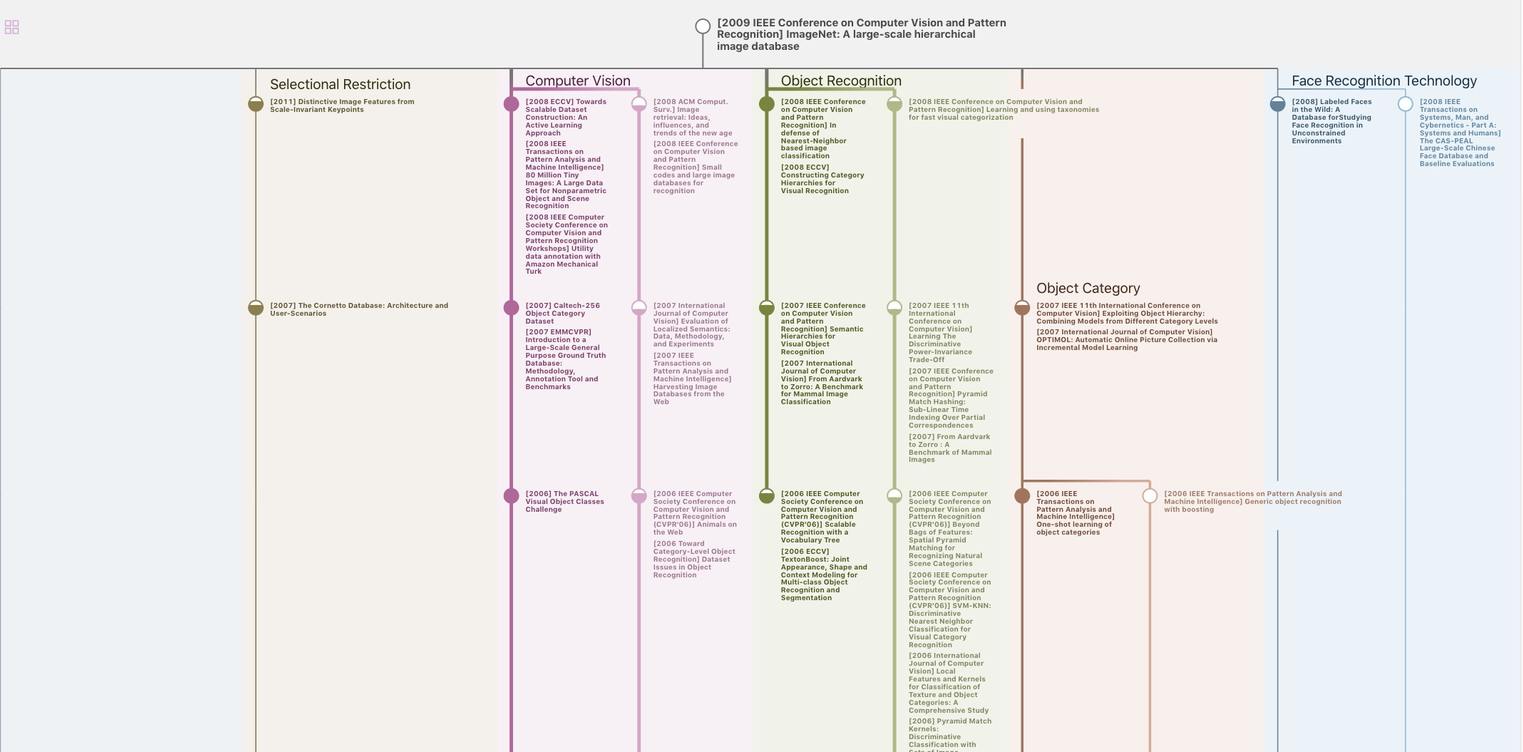
生成溯源树,研究论文发展脉络
Chat Paper
正在生成论文摘要