A LightGBM-Based Multiscale Weighted Ensemble Model for Few-Shot Fault Diagnosis.
IEEE Trans. Instrum. Meas.(2023)
Abstract
Effective fault diagnosis on rotating machinery is crucial for ensuring the reliability and safety of mechanical equipment. However, available fault data are frequently scarce in real industrial settings, leading most deep learning (DL)-based diagnostic approaches to employ a source domain with rich cross-domain data to assist model training, but obviously, creating such a domain is expensive and time-consuming. To address this issue, a novel light gradient boosting machine-based multiscale weighted ensemble model (MWE-LightGBM) is proposed, aiming to perform effective few-shot fault diagnosis without requiring cross-domain data. First, multiscale sliding windows are constructed to subsample target samples for data augmentation and overfitting avoidance. Second, the augmented data are fed into multiple LightGBM classifiers, which are then further integrated as an ensemble model. Through the ensemble, the potential diagnostic error of each classifier can be reduced, thus increasing the overall model's generalization ability. Finally, a weighting strategy based on similarity metric is developed to adjust the output of the ensemble model for further improved diagnostic accuracy. Experimental results demonstrate that the suggested approach exhibits superior classification performance in few-shot scenarios even without source domain assistance and requires less computation time, significantly outperforming other comparison approaches.
MoreTranslated text
Key words
Ensemble learning,fault diagnosis,few-shot learning,LightGBM,similarity metric
AI Read Science
Must-Reading Tree
Example
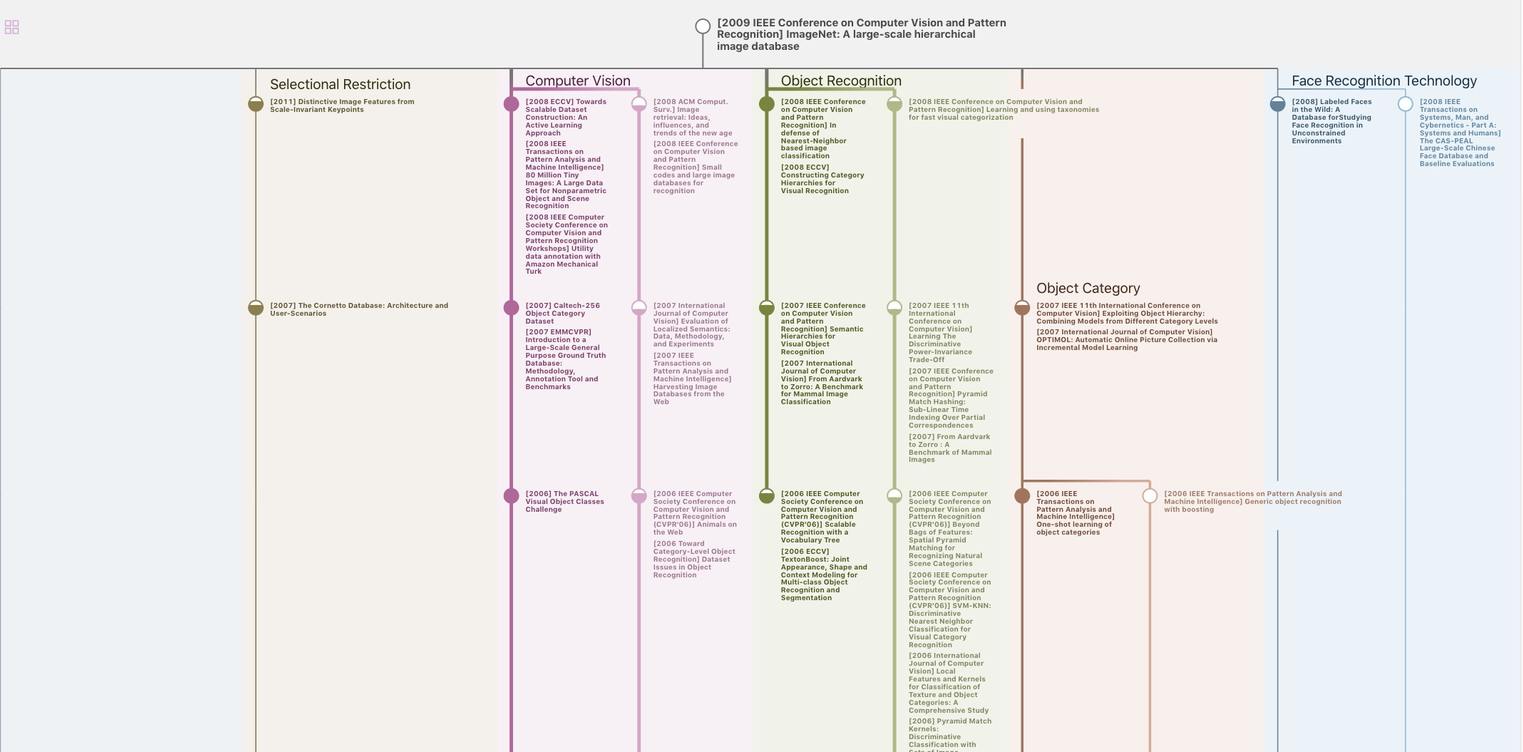
Generate MRT to find the research sequence of this paper
Chat Paper
Summary is being generated by the instructions you defined