A Novel Bearing Fault Diagnosis Algorithm Based on Adaptive Weighted Multiview Learning.
IEEE Trans. Instrum. Meas.(2023)
摘要
In fault diagnosis, feature extraction has always been regarded as the most critical step, which is directly related to the results and accuracy of diagnosis. To address the problems of insufficient feature extraction capability of single view for complex vibration signals and the high economic cost of multisource information fusion, this article proposes a fast-bearing fault diagnosis algorithm based on adaptive weighted multiview learning. First, in order to obtain different views of the vibration signal, the time-domain, frequency-domain, wavelet-domain, and entropy-domain features of the signal are extracted as individual views for the input of multiview learning. Then, the weights of different views are initialized by using the reciprocal of the view number and mapped to a kernel space with radial basis functions. To enhance the complementarity between different views, the corresponding weights are updated by iterative self-weighting operation according to the contributions of views. Finally, the classification task is converted to a regression problem to achieve fault diagnosis by selecting the maximum predicted value as the category's label. In order to verify the performance of the proposed algorithm, we conduct a series of experiments on four datasets and compared the results with some classical fault diagnosis algorithms. Experimental results demonstrate that the proposed algorithm has higher accuracy and better performance than the comparison algorithms. Moreover, it also exhibits excellent generalization and robustness on complex vibration signals.
更多查看译文
关键词
Bearing fault diagnosis,entropy,multiview learning,tunable Q-factor wavelet transform (TQWT),weight self-updating
AI 理解论文
溯源树
样例
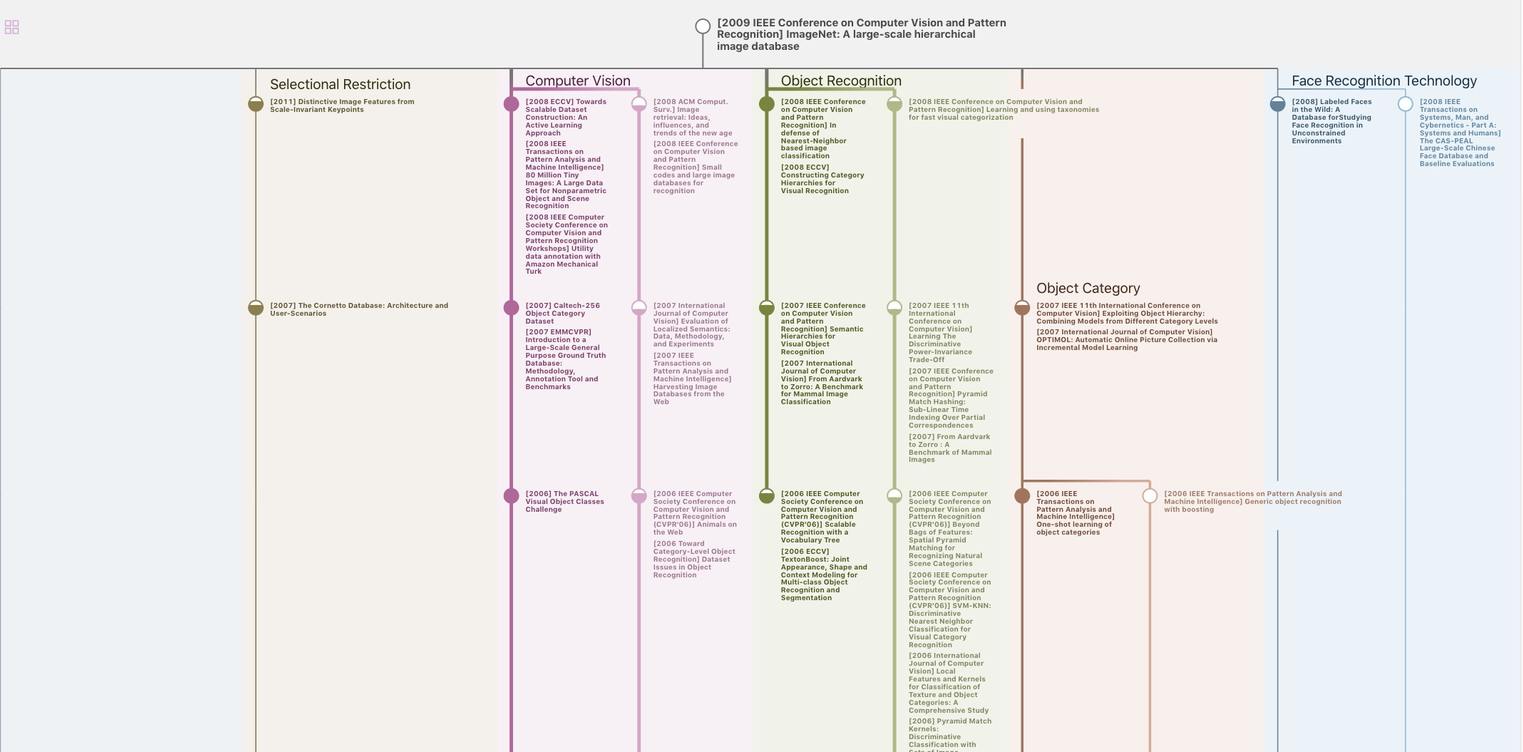
生成溯源树,研究论文发展脉络
Chat Paper
正在生成论文摘要