An Imbalanced Data Augmentation and Assessment Method for Industrial Process Fault Classification with Application in Air Compressors.
IEEE TRANSACTIONS ON INSTRUMENTATION AND MEASUREMENT(2023)
Key words
Air compressor,dynamic time warping (DTW),fault diagnosis,generative adversarial network (GAN),maximum information coefficient (MIC)
AI Read Science
Must-Reading Tree
Example
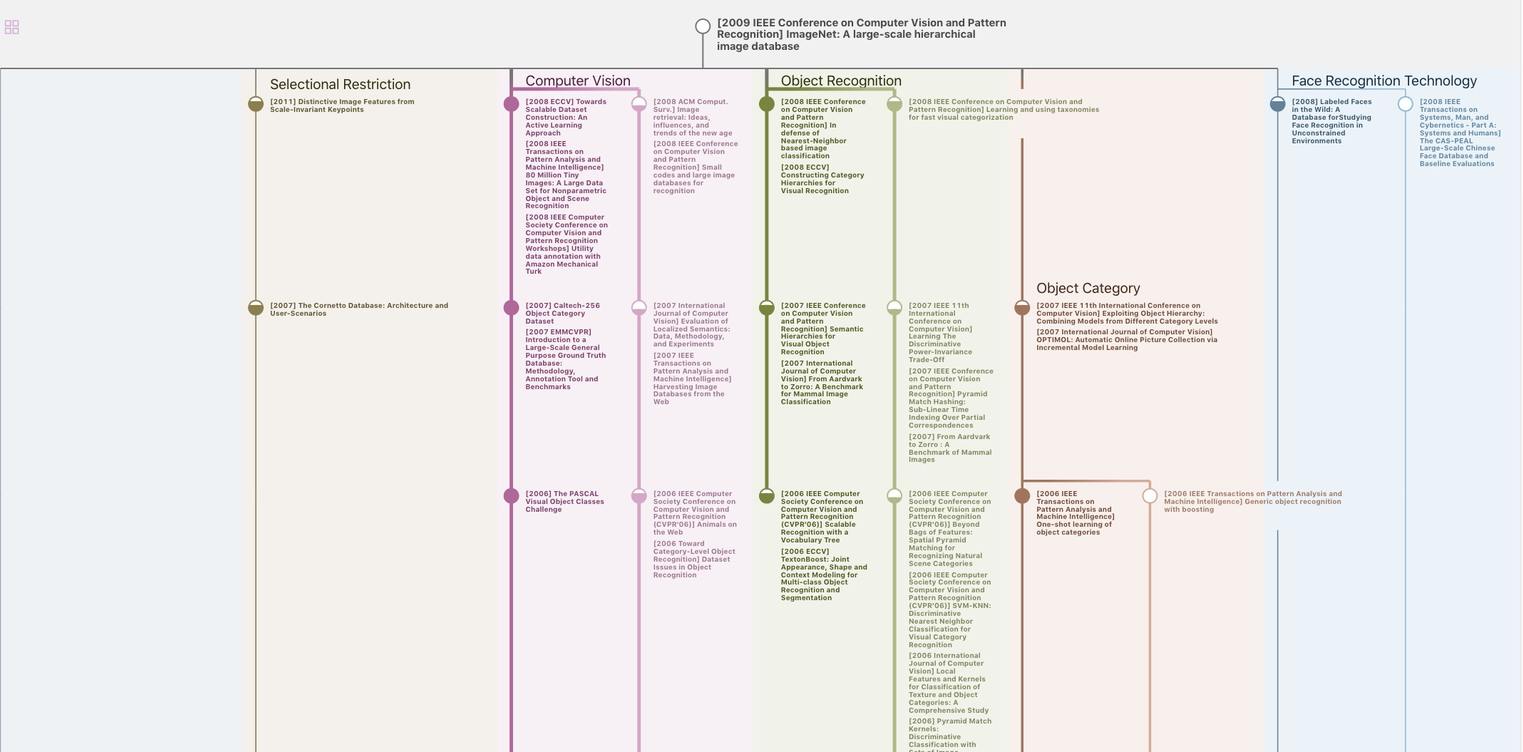
Generate MRT to find the research sequence of this paper
Chat Paper
Summary is being generated by the instructions you defined