Multiple-Instance Metric Learning Network for Hyperspectral Target Detection.
IEEE Trans. Geosci. Remote. Sens.(2023)
Abstract
Target detection becomes increasingly important in hyperspectral image (HSI) analysis but is limited by difficulties in acquiring accurate pixel-level training labels. This article proposes a multiple-instance metric learning neural network (MIML-Net) for hyperspectral target detection tasks, which only requires region-level labels and greatly alleviates the laborious pixel-level annotation problems. Our method learns the embeddings of regions with weak labels under attention-based multiple-instance learning (MIL) framework. Based on which, we impose a novel metric-based regularizer to constrain target and background embeddings to two learnable compact clusters with distinct centroids, which further boosts the spectral feature representation ability. The proposed metric-based regularizer enforces a discriminative detector due to its capability to reduce the intraclass variations and encourage the interclass separations simultaneously. Extensive experimental results from both simulated and real-field datasets demonstrate the effectiveness of the proposed MIML-Net in comparison with the state-of-the-art weakly supervised techniques.
MoreTranslated text
AI Read Science
Must-Reading Tree
Example
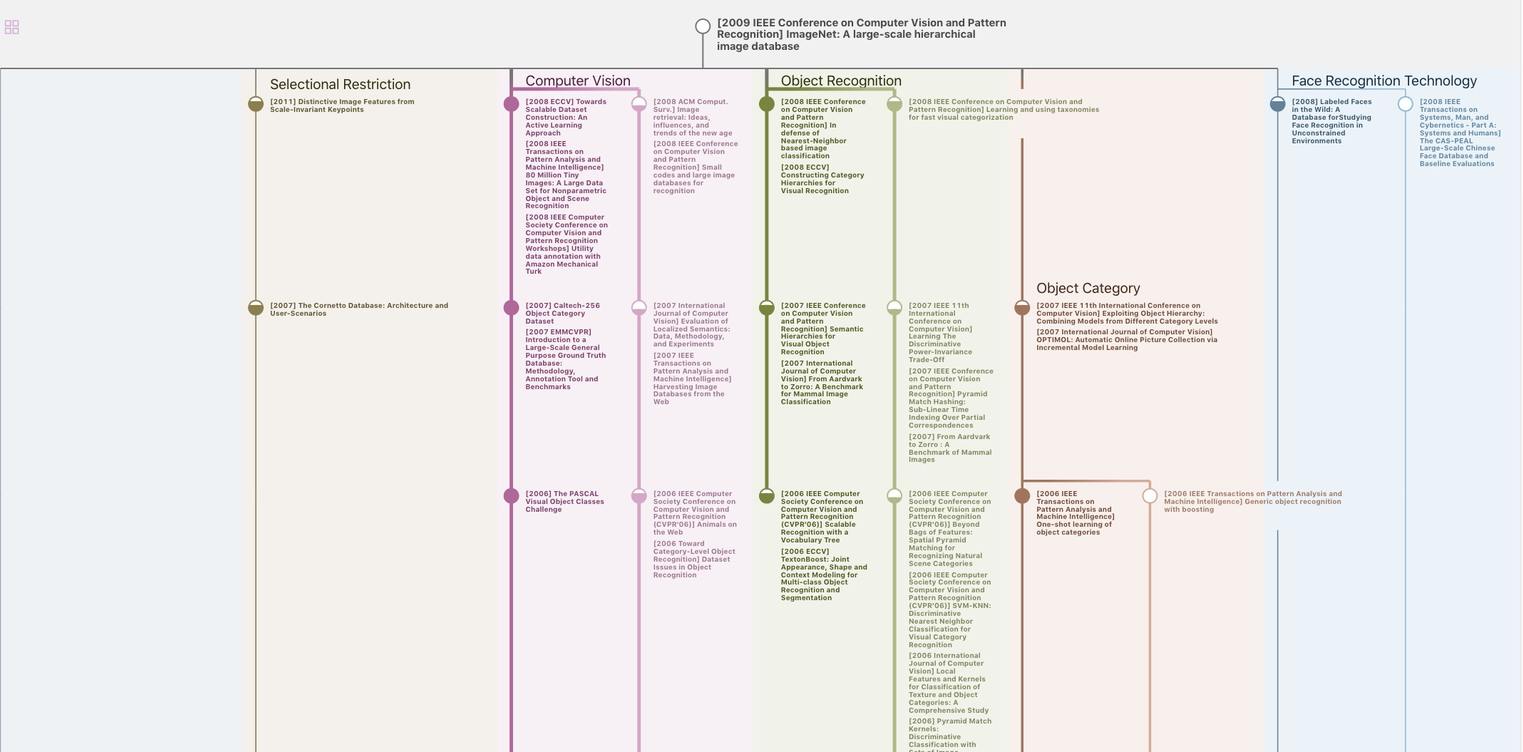
Generate MRT to find the research sequence of this paper
Chat Paper
Summary is being generated by the instructions you defined