Galectin-3-ITGB1 Signaling Mediates Interleukin 10 Production of Hepatic Conventional Natural Killer Cells in Hepatitis B Virus Transgenic Mice and Correlates with Hepatocellular Carcinoma Progression in Patients.
Viruses(2024)
Abstract
BACKGROUND AND AIMS:The outcomes of HBV infections are related to complex immune imbalances; however, the precise mechanisms by which HBV induces immune dysfunction are not well understood.
METHODS:HBV transgenic (HBs-Tg) mice were used to investigate intrahepatic NK cells in two distinct subsets: conventional NK (cNK) and liver-resident NK (LrNK) cells during a chronic HBV infection.
RESULTS:The cNK cells, but not the LrNK cells, were primarily responsible for the increase in the number of bulk NK cells in the livers of ageing HBs-Tg mice. The hepatic cNK cells showed a stronger ability to produce IL-10, coupled with a higher expression of CD69, TIGIT and PD-L1, and lower NKG2D expression in ageing HBs-Tg mice. A lower mitochondrial mass and membrane potential, and less polarized localization were observed in the hepatic cNK cells compared with the splenic cNK cells in the HBs-Tg mice. The enhanced galectin-3 (Gal-3) secreted from HBsAg+ hepatocytes accounted for the IL-10 production of hepatic cNK cells via ITGB1 signaling. For humans, LGALS3 and ITGB1 expression is positively correlated with IL-10 expression, and negatively correlated with the poor clinical progression of HCC.
CONCLUSIONS:Gal-3-ITGB1 signaling shapes hepatic cNK cells but not LrNK cells during a chronic HBV infection, which may correlate with HCC progression.
MoreTranslated text
Key words
HBV,natural killer cell,galectin-3,ITGB1,interleukin 10
AI Read Science
Must-Reading Tree
Example
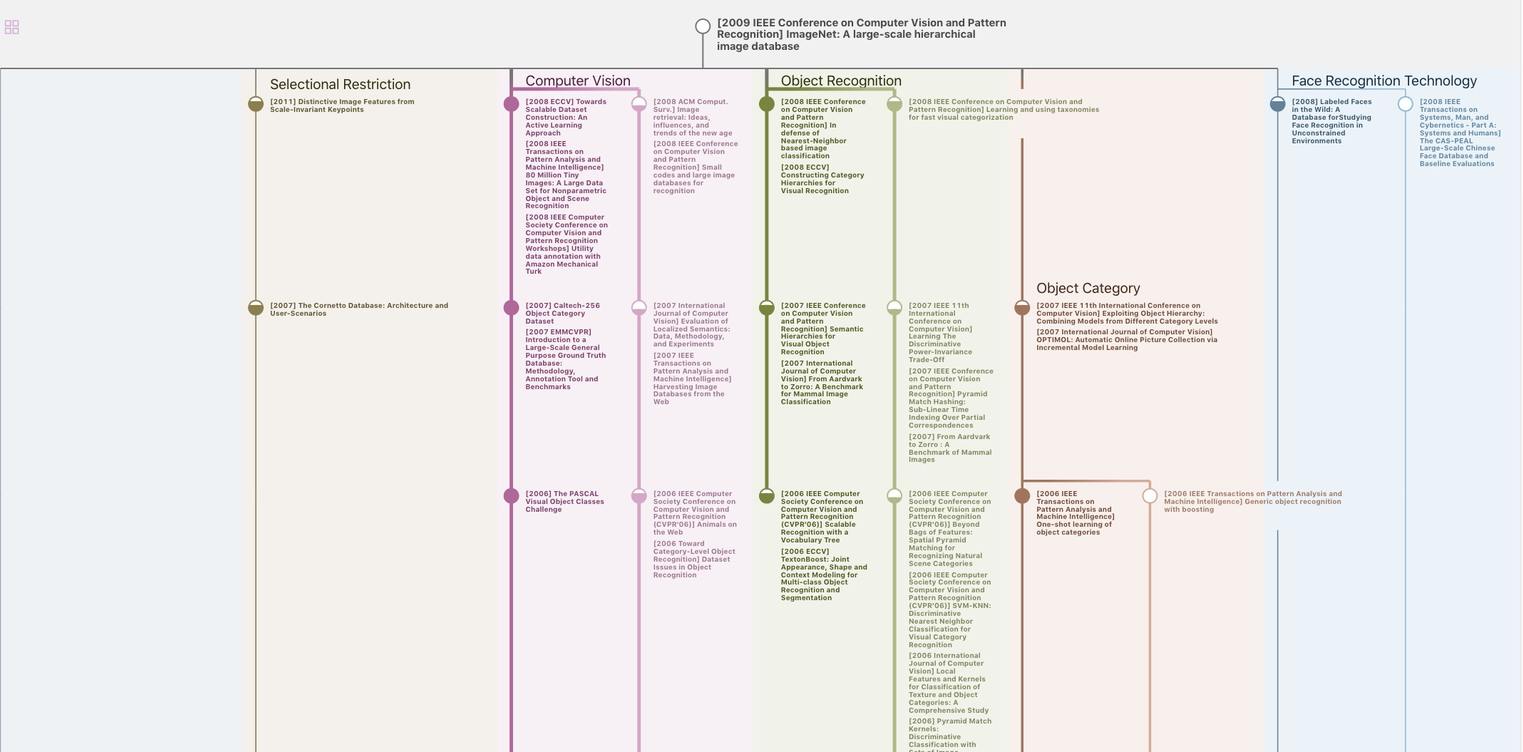
Generate MRT to find the research sequence of this paper
Chat Paper
Summary is being generated by the instructions you defined