Unrolling Nonnegative Matrix Factorization With Group Sparsity for Blind Hyperspectral Unmixing.
IEEE Trans. Geosci. Remote. Sens.(2023)
摘要
Deep neural networks have shown huge potential in hyperspectral unmixing (HU). However, the large function space increases the difficulty of obtaining the optimal solution with limited unmixing data. The autoencoder-based blind unmixing methods are sensitive to the hyperparameters, and the optimal solution can be difficult to obtain. Algorithm unrolling, which integrates deep learning and iterative algorithms, can shrink the search space and improve the efficiency of obtaining optimal results. Based on this, a model-driven deep neural network named the group sparsity regularized unmixing unrolling (GSUU) network, which unrolls a regularized matrix factorization objective function for blind HU, is proposed in this article. Based on the nonnegative matrix factorization (NMF) optimization rules, the GSUU network contains two subnetworks-the A-Block and the S-Block-for alternately and iteratively estimating the optimal endmember spectra and abundance maps. The GSUU method incorporates the spatial group sparsity prior to the abundances, i.e., the fact that spatially adjacent mixed pixels share similar sparse abundances, into a deep unrolling network. The experimental results obtained with both synthetic and real hyperspectral data illustrate that the proposed algorithm can obtain a superior accuracy, compared to the other state-of-the-art unmixing algorithms.
更多查看译文
关键词
Deep neural network, deep unrolling, group sparsity, hyperspectral unmixing (HU)
AI 理解论文
溯源树
样例
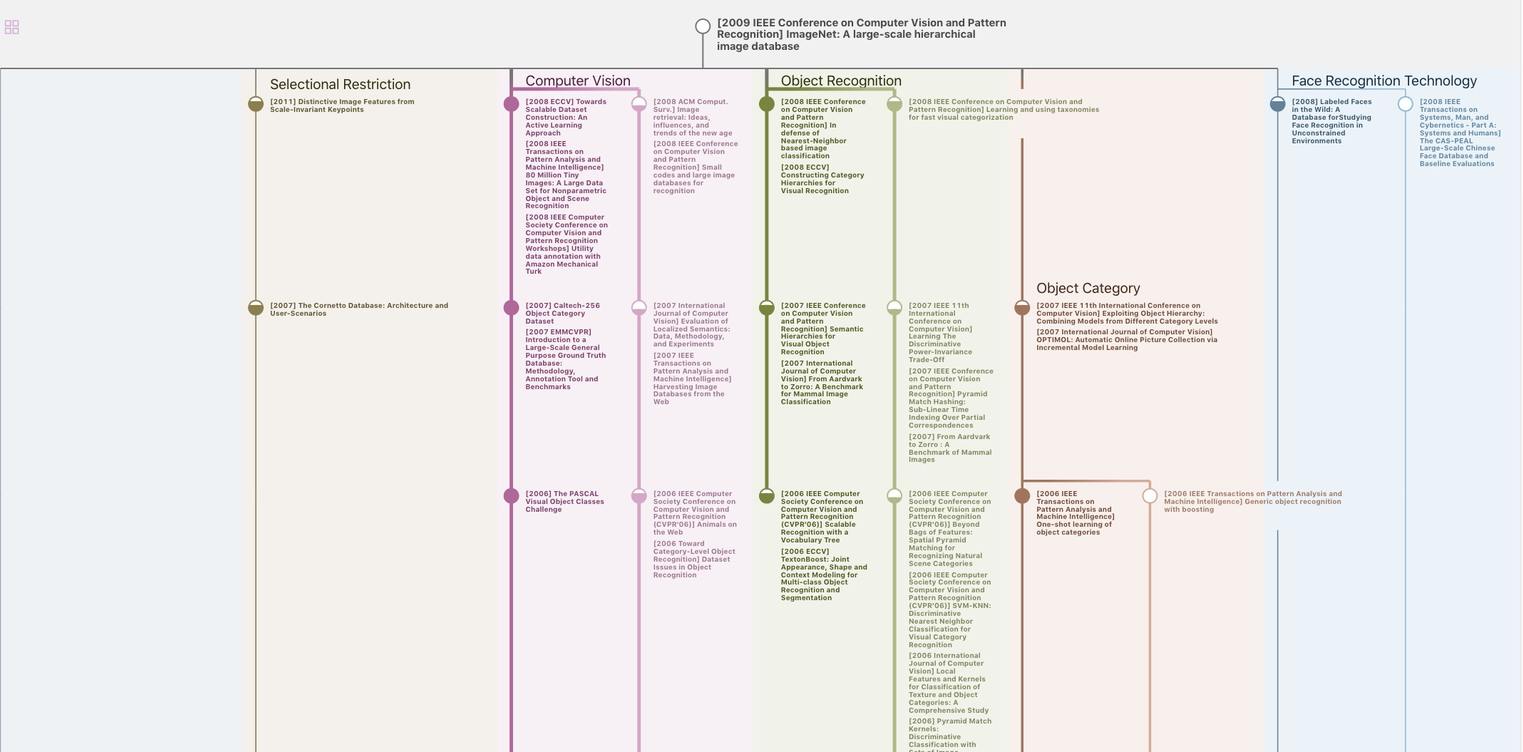
生成溯源树,研究论文发展脉络
Chat Paper
正在生成论文摘要