Data-Driven RBFNN-Enhanced Model-Free Adaptive Traffic Symmetrical Signal Control for a Multi-Phase Intersection with Fast-Changing Traffic Flow.
Symmetry(2023)
摘要
Fast-changing demand in real traffic systems always leads to asymmetrical traffic flow and queues, which aggravates congestion and energy waste. In this paper, the traffic signal control problem of multi-phase intersections was studied with fast-changing traffic flows. First, a novel model-free adaptive control-based symmetrical queuing balancing method was designed by using the full-format dynamic linearization (FFDL) technique. Second, in order to deal with the fast-changing traffic flow, a radial basis function neural network (RBFNN) was added to adjust parameters in a two-layer structure. Moreover, a variable cycle tuning algorithm was introduced to further reduce the time loss. Using the simulation, the proposed algorithm was compared with three other control strategies under low and high traffic demand, respectively, and the results showed the capability of the proposed algorithm.
更多查看译文
关键词
data-driven control, traffic signal control, model-free adaptive control, artificial neural network
AI 理解论文
溯源树
样例
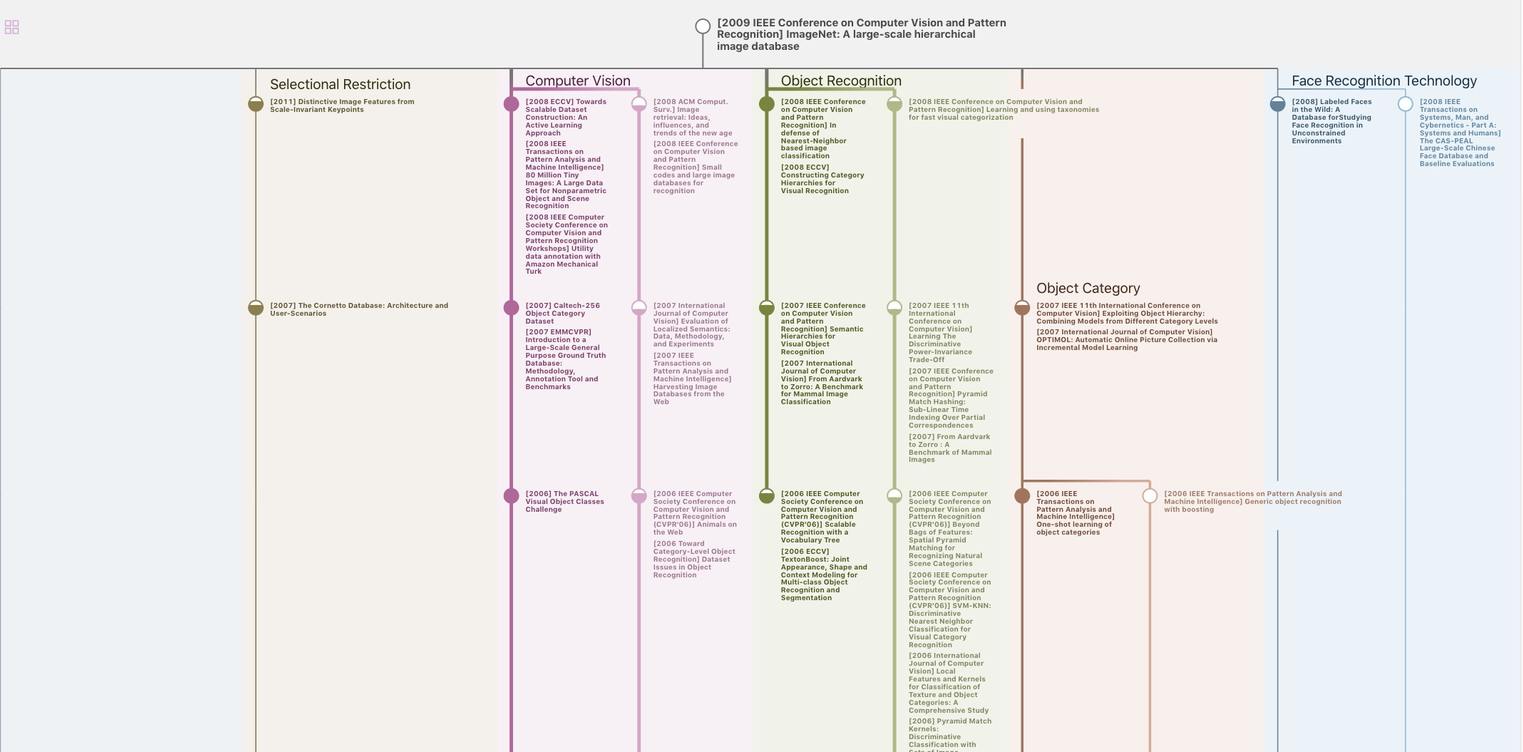
生成溯源树,研究论文发展脉络
Chat Paper
正在生成论文摘要