Robust Kalman and Bayesian Set-Valued Filtering and Model Validation for Linear Stochastic Systems.
SIAM/ASA J. Uncertain. Quantification(2023)
摘要
Consider a linear stochastic filtering problem in which the probability measure specifying all randomness is only partially known. The deviation between the real and assumed probability models is constrained by a divergence bound between the respective probability measures under which the models are defined. This bound defines a so-called uncertainty set. A recursive set-valued filtering characterization is derived and is guaranteed (with probability one) to contain the true conditional posterior of the unknown, real world, filtering problem when the real world measure is within this uncertainty set. Some filtering approximations and related results are given. The set-valued characterization is related to the problem of robust model validation and model goodness-of-fit statistical hypothesis testing. It is shown how relevant terms involving the innovation sequence (re)appear in multiple settings from set-valued filtering to statistical model evaluation.
更多查看译文
关键词
Kalman filtering, robust filtering, set-valued filtering, linear stochastic systems, partial prior information, change of measure
AI 理解论文
溯源树
样例
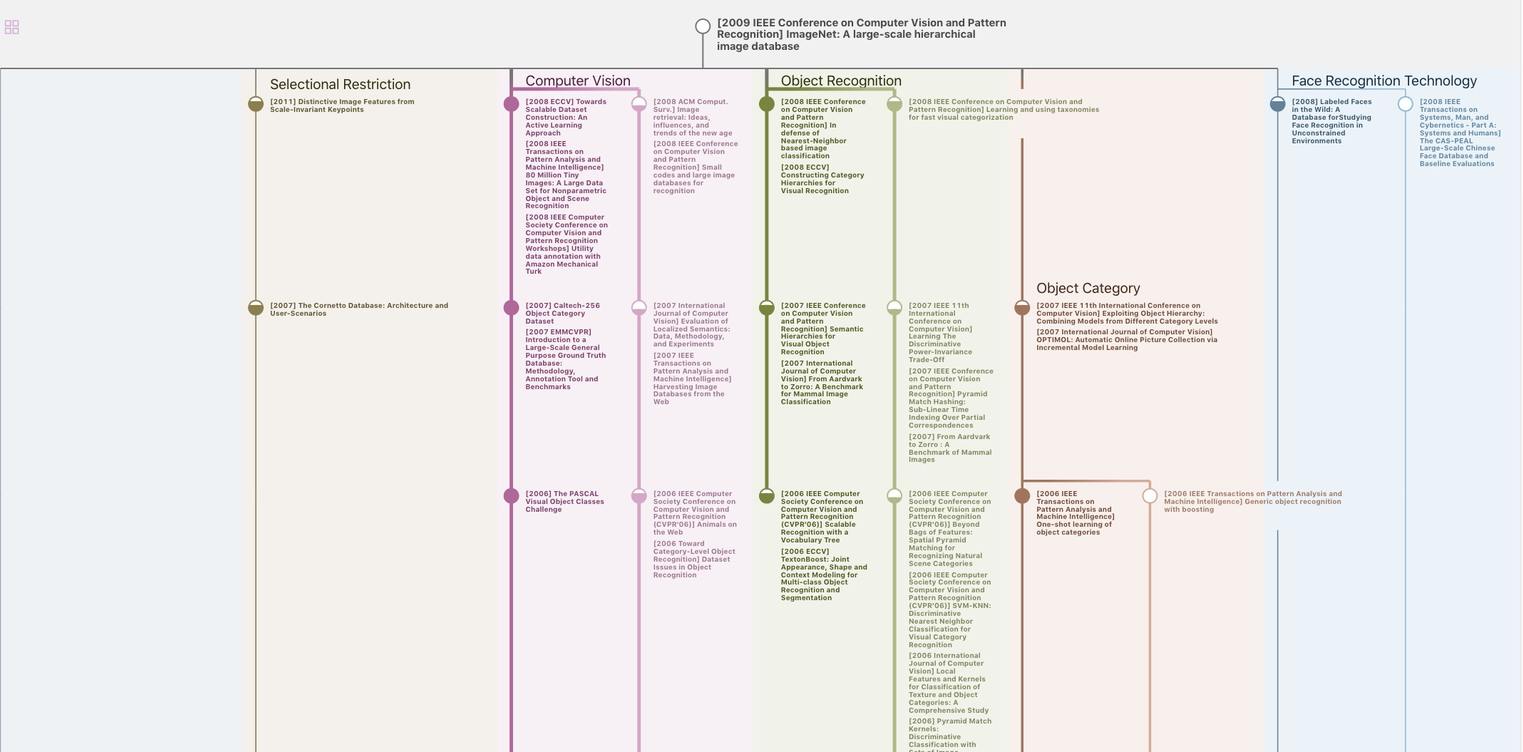
生成溯源树,研究论文发展脉络
Chat Paper
正在生成论文摘要