HIM: An End-to-End Hierarchical Interaction Model for Aspect Sentiment Triplet Extraction
IEEE ACM Trans. Audio Speech Lang. Process.(2023)
摘要
Aspect Sentiment Triplet Extraction (ASTE) is an emerging task of fine-grained sentiment analysis, which aims to extract aspect terms, associated opinion terms, and sentiment polarities in the form of triplets. Thus, ASTE involves two groups of subtasks: aspect/opinion term extraction and aspect-opinion-pair sentiment classification. Due to the high correlations of subtasks, three categories of joint methods have been proposed, including end-to-end tagging-based methods, cascaded span-based methods, and sequence-to-sequence generation-based methods. These methods basically learn either a shared feature space or a shared sentence encoder to capture interactions across all subtasks by parameter sharing. However, they fail to learn deep and mutual interactive features for ASTE. In this work, we present a novel tagging scheme to cast ASTE as a unified boundary-words relation classification problem. Subsequently, we propose an end-to-end Hierarchical Interaction Model (HIM), exploiting deep and mutual interactions across subtasks mainly with two interaction modules. The first-level interaction module primarily leverages multi-task learning models to capture implicit subtask interactions. Then, the second-level interaction module, namely Gated Interaction Network (GIN), adopts a novel gated control mechanism and a newly-designed Conditional BiLSTM (Cond-BiLSTM) network to capture explicit subtask interactions. Moreover, to refine the unreliable outputs of the first-level module, we develop a General word-Pair Relationship Learning (G-PRL) component. With the task-shared features as input, G-PRL further facilitates interactions between term extraction and pair classification. We conduct experiments on two benchmarks and achieve promising results. Extensive analyses demonstrate the effectiveness and flexibility of our work.
更多查看译文
关键词
Task analysis,Tagging,Feature extraction,Logic gates,Multitasking,Data mining,Speech processing,Conditional BiLSTM,end-to-end framework,fine-grained sentiment analysis,gated control mechanism,hierarchical interaction modeling,multi-task learning
AI 理解论文
溯源树
样例
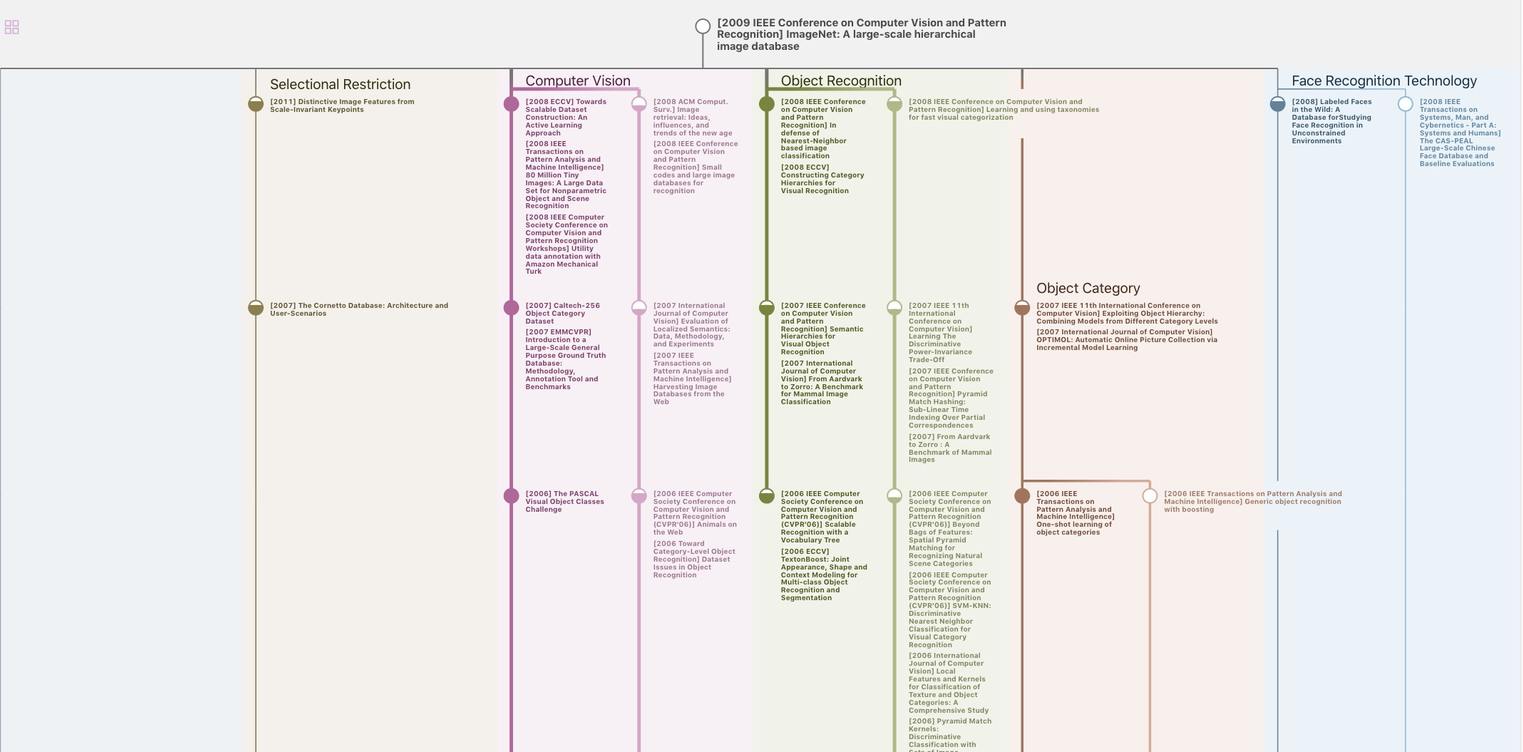
生成溯源树,研究论文发展脉络
Chat Paper
正在生成论文摘要