How Robust are Audio Embeddings for Polyphonic Sound Event Tagging?
IEEE ACM Trans. Audio Speech Lang. Process.(2023)
Abstract
Sound classification algorithms are challenged by the natural variability of everyday sounds, particularly for large sound class taxonomies. In order to be applicable in real-life environments, such algorithms must also be able to handle polyphonic scenarios, where simultaneously occurring and overlapping sound events need to be classified. With the rapid progress of deep learning, several deep audio embeddings (DAEs) have been proposed as pre-trained feature representations for sound classification. In this article, we analyze the embedding spaces of two non-trainable audio representations (NTARs) and five DAEs for sound classification in polyphonic scenarios (sound event tagging) and make several contributions. First, we compare general properties like the inter-correlation between feature dimensions and the scattering of sound classes in the embedding spaces. Second, we test the robustness of the embeddings against several audio degradations and propose two sensitivity measures based on a class-agnostic and a class-centric view on the resulting drift in the embedding space. Finally, as a central contribution, we study how a blending between pairs of sounds maps to embedding space trajectories and how the path of these trajectories can cause classification errors due to their proximity to other sound classes. Throughout our analyses, the PANN embeddings have shown the best overall performance for low-polyphony sound event tagging.
MoreTranslated text
Key words
Sound event tagging, sound polyphony, deep audio embeddings, embedding space
AI Read Science
Must-Reading Tree
Example
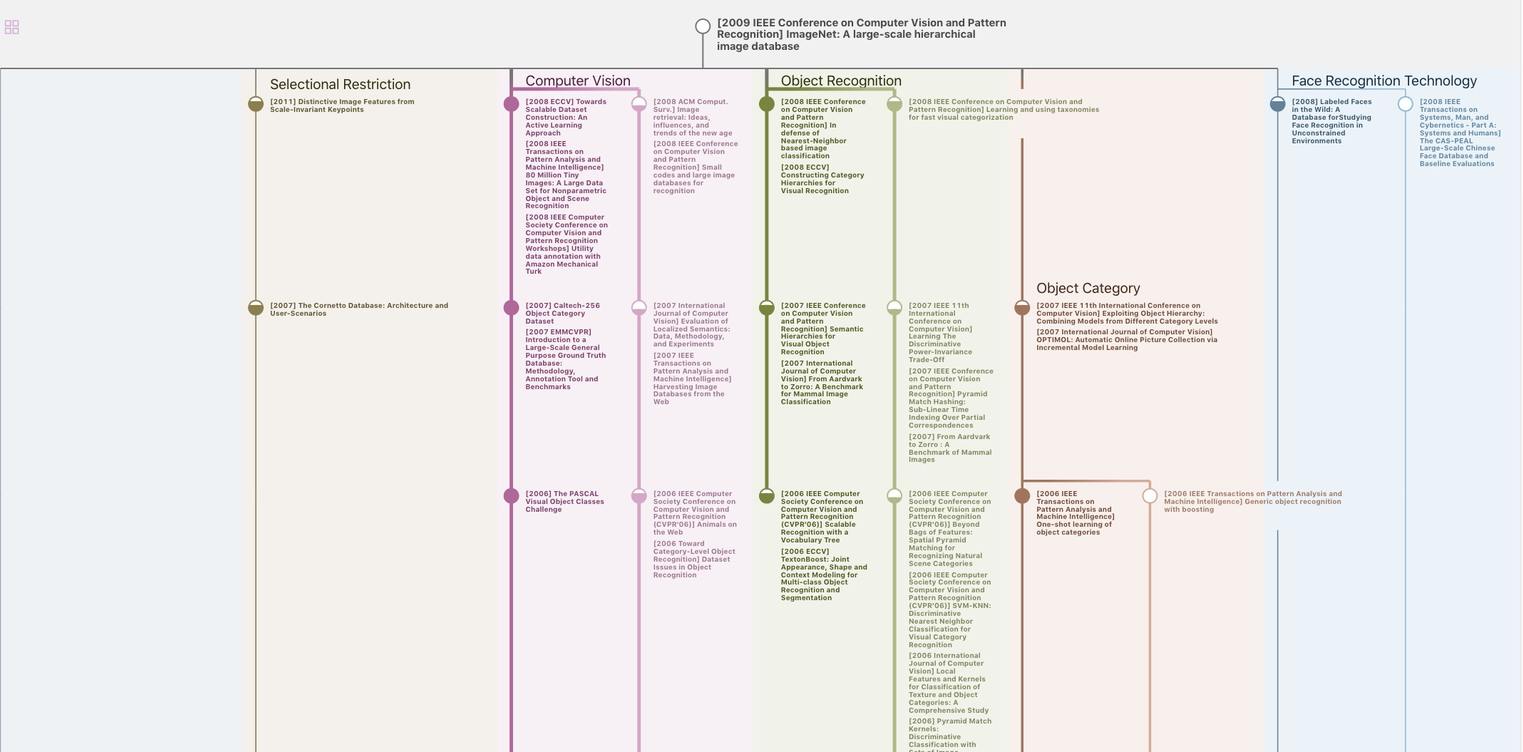
Generate MRT to find the research sequence of this paper
Chat Paper
Summary is being generated by the instructions you defined