Comparison Study Of Two Reinforcement Learning Based Real-Time Control Policies For Two-Machine-One-Buffer Production System
2017 13TH IEEE CONFERENCE ON AUTOMATION SCIENCE AND ENGINEERING (CASE)(2017)
Abstract
Real-time control policy of production system is attractive to reduce the total cost that is mainly composed of the production cost, the penalty of the permanent production loss, and the Work-In-Process(WIP) inventory level cost. Because of the starved and blocked phenomena, the random failures and the maintenances, it is difficult to analyze production system, let alone to find a good control policy. Two reinforcement learning based control decision policies are proposed based on the actions of switching the machines on or off at the start of each time slot. Samples collected from a simulated model are used to obtain two sub-optimal policies named LSPI and TH. TH policy is a simplified form of LSPI, while LSPI performs better in reducing total production cost.
MoreTranslated text
Key words
production loss,sub-optimal policy,two-machine-one-buffer production system,work-in-process inventory level cost,production cost reduction,reinforcement learning based real-time control policy,simulation model,maintenance process,machine failure,TH policy,least-square policy iteration,decision policies
AI Read Science
Must-Reading Tree
Example
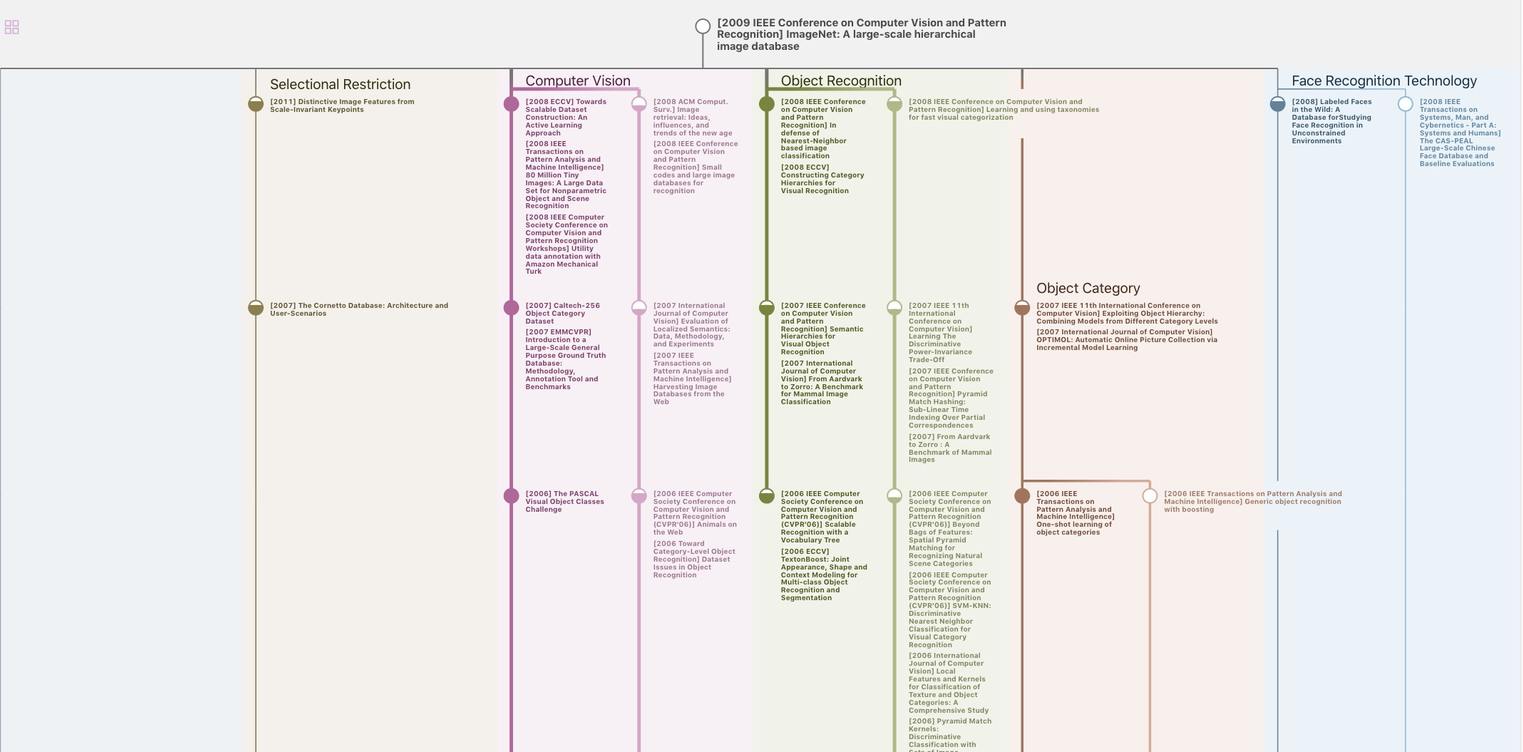
Generate MRT to find the research sequence of this paper
Chat Paper
Summary is being generated by the instructions you defined