Revisiting Fully Convolutional Geometric Features for Object 6D Pose Estimation
2023 IEEE/CVF INTERNATIONAL CONFERENCE ON COMPUTER VISION WORKSHOPS, ICCVW(2023)
Abstract
Recent works on 6D object pose estimation focus on learning keypoint correspondences between images and object models, and then determine the object pose through RANSAC-based algorithms or by directly regressing the pose with end-to-end optimisations. We argue that learning point-level discriminative features is overlooked in the literature. To this end, we revisit Fully Convolutional Geometric Features (FCGF) and tailor it for object 6D pose estimation to achieve state-of-the-art performance. FCGF employs sparse convolutions and learns point-level features using a fully-convolutional network by optimising a hardest contrastive loss. We can outperform recent competitors on popular benchmarks by adopting key modifications to the loss and to the input data representations, by carefully tuning the training strategies, and by employing data augmentations suitable for the underlying problem. We carry out a thorough ablation to study the contribution of each modification. The code is available at https://github.com/jcorsetti/FCGF6D.
MoreTranslated text
Key words
object 6d pose,convolutional geometric features
AI Read Science
Must-Reading Tree
Example
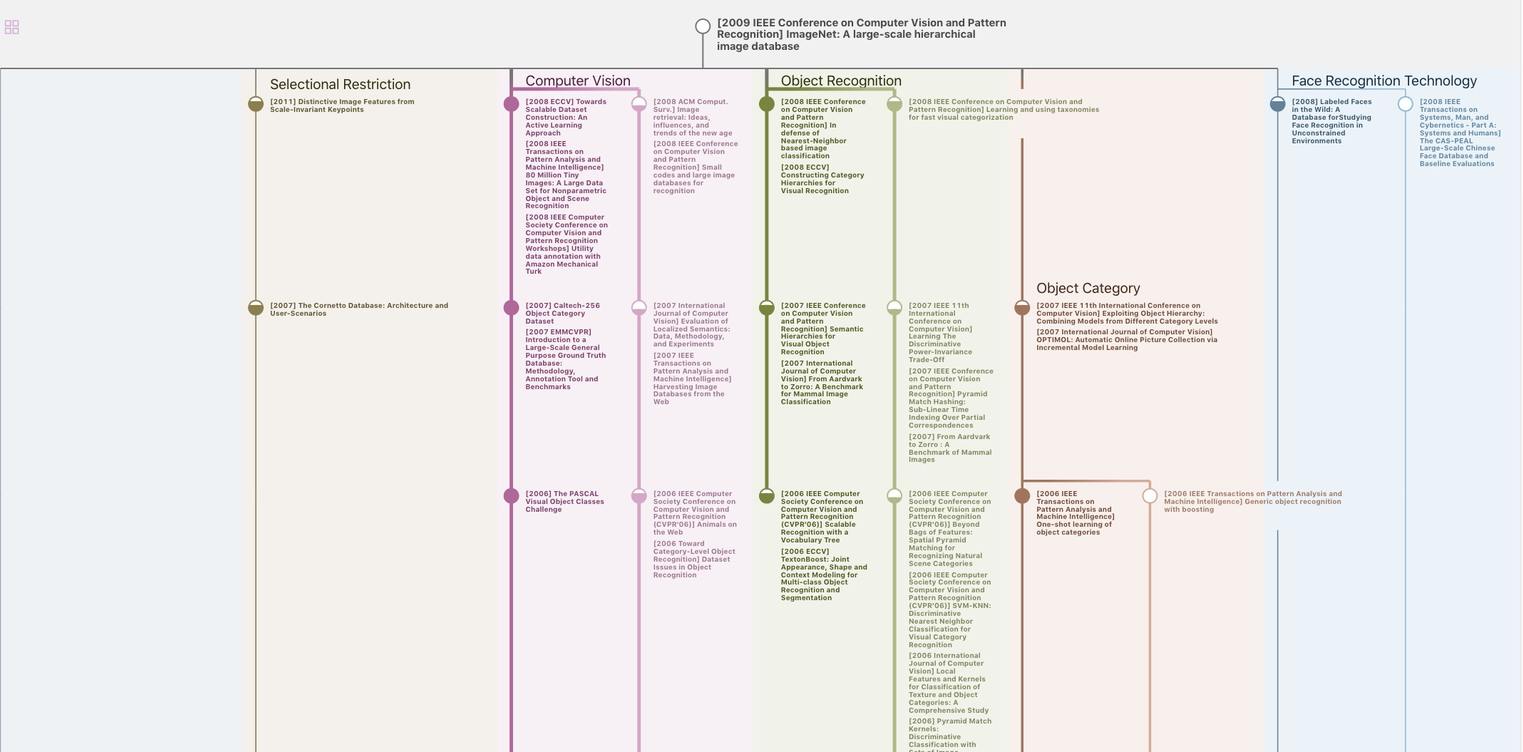
Generate MRT to find the research sequence of this paper
Chat Paper
Summary is being generated by the instructions you defined