Online tree expansion could help solve the problem of scalability in Bayesian phylogenetics
SYSTEMATIC BIOLOGY(2023)
摘要
Bayesian phylogenetics is now facing a critical point. Over the last 20 years, Bayesian methods have reshaped phylogenetic inference and gained widespread popularity due to their high accuracy, the ability to quantify the uncertainty of inferences and the possibility of accommodating multiple aspects of evolutionary processes in the models that are used. Unfortunately, Bayesian methods are computationally expensive, and typical applications involve at most a few hundred sequences. This is problematic in the age of rapidly expanding genomic data and increasing scope of evolutionary analyses, forcing researchers to resort to less accurate but faster methods, such as maximum parsimony and maximum likelihood. Does this spell doom for Bayesian methods? Not necessarily. Here, we discuss some recently proposed approaches that could help scale up Bayesian analyses of evolutionary problems considerably. We focus on two particular aspects: online phylogenetics, where new data sequences are added to existing analyses, and alternatives to Markov chain Monte Carlo (MCMC) for scalable Bayesian inference. We identify 5 specific challenges and discuss how they might be overcome. We believe that online phylogenetic approaches and Sequential Monte Carlo hold great promise and could potentially speed up tree inference by orders of magnitude. We call for collaborative efforts to speed up the development of methods for real-time tree expansion through online phylogenetics.
更多查看译文
关键词
Bayesian inference,MCMC,phylogeny,sequential Monte Carlo
AI 理解论文
溯源树
样例
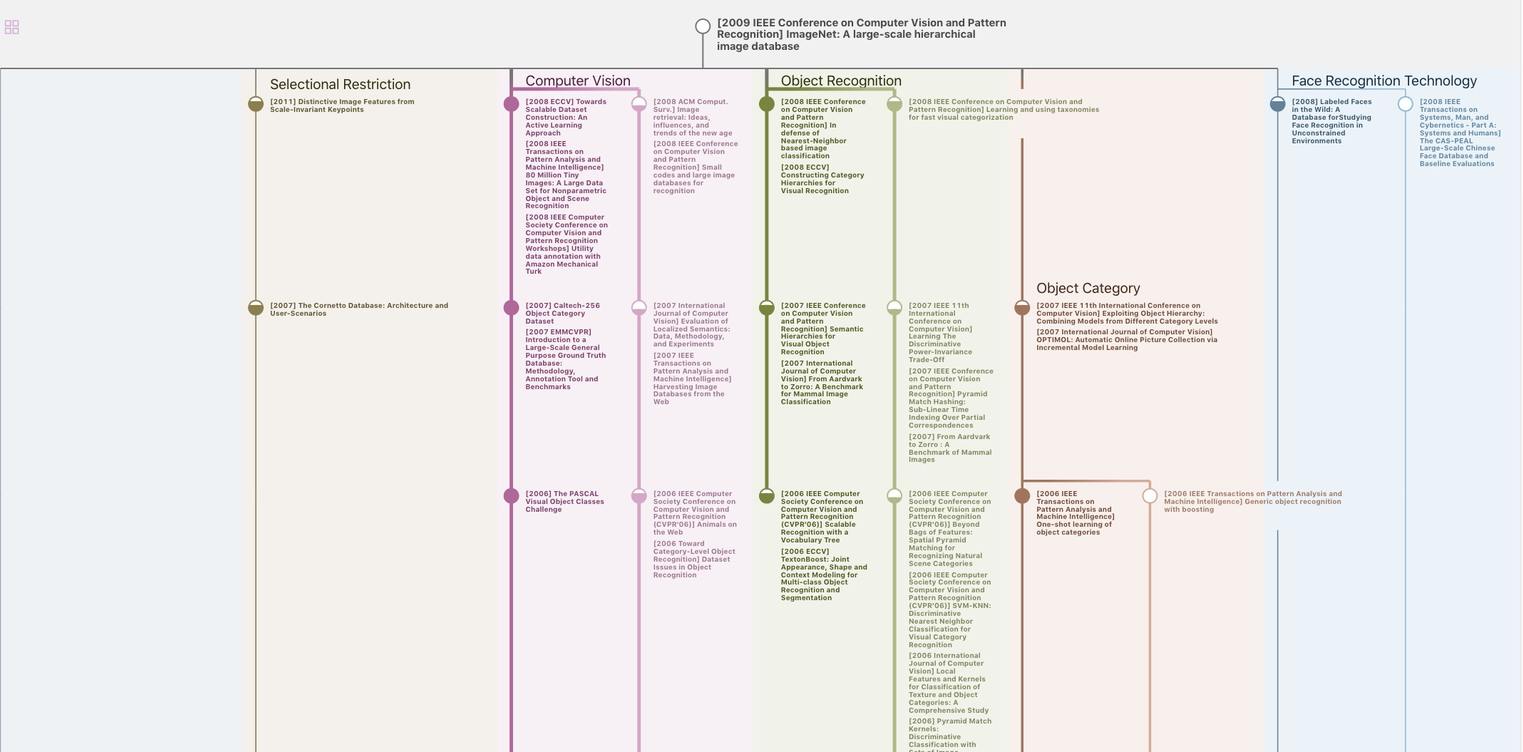
生成溯源树,研究论文发展脉络
Chat Paper
正在生成论文摘要