Material parameter optimization of flax/epoxy composite laminates under low-velocity impact
Composite Structures(2023)
摘要
Since natural fibers have great potential as an alternative to synthetic fibers when the components are impacted at low energies, their mechanical properties under different types of loads need to be investigated. This can be accomplished by using finite element analysis, which is based on the definition of numerical models that reproduce the objects of the physical phenomenon under study. In defining these models, many parameters of the material cards are determined by experimental tests. However, experiments are time-consuming and costly, and it is not always possible to perform all the necessary tests to determine the values for all unknown parameters. For this purpose, the trial-and-error method is usually used. In this work, we present an optimization procedure for predicting the behavior of flax/epoxy composite laminates under low-velocity impact, using the LS-DYNA solver for numerical simulation. The study aims at identifying the values of relevant parameters that allow for predicting the experimental force-displacement trend as accurately as possible and reproducing the damage mechanisms numerically. Each step of the optimization flow is performed with the external tool LS-OPT, using dynamic time warping as a similarity measure to efficiently handle noise. For this purpose, we use the Efficient Global Optimization algorithm, a strategy based on surrogate modeling techniques. We address a multi-target scenario, i.e., we consider several energy levels simultaneously, aiming to find an optimal parameter configuration that is less sensitive to variations in impact energy. The results obtained not only demonstrate the potential of surrogate-based optimization to identify material parameters, but also provide a characterization of the studied composite configuration in view of future applications.
更多查看译文
关键词
Natural-fiber composites,Impact behavior,Finite element analysis,Efficient global optimization,Surrogate models,LS-OPT
AI 理解论文
溯源树
样例
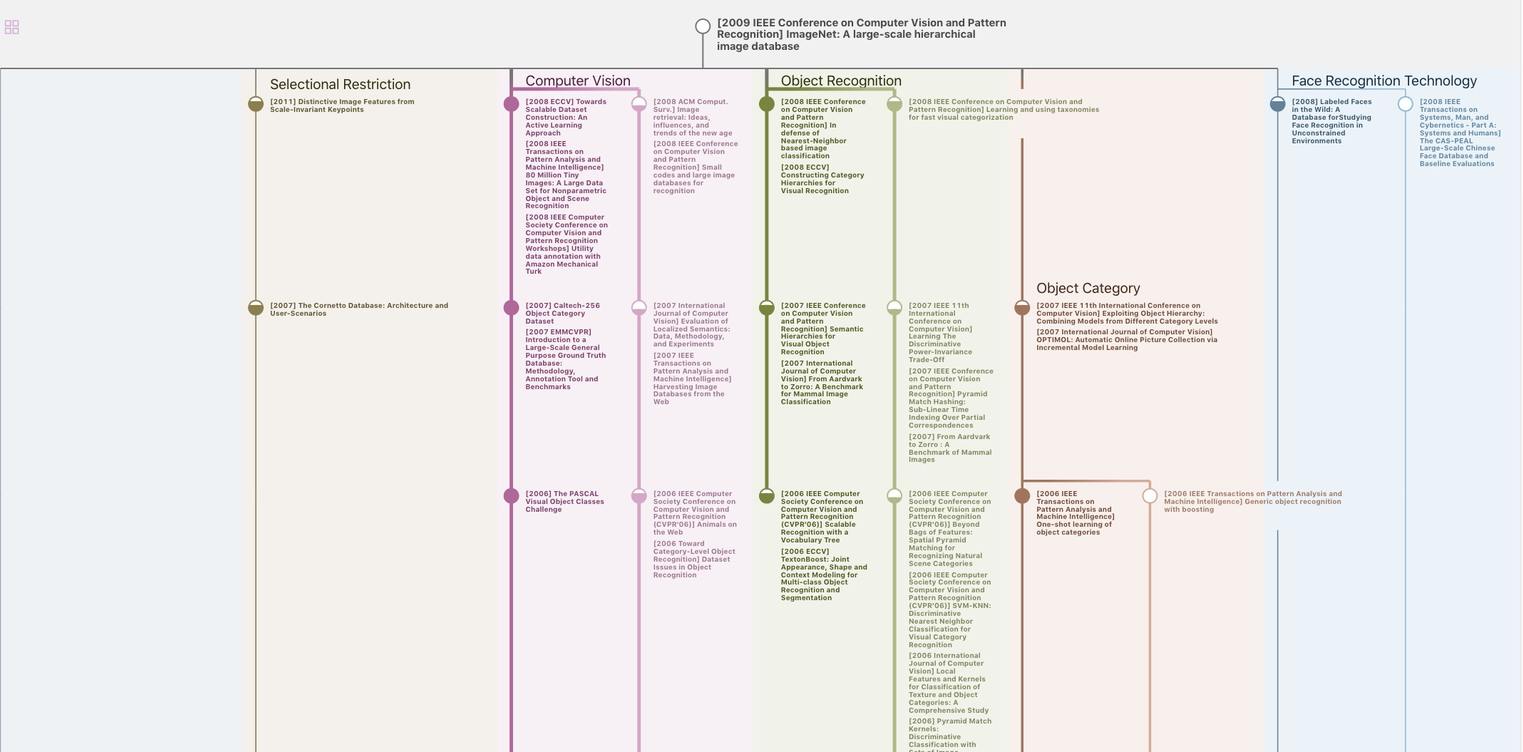
生成溯源树,研究论文发展脉络
Chat Paper
正在生成论文摘要