DeepQMC: An open-source software suite for variational optimization of deep-learning molecular wave functions
JOURNAL OF CHEMICAL PHYSICS(2023)
摘要
Computing accurate yet efficient approximations to the solutions of the electronic Schrodinger equation has been a paramount challenge of computational chemistry for decades. Quantum Monte Carlo methods are a promising avenue of development as their core algorithm exhibits a number of favorable properties: it is highly parallel and scales favorably with the considered system size, with an accuracy that is limited only by the choice of the wave function Ansatz. The recently introduced machine-learned parametrizations of quantum Monte Carlo Ansatze rely on the efficiency of neural networks as universal function approximators to achieve state of the art accuracy on a variety of molecular systems. With interest in the field growing rapidly, there is a clear need for easy to use, modular, and extendable software libraries facilitating the development and adoption of this new class of methods. In this contribution, the DEEPQMC program package is introduced, in an attempt to provide a common framework for future investigations by unifying many of the currently available deep-learning quantum Monte Carlo architectures. Furthermore, the manuscript provides a brief introduction to the methodology of variational quantum Monte Carlo in real space, highlights some technical challenges of optimizing neural network wave functions, and presents example black-box applications of the program package. We thereby intend to make this novel field accessible to a broader class of practitioners from both the quantum chemistry and the machine learning communities.
更多查看译文
关键词
variational optimization,deepqmc deep-learning,molecular,suite,open-source
AI 理解论文
溯源树
样例
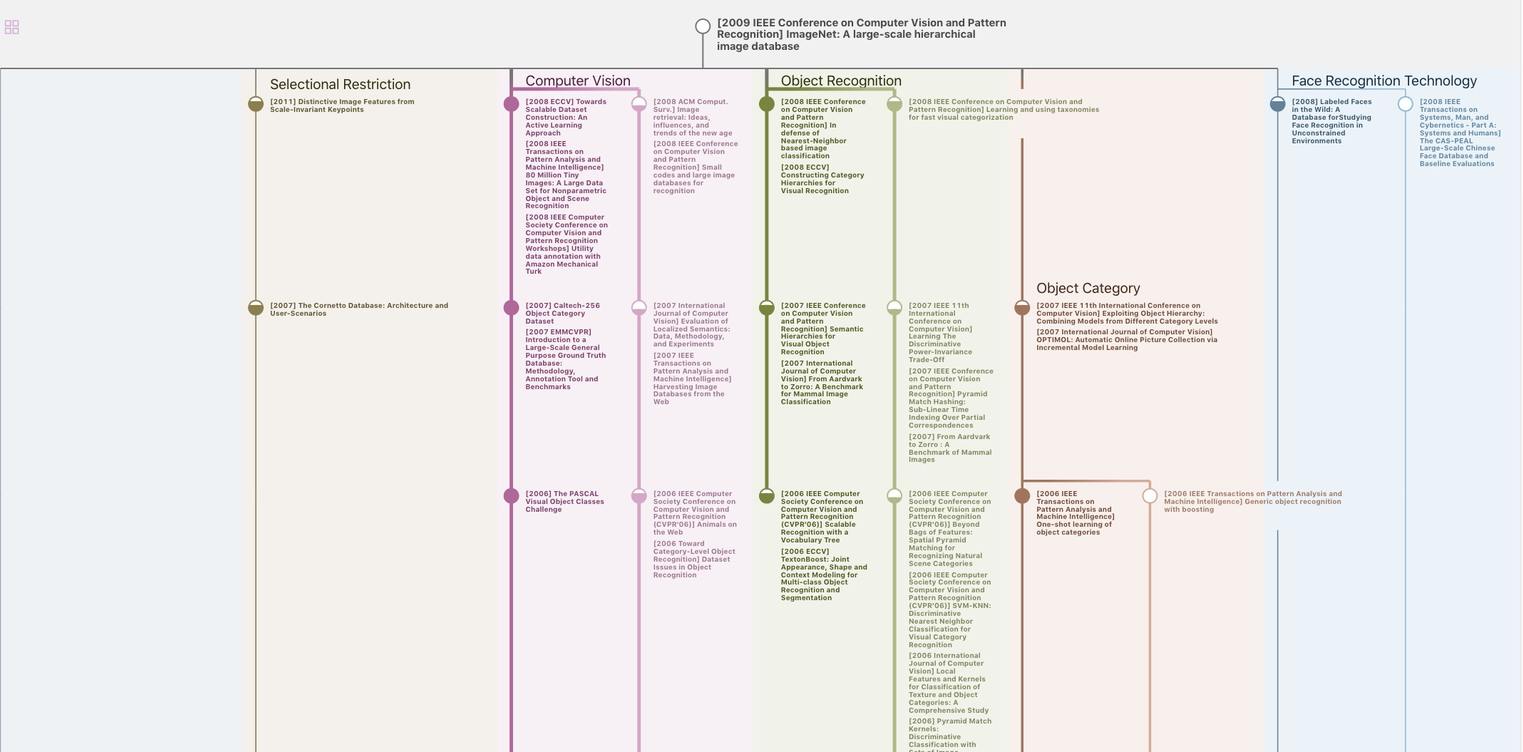
生成溯源树,研究论文发展脉络
Chat Paper
正在生成论文摘要