Are Transformers with One Layer Self-Attention Using Low-Rank Weight Matrices Universal Approximators?
ICLR 2024(2023)
摘要
Existing analyses of the expressive capacity of Transformer models have
required excessively deep layers for data memorization, leading to a
discrepancy with the Transformers actually used in practice. This is primarily
due to the interpretation of the softmax function as an approximation of the
hardmax function. By clarifying the connection between the softmax function and
the Boltzmann operator, we prove that a single layer of self-attention with
low-rank weight matrices possesses the capability to perfectly capture the
context of an entire input sequence. As a consequence, we show that one-layer
and single-head Transformers have a memorization capacity for finite samples,
and that Transformers consisting of one self-attention layer with two
feed-forward neural networks are universal approximators for continuous
permutation equivariant functions on a compact domain.
更多查看译文
关键词
Transformer,Self-Attention,Memorization,Universal Approximation Theorem,Contextual Mapping
AI 理解论文
溯源树
样例
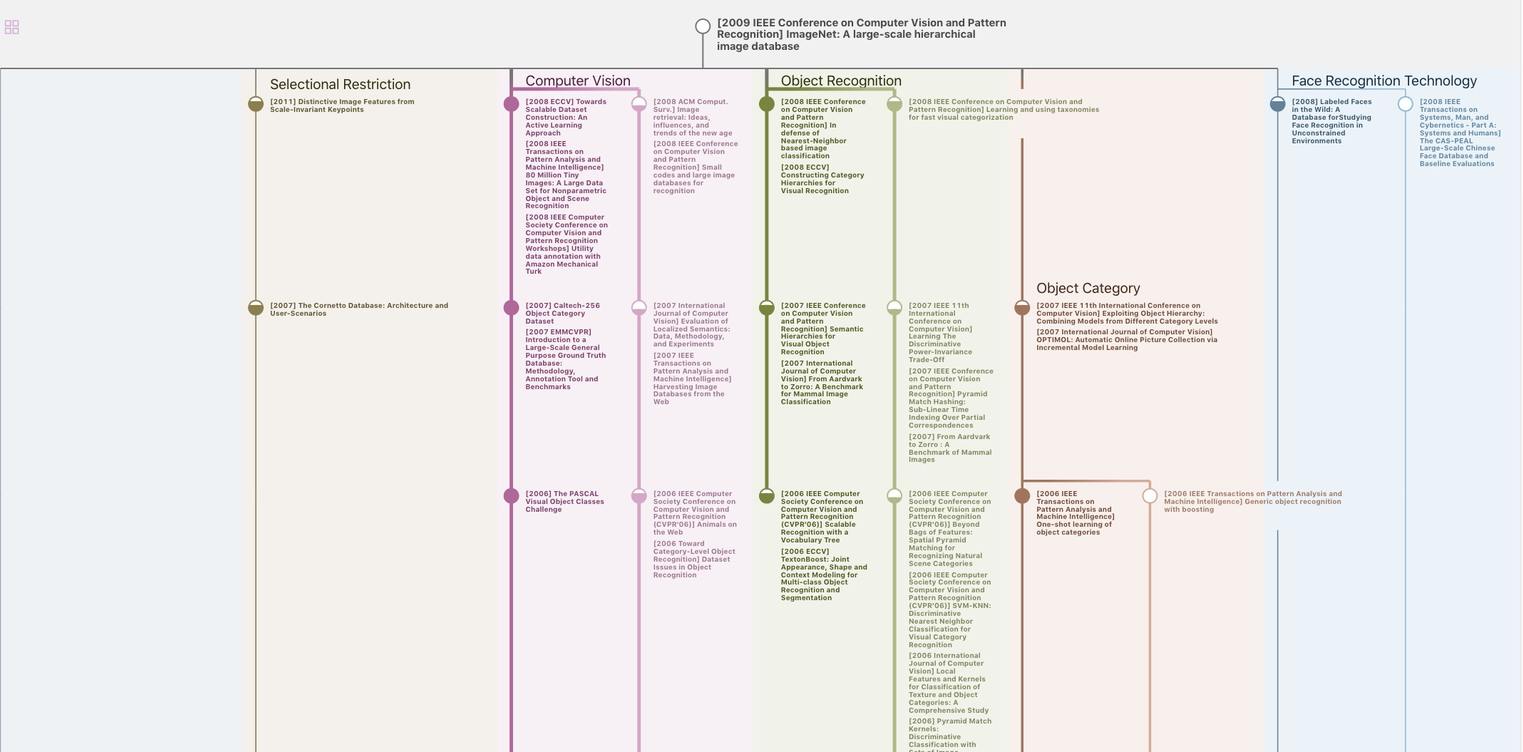
生成溯源树,研究论文发展脉络
Chat Paper
正在生成论文摘要