Deep Learning Based on Connected Vehicles for Icing Pavement Detection
AI in Civil Engineering(2023)
关键词
Connected vehicles,Deep learning,Long short-term memory network,Pavement conditions,Slippery detection,VISSIM
AI 理解论文
溯源树
样例
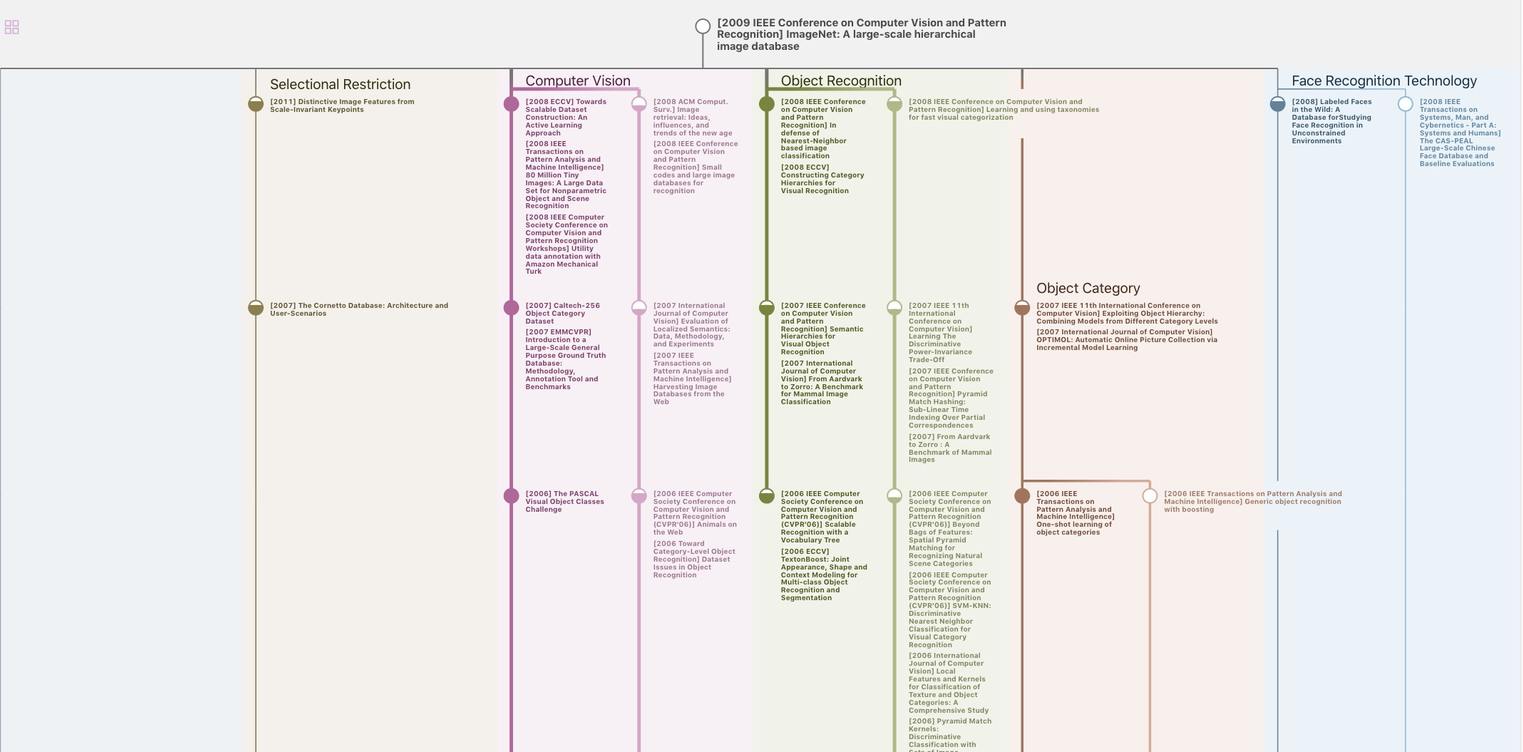
生成溯源树,研究论文发展脉络
Chat Paper
正在生成论文摘要