DIY Your EasyNAS for Vision: Convolution Operation Merging, Map Channel Reducing, and Search Space to Supernet Conversion Tooling.
IEEE transactions on pattern analysis and machine intelligence(2023)
摘要
Despite its popularity as a one-shot Neural Architecture Search (NAS) approach, the applicability of differentiable architecture search (DARTS) on complex vision tasks is still limited by the high computation and memory costs incurred by the over-parameterized supernet. We propose a new architecture search method called EasyNAS, whose memory and computational efficiency is achieved via our devised operator merging technique which shares and merges the weights of candidate convolution operations into a single convolution, and a dynamic channel refinement strategy. We also introduce a configurable search space-to-supernet conversion tool, leveraging the concept of atomic search components, to enable its application from classification to more complex vision tasks: detection and semantic segmentation. In classification, EasyNAS achieves state-of-the-art performance on the NAS-Bench-201 benchmark, attaining an impressive 76.2% accuracy on ImageNet. For detection, it achieves a mean average precision (mAP) of 40.1 with 120 frames per second (FPS) on MS-COCO test-dev. Additionally, we transfer the discovered architecture to the rotation detection task, where EasyNAS achieves a remarkable 77.05 mAP on the DOTA-v1.0 test set, using only 21.1 M parameters. In semantic segmentation, it achieves a competitive mean intersection over union (mIoU) of 72.6% at 173 FPS on Cityscape, after searching for only 0.7 GPU-day.
更多查看译文
关键词
easynas,convolution operation merging,map channel reducing,vision
AI 理解论文
溯源树
样例
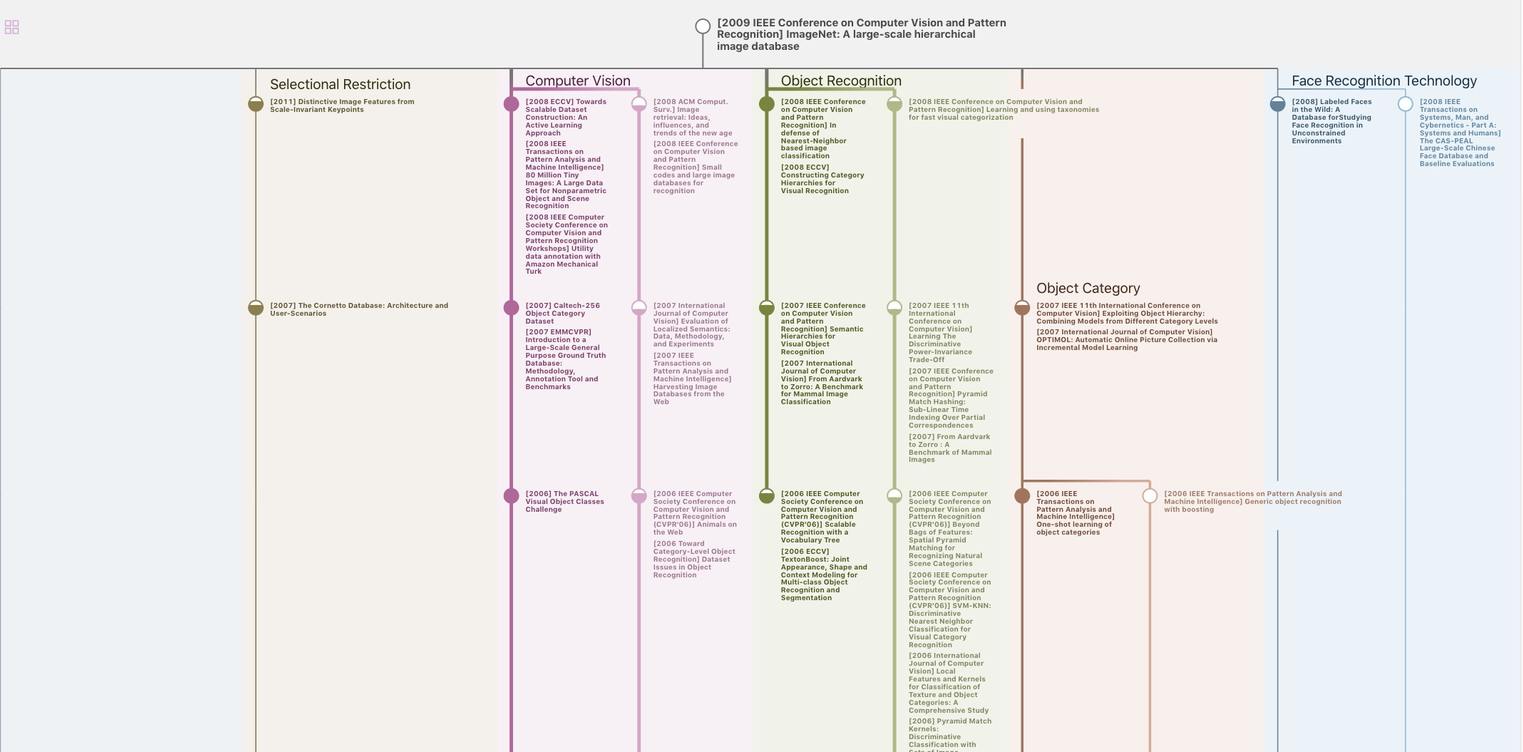
生成溯源树,研究论文发展脉络
Chat Paper
正在生成论文摘要