Scaling up machine learning-based chemical plant simulation: A method for fine-tuning a model to induce stable fixed points
COMPUTERS & CHEMICAL ENGINEERING(2024)
摘要
Idealized first-principles models of chemical plants can be inaccurate. An alternative is to fit a Machine Learning (ML) model directly to plant sensor data. We use a structured approach: Each unit within the plant gets represented by one ML model. After fitting the models to the data, the models are connected into a flowsheet-like directed graph. We find that for smaller plants, this approach works well, but for larger plants, the complex dynamics arising from large and nested cycles in the flowsheet lead to instabilities in the solver during model initialization. We show that a high accuracy of the single -unit models is not enough: The gradient can point in unexpected directions, which prevents the solver from converging to the correct stationary state. To address this problem, we present a way to fine -tune ML models such that initialization, even with very simple solvers, becomes robust.
更多查看译文
关键词
Machine learning,Surrogate model,Flowsheet simulation,End-to-end training,Cycle solving,Fixed point iteration,Model initialization,Digital twin
AI 理解论文
溯源树
样例
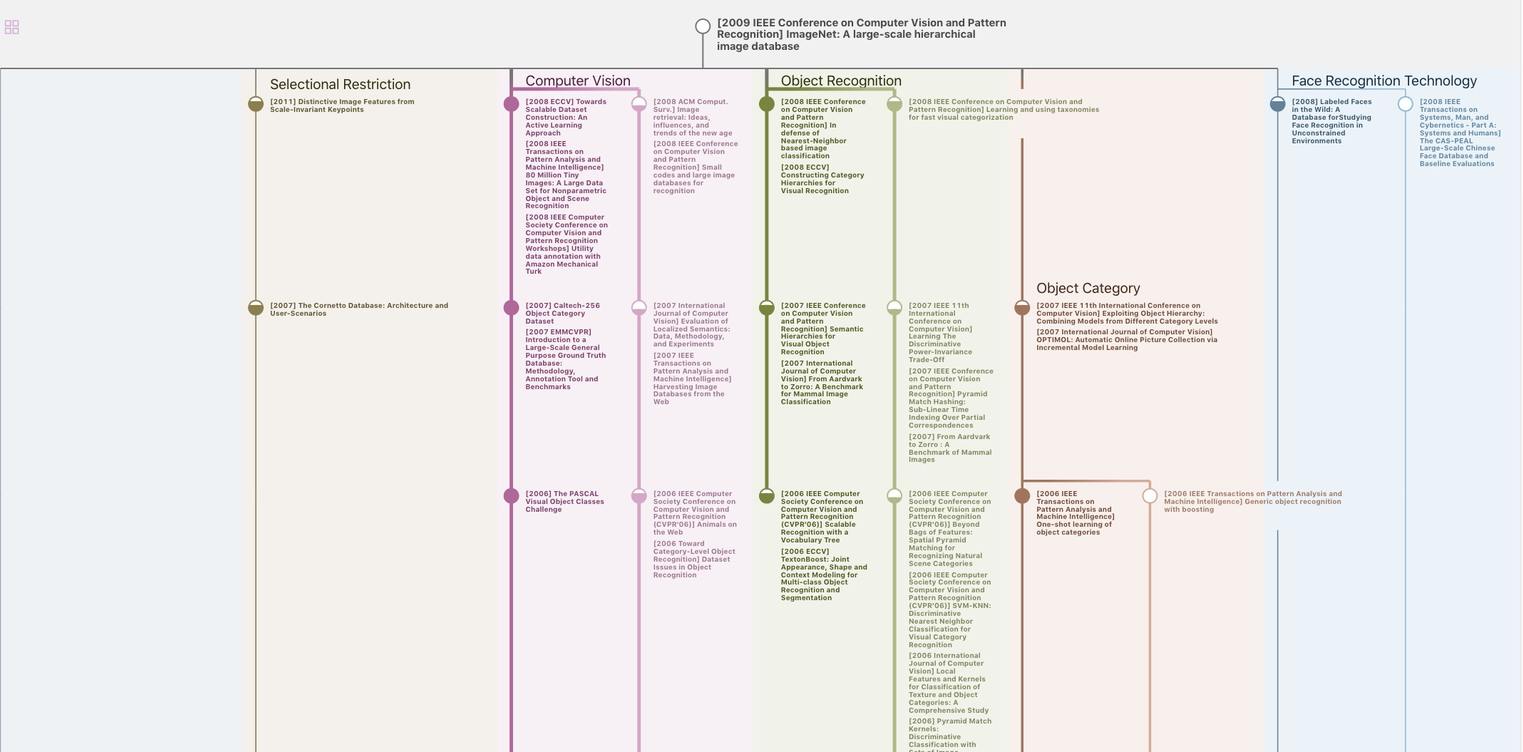
生成溯源树,研究论文发展脉络
Chat Paper
正在生成论文摘要