An Explainable Geometric-Weighted Graph Attention Network for Identifying Functional Networks Associated with Gait Impairment
MEDICAL IMAGE COMPUTING AND COMPUTER ASSISTED INTERVENTION, MICCAI 2023, PT II(2023)
摘要
One of the hallmark symptoms of Parkinson's Disease (PD) is the progressive loss of postural reflexes, which eventually leads to gait difficulties and balance problems. Identifying disruptions in brain function associated with gait impairment could be crucial in better understanding PD motor progression, thus advancing the development of more effective and personalized therapeutics. In this work, we present an explainable, geometric, weighted-graph attention neural network (xGW-GAT) to identify functional networks predictive of the progression of gait difficulties in individuals with PD. xGW-GAT predicts the multi-class gait impairment on the MDS-Unified PD Rating Scale (MDS-UPDRS). Our computational- and data-efficient model represents functional connectomes as symmetric positive definite (SPD) matrices on a Riemannian manifold to explicitly encode pairwise interactions of entire connectomes, based on which we learn an attention mask yielding individual- and group-level explainability. Applied to our resting-state functional MRI (rs-fMRI) dataset of individuals with PD, xGW-GAT identifies functional connectivity patterns associated with gait impairment in PD and offers interpretable explanations of functional subnetworks associated with motor impairment. Our model successfully outperforms several existing methods while simultaneously revealing clinicallyrelevant connectivity patterns. The source code is available at https://github.com/favour-nerrise/xGW-GAT.
更多查看译文
关键词
Resting-state fMRI,Geometric learning,Attention mechanism,Gait impairment,Explainability,Neuroimaging biomarkers
AI 理解论文
溯源树
样例
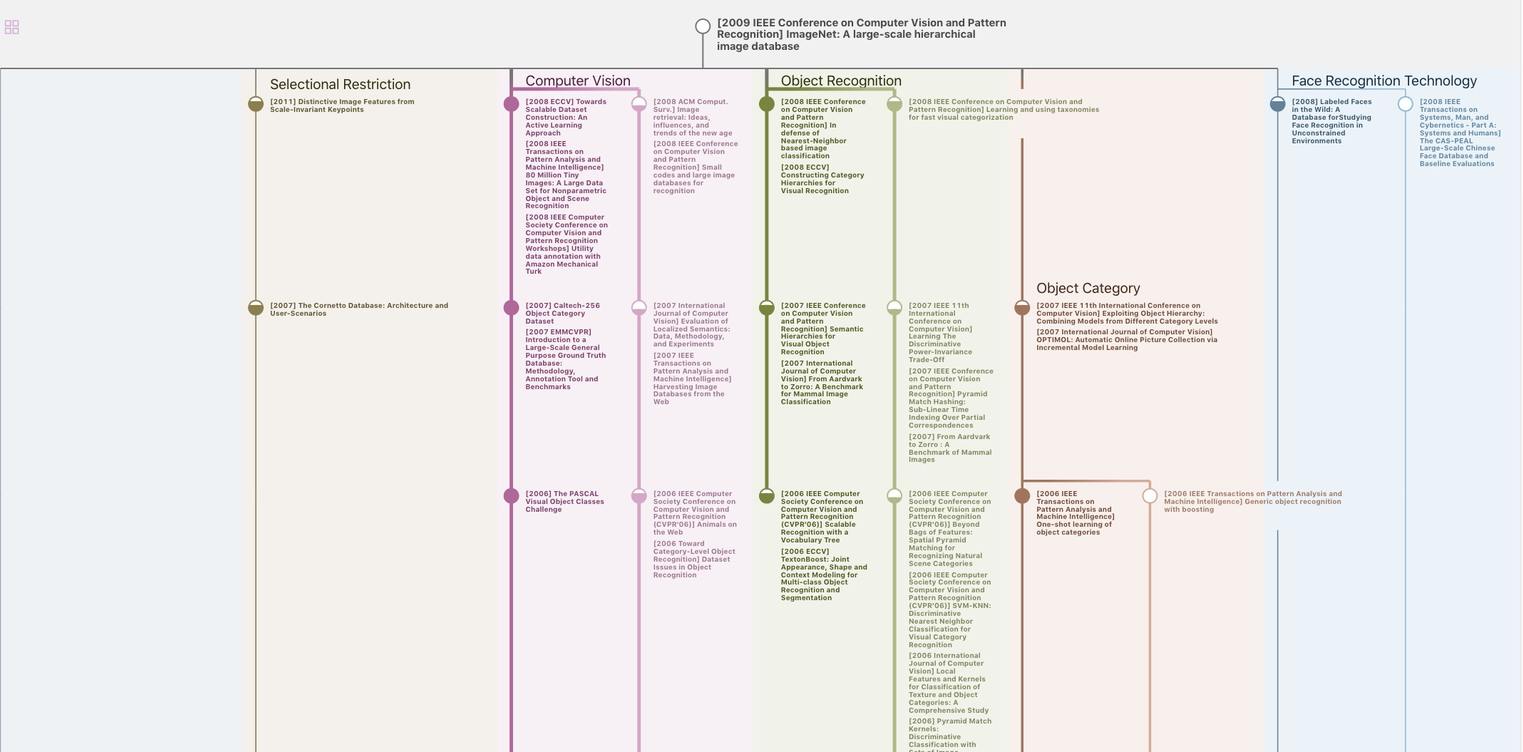
生成溯源树,研究论文发展脉络
Chat Paper
正在生成论文摘要