High-Dimensional Bayesian Likelihood Normalisation for CRESST's Background Model
arXiv (Cornell University)(2023)
摘要
Using CaWO$_4$ crystals as cryogenic calorimeters, the CRESST experiment searches for nuclear recoils caused by the scattering of potential Dark Matter particles. A reliable identification of a potential signal crucially depends on an accurate background model. In this work we introduce an improved normalisation method for CRESST's model of the electromagnetic backgrounds. Spectral templates, based on Geant4 simulations, are normalised via a Bayesian likelihood fit to experimental background data. Contrary to our previous work, no assumption of partial secular equilibrium is required, which results in a more robust and versatile applicability. Furthermore, considering the correlation between all background components allows us to explain 82.7% of the experimental background within [1 keV, 40 keV], an improvement of 18.6% compared to our previous method.
更多查看译文
关键词
normalisation,cresst
AI 理解论文
溯源树
样例
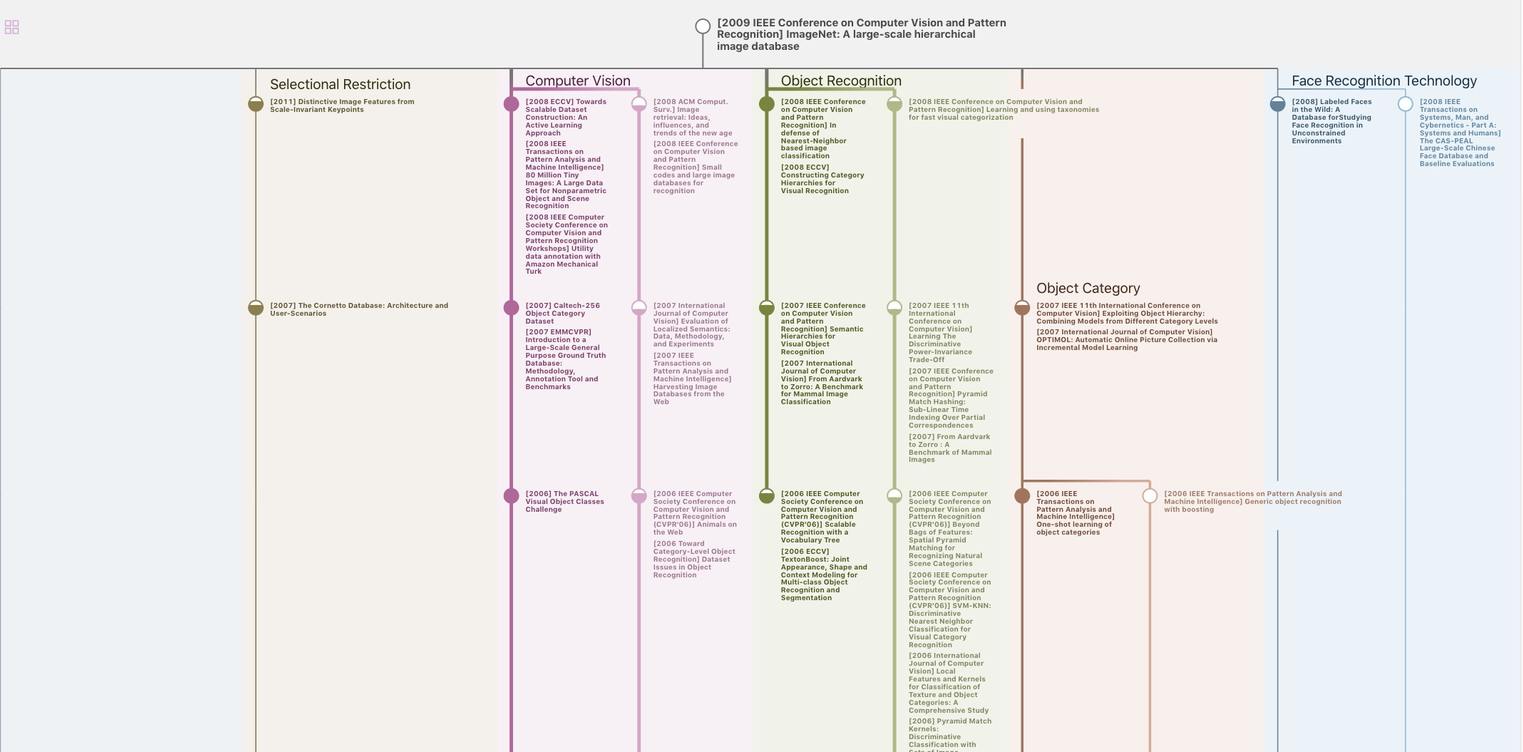
生成溯源树,研究论文发展脉络
Chat Paper
正在生成论文摘要