An Isometric Stochastic Optimizer
CoRR(2023)
摘要
The Adam optimizer is the standard choice in deep learning applications. I propose a simple explanation of Adam's success: it makes each parameter's step size independent of the norms of the other parameters. Based on this principle I derive Iso, a new optimizer which makes the norm of a parameter's update invariant to the application of any linear transformation to its inputs and outputs. I develop a variant of Iso called IsoAdam that allows optimal hyperparameters to be transferred from Adam, and demonstrate that IsoAdam obtains a speedup over Adam when training a small Transformer.
更多查看译文
AI 理解论文
溯源树
样例
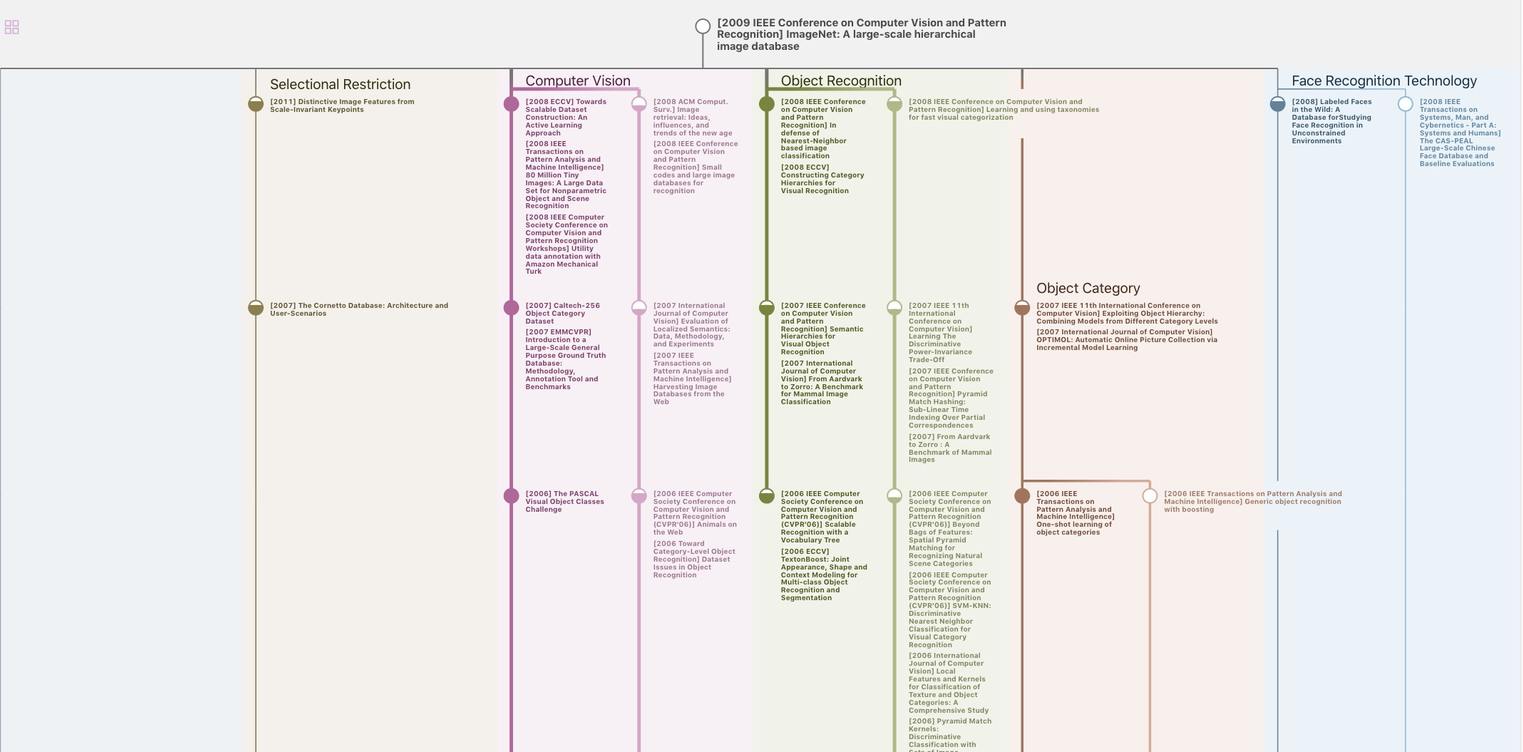
生成溯源树,研究论文发展脉络
Chat Paper
正在生成论文摘要