MC-JEPA: A Joint-Embedding Predictive Architecture for Self-Supervised Learning of Motion and Content Features
CoRR(2023)
Abstract
Self-supervised learning of visual representations has been focusing on learning content features, which do not capture object motion or location, and focus on identifying and differentiating objects in images and videos. On the other hand, optical flow estimation is a task that does not involve understanding the content of the images on which it is estimated. We unify the two approaches and introduce MC-JEPA, a joint-embedding predictive architecture and self-supervised learning approach to jointly learn optical flow and content features within a shared encoder, demonstrating that the two associated objectives; the optical flow estimation objective and the self-supervised learning objective; benefit from each other and thus learn content features that incorporate motion information. The proposed approach achieves performance on-par with existing unsupervised optical flow benchmarks, as well as with common self-supervised learning approaches on downstream tasks such as semantic segmentation of images and videos.
MoreTranslated text
Key words
motion,predictive architecture,learning,features,mc-jepa,joint-embedding,self-supervised
AI Read Science
Must-Reading Tree
Example
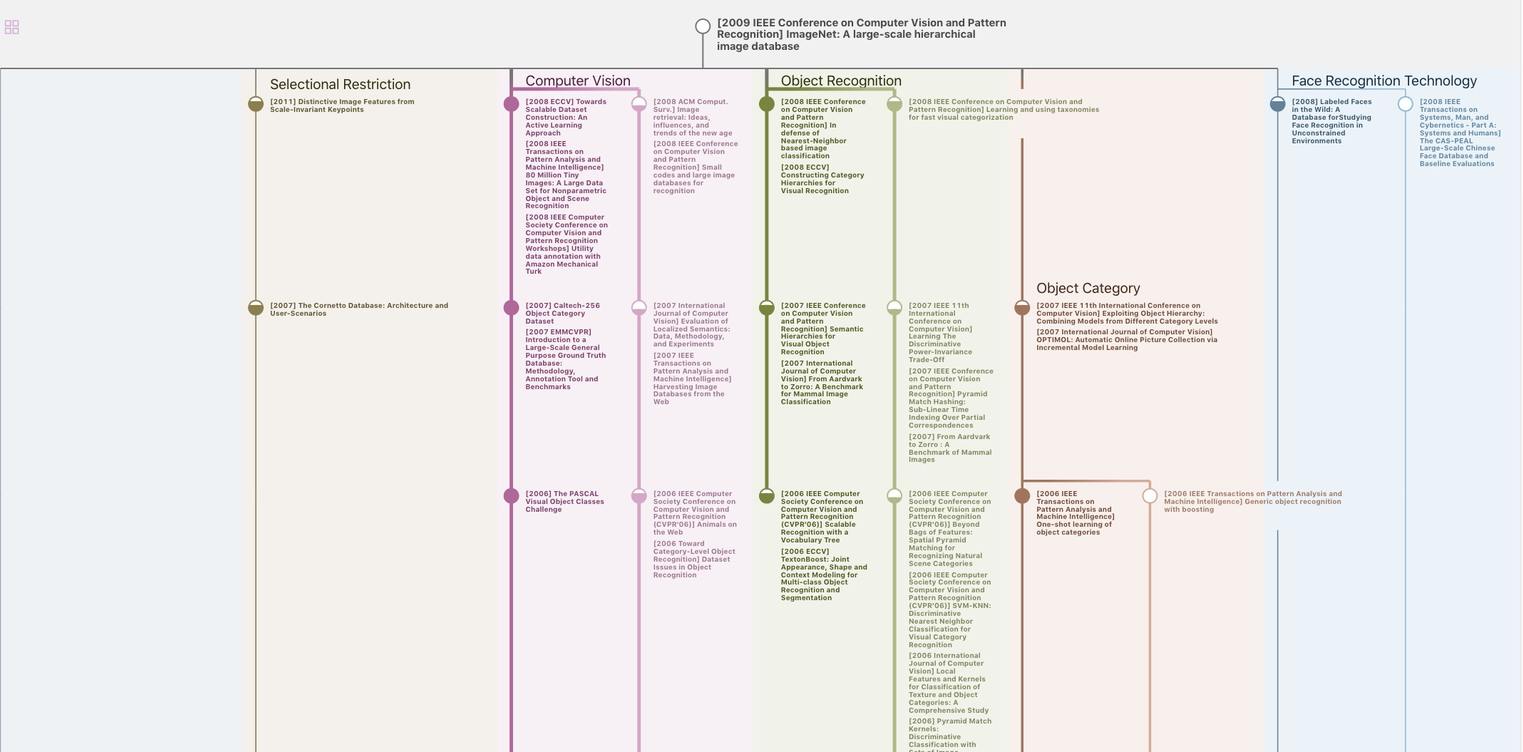
Generate MRT to find the research sequence of this paper
Chat Paper
Summary is being generated by the instructions you defined