WEPRO: Weight Prediction for Efficient Optimization of Hybrid Quantum-Classical Algorithms
CoRR(2023)
摘要
The exponential run time of quantum simulators on classical machines and long queue depths and high costs of real quantum devices present significant challenges in the effective training of Variational Quantum Algorithms (VQAs) like Quantum Neural Networks (QNNs), Variational Quantum Eigensolver (VQE) and Quantum Approximate Optimization Algorithm (QAOA). To address these limitations, we propose a new approach, WEPRO (Weight Prediction), which accelerates the convergence of VQAs by exploiting regular trends in the parameter weights. We introduce two techniques for optimal prediction performance namely, Naive Prediction (NaP) and Adaptive Prediction (AdaP). Through extensive experimentation and training of multiple QNN models on various datasets, we demonstrate that WEPRO offers a speedup of approximately $2.25\times$ compared to standard training methods, while also providing improved accuracy (up to $2.3\%$ higher) and loss (up to $6.1\%$ lower) with low storage and computational overheads. We also evaluate WEPRO's effectiveness in VQE for molecular ground-state energy estimation and in QAOA for graph MaxCut. Our results show that WEPRO leads to speed improvements of up to $3.1\times$ for VQE and $2.91\times$ for QAOA, compared to traditional optimization techniques, while using up to $3.3\times$ less number of shots (i.e., repeated circuit executions) per training iteration.
更多查看译文
关键词
efficient optimization,weight prediction,quantum-classical
AI 理解论文
溯源树
样例
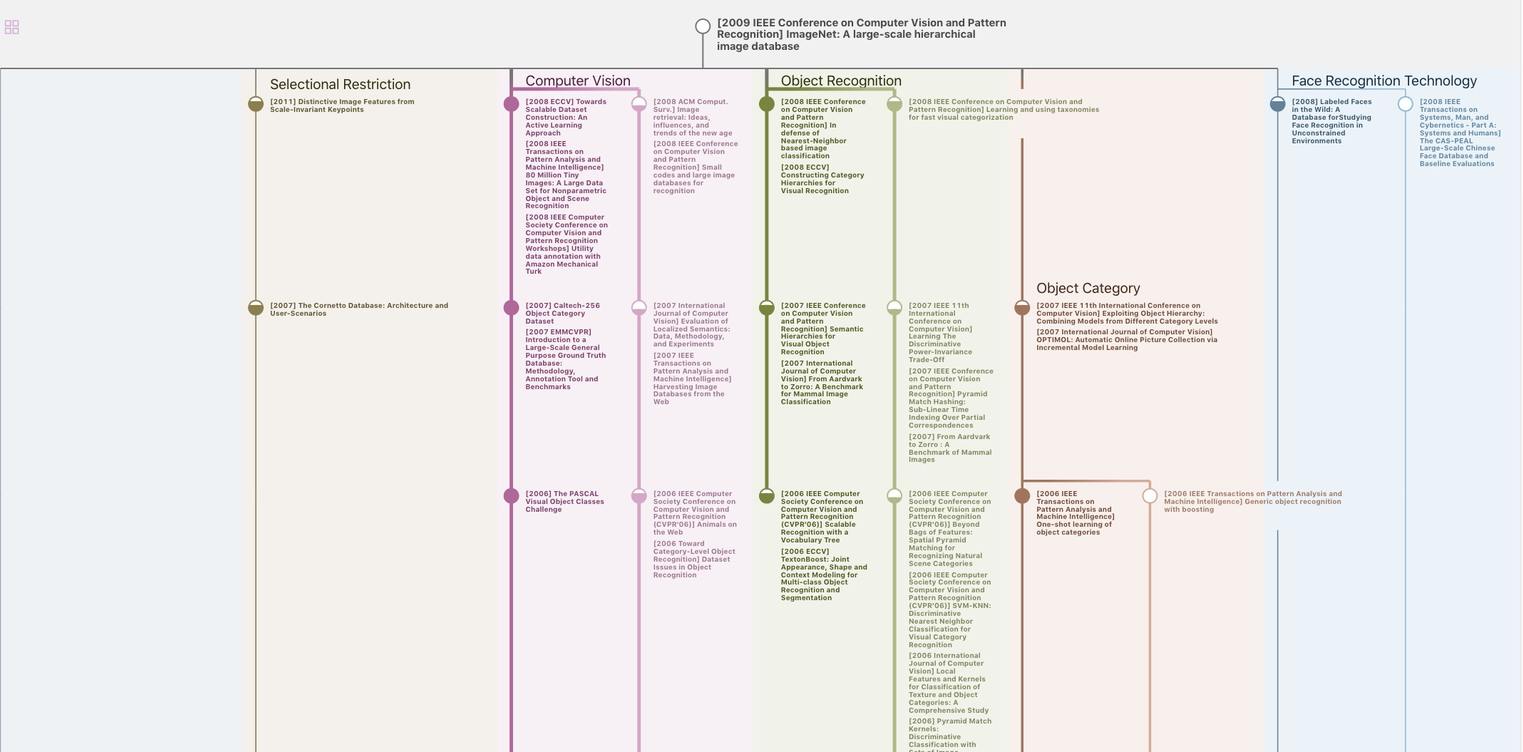
生成溯源树,研究论文发展脉络
Chat Paper
正在生成论文摘要