EnTri: Ensemble Learning with Tri-level Representations for Explainable Scene Recognition
CoRR(2023)
摘要
Scene recognition based on deep-learning has made significant progress, but there are still limitations in its performance due to challenges posed by inter-class similarities and intra-class dissimilarities. Furthermore, prior research has primarily focused on improving classification accuracy, yet it has given less attention to achieving interpretable, precise scene classification. Therefore, we are motivated to propose EnTri, an ensemble scene recognition framework that employs ensemble learning using a hierarchy of visual features. EnTri represents features at three distinct levels of detail: pixel-level, semantic segmentation-level, and object class and frequency level. By incorporating distinct feature encoding schemes of differing complexity and leveraging ensemble strategies, our approach aims to improve classification accuracy while enhancing transparency and interpretability via visual and textual explanations. To achieve interpretability, we devised an extension algorithm that generates both visual and textual explanations highlighting various properties of a given scene that contribute to the final prediction of its category. This includes information about objects, statistics, spatial layout, and textural details. Through experiments on benchmark scene classification datasets, EnTri has demonstrated superiority in terms of recognition accuracy, achieving competitive performance compared to state-of-the-art approaches, with an accuracy of 87.69%, 75.56%, and 99.17% on the MIT67, SUN397, and UIUC8 datasets, respectively.
更多查看译文
AI 理解论文
溯源树
样例
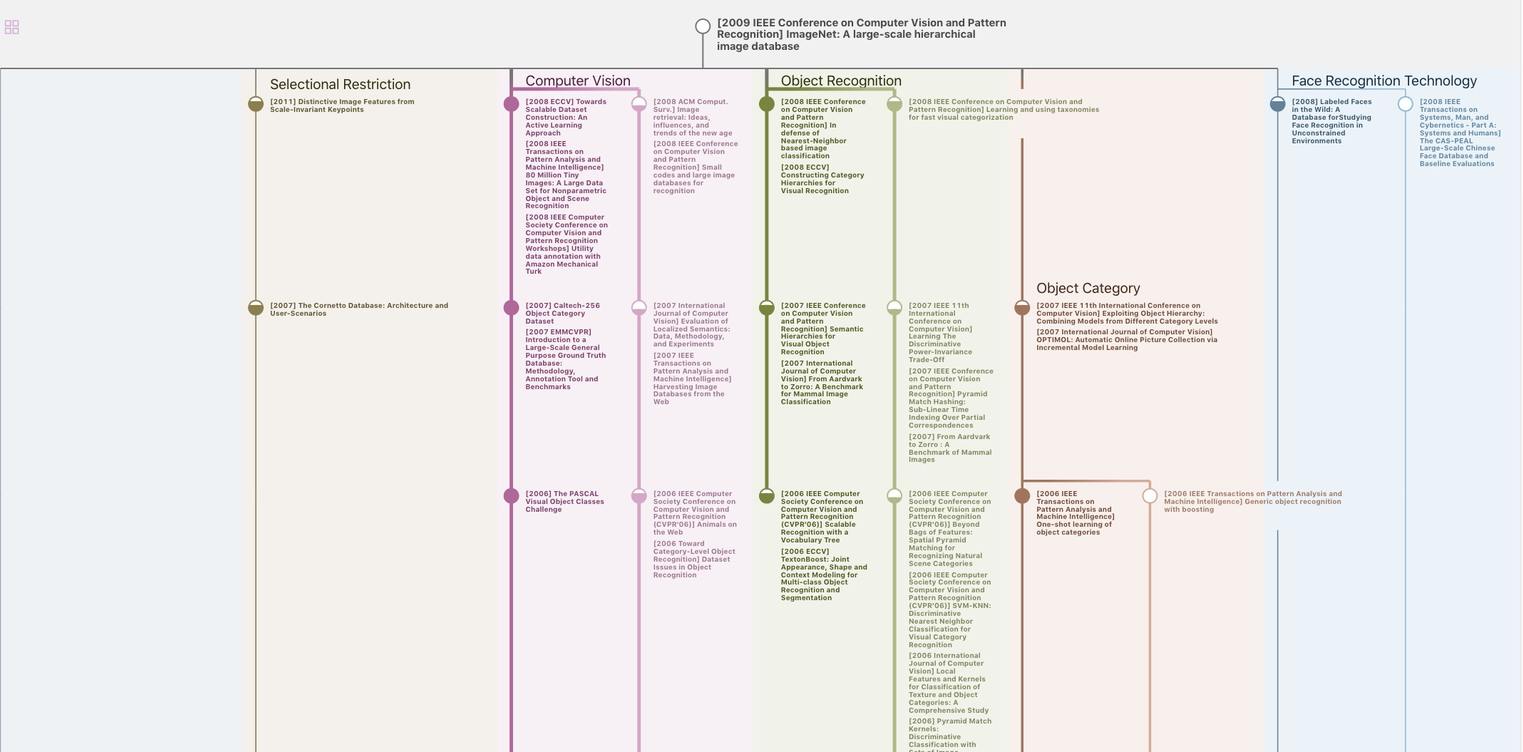
生成溯源树,研究论文发展脉络
Chat Paper
正在生成论文摘要