A Generalized Schwarz-type Non-overlapping Domain Decomposition Method using Physics-constrained Neural Networks
CoRR(2023)
摘要
We present a meshless Schwarz-type non-overlapping domain decomposition method based on artificial neural networks for solving forward and inverse problems involving partial differential equations (PDEs). To ensure the consistency of solutions across neighboring subdomains, we adopt a generalized Robin-type interface condition, assigning unique Robin parameters to each subdomain. These subdomain-specific Robin parameters are learned to minimize the mismatch on the Robin interface condition, facilitating efficient information exchange during training. Our method is applicable to both the Laplace's and Helmholtz equations. It represents local solutions by an independent neural network model which is trained to minimize the loss on the governing PDE while strictly enforcing boundary and interface conditions through an augmented Lagrangian formalism. A key strength of our method lies in its ability to learn a Robin parameter for each subdomain, thereby enhancing information exchange with its neighboring subdomains. We observe that the learned Robin parameters adapt to the local behavior of the solution, domain partitioning and subdomain location relative to the overall domain. Extensive experiments on forward and inverse problems, including one-way and two-way decompositions with crosspoints, demonstrate the versatility and performance of our proposed approach.
更多查看译文
关键词
neural networks,decomposition,schwarz-type,non-overlapping,physics-constrained
AI 理解论文
溯源树
样例
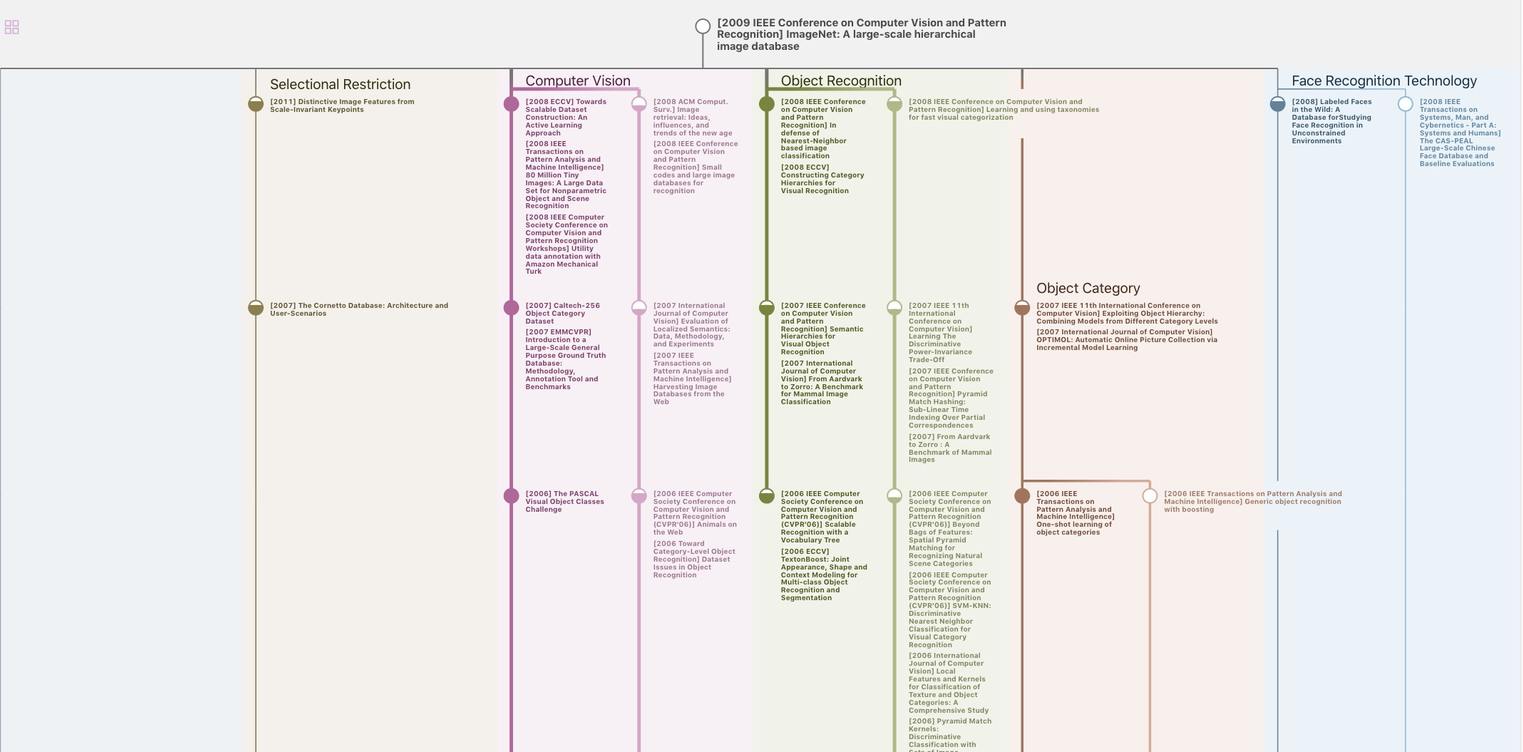
生成溯源树,研究论文发展脉络
Chat Paper
正在生成论文摘要