Collaboratively Learning Linear Models with Structured Missing Data
arXiv (Cornell University)(2023)
摘要
We study the problem of collaboratively learning least squares estimates for $m$ agents. Each agent observes a different subset of the features$\unicode{x2013}$e.g., containing data collected from sensors of varying resolution. Our goal is to determine how to coordinate the agents in order to produce the best estimator for each agent. We propose a distributed, semi-supervised algorithm Collab, consisting of three steps: local training, aggregation, and distribution. Our procedure does not require communicating the labeled data, making it communication efficient and useful in settings where the labeled data is inaccessible. Despite this handicap, our procedure is nearly asymptotically local minimax optimal$\unicode{x2013}$even among estimators allowed to communicate the labeled data such as imputation methods. We test our method on real and synthetic data.
更多查看译文
关键词
linear models,learning,data
AI 理解论文
溯源树
样例
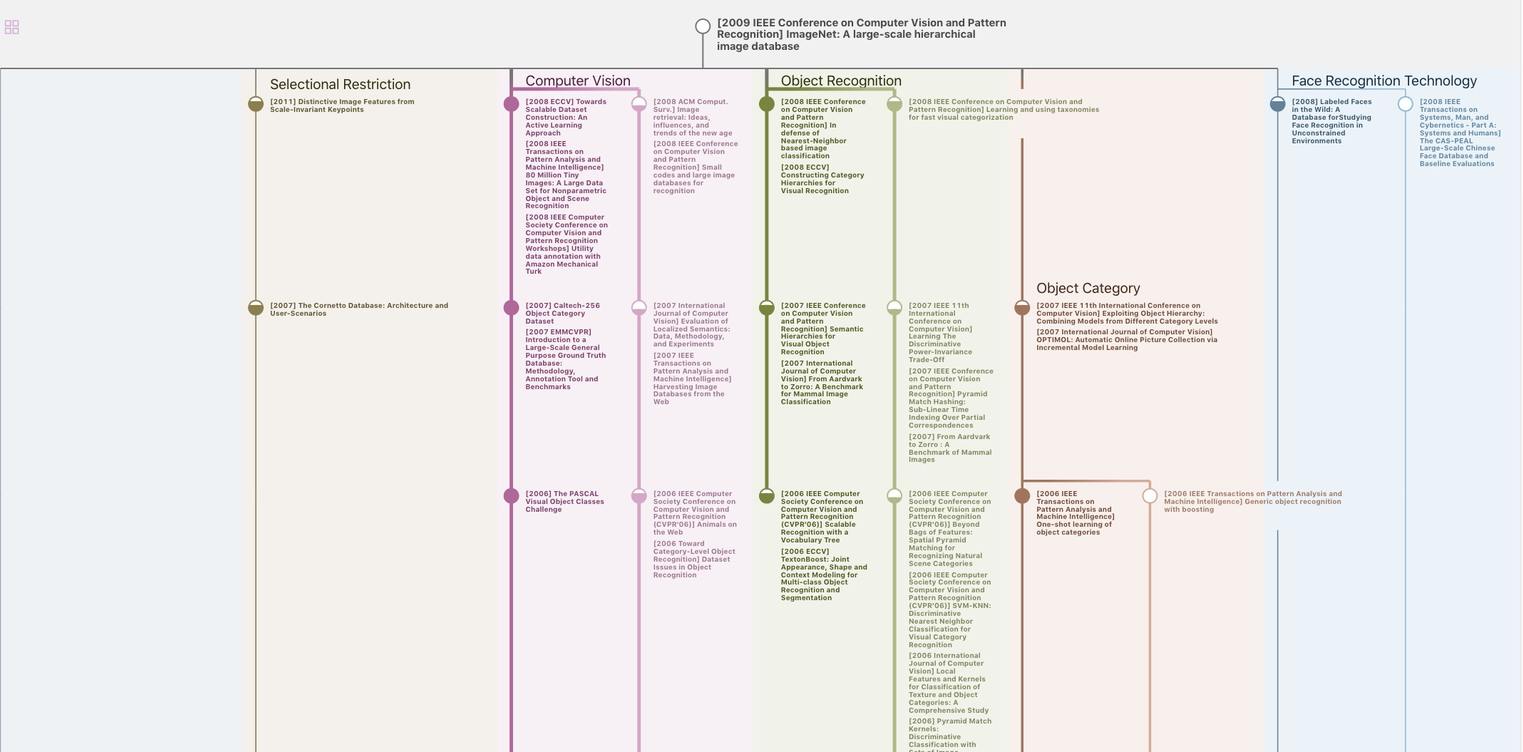
生成溯源树,研究论文发展脉络
Chat Paper
正在生成论文摘要