Bayesian Design Principles for Frequentist Sequential Learning
ICML 2023(2023)
摘要
We develop a general theory to optimize the frequentist regret for sequential learning problems, where efficient bandit and reinforcement learning algorithms can be derived from unified Bayesian principles. We propose a novel optimization approach to create "algorithmic beliefs" at each round, and use Bayesian posteriors to make decisions. This is the first approach to make Bayesian-type algorithms prior-free and applicable to adversarial settings, in a generic and optimal manner. Moreover, the algorithms are simple and often efficient to implement. As a major application, we present a novel algorithm for multi-armed bandits that achieves the "best-of-all-worlds" empirical performance in the stochastic, adversarial, and non-stationary environments. And we illustrate how these principles can be used in linear bandits, convex bandits, and reinforcement learning.
更多查看译文
关键词
learning,design
AI 理解论文
溯源树
样例
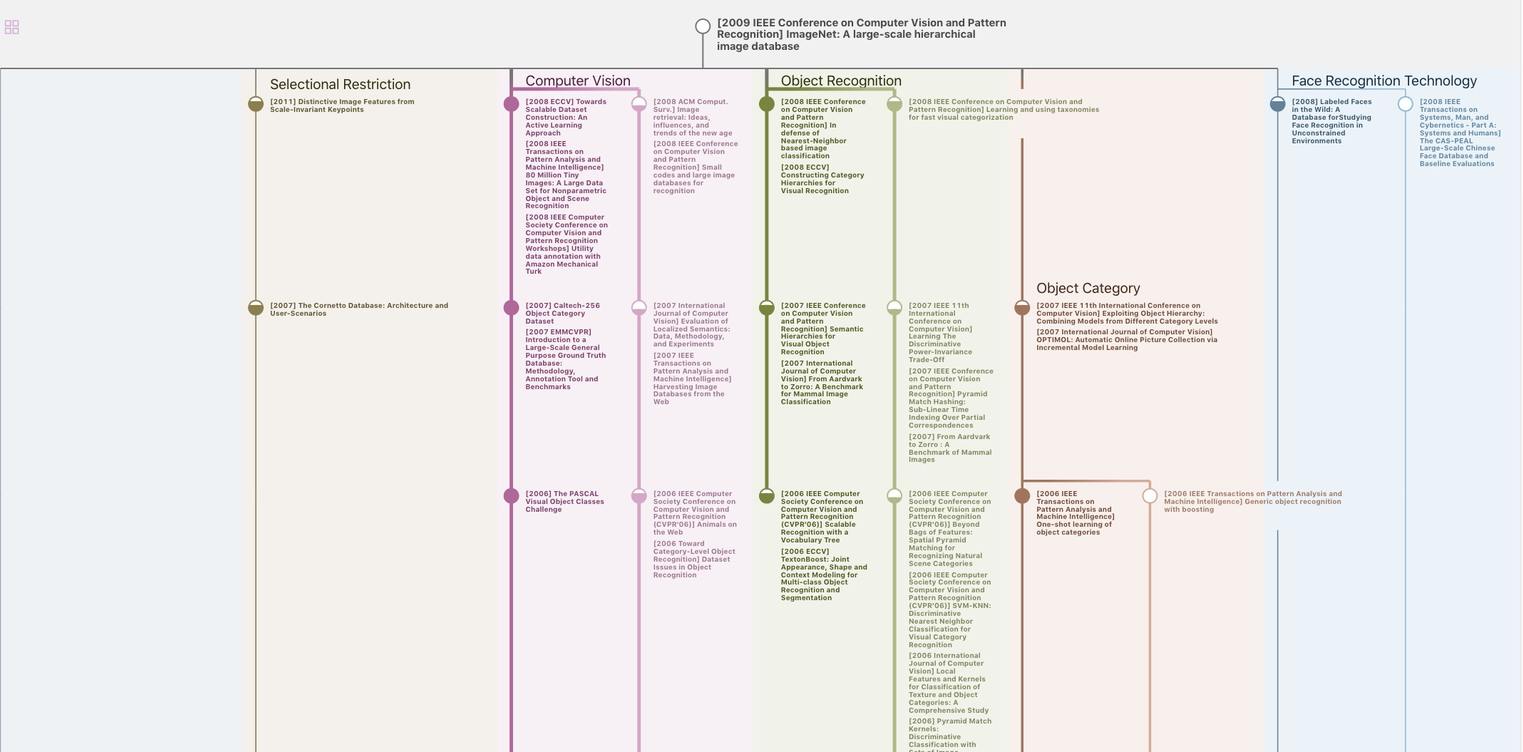
生成溯源树,研究论文发展脉络
Chat Paper
正在生成论文摘要