Optimal Shrinkage for Distributed Second-Order Optimization
ICML 2023(2024)
摘要
In this work, we address the problem of Hessian inversion bias in distributed
second-order optimization algorithms. We introduce a novel shrinkage-based
estimator for the resolvent of gram matrices which is asymptotically unbiased,
and characterize its non-asymptotic convergence rate in the isotropic case. We
apply this estimator to bias correction of Newton steps in distributed
second-order optimization algorithms, as well as randomized sketching based
methods. We examine the bias present in the naive averaging-based distributed
Newton's method using analytical expressions and contrast it with our proposed
bias-free approach. Our approach leads to significant improvements in
convergence rate compared to standard baselines and recent proposals, as shown
through experiments on both real and synthetic datasets.
更多查看译文
AI 理解论文
溯源树
样例
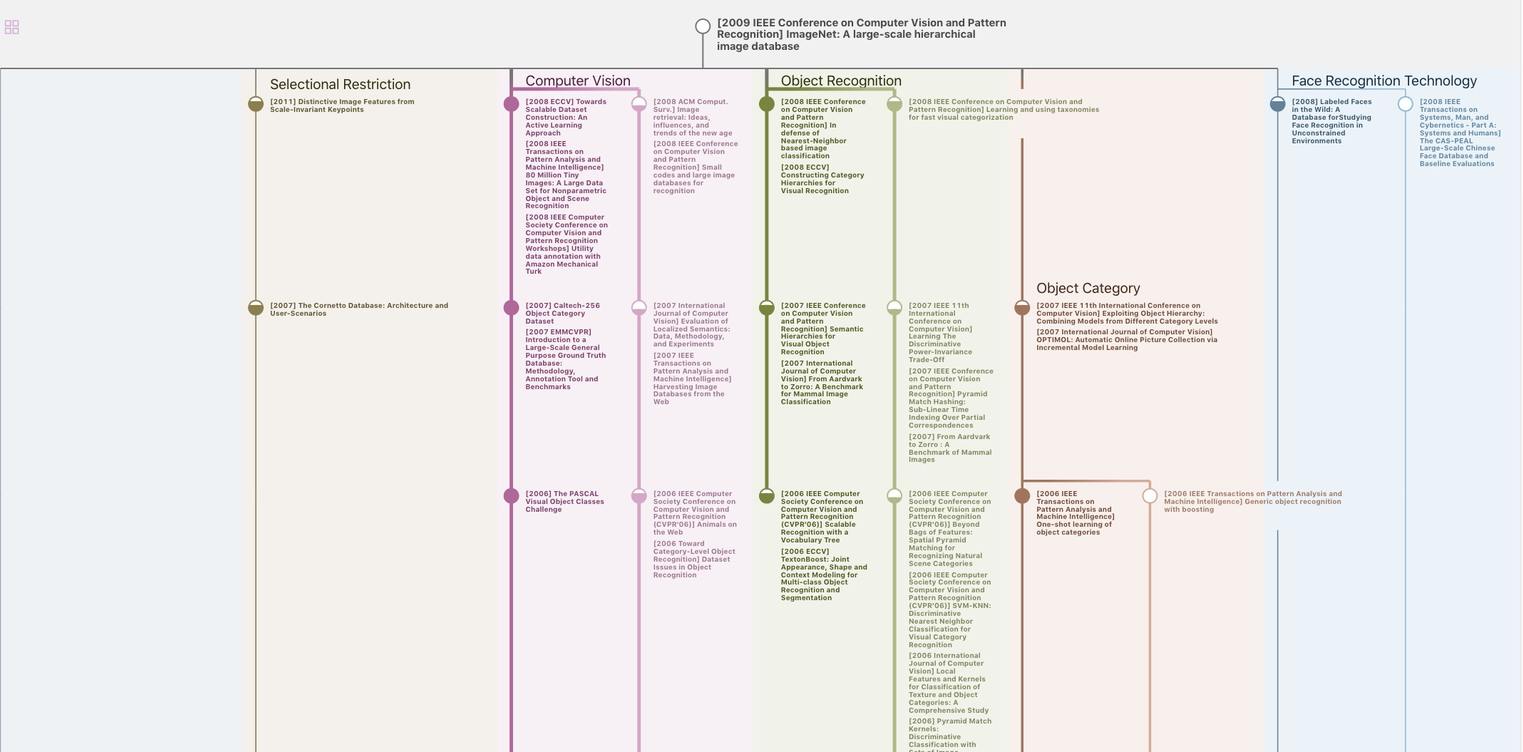
生成溯源树,研究论文发展脉络
Chat Paper
正在生成论文摘要