Topological Singularity Detection at Multiple Scales
ICML 2023(2023)
摘要
The manifold hypothesis, which assumes that data lies on or close to an unknown manifold of low intrinsic dimension, is a staple of modern machine learning research. However, recent work has shown that real-world data exhibits distinct non-manifold structures, i.e. singularities, that can lead to erroneous findings. Detecting such singularities is therefore crucial as a precursor to interpolation and inference tasks. We address this issue by developing a topological framework that (i) quantifies the local intrinsic dimension, and (ii) yields a Euclidicity score for assessing the 'manifoldness' of a point along multiple scales. Our approach identifies singularities of complex spaces, while also capturing singular structures and local geometric complexity in image data.
更多查看译文
关键词
topological singularity detection,scales
AI 理解论文
溯源树
样例
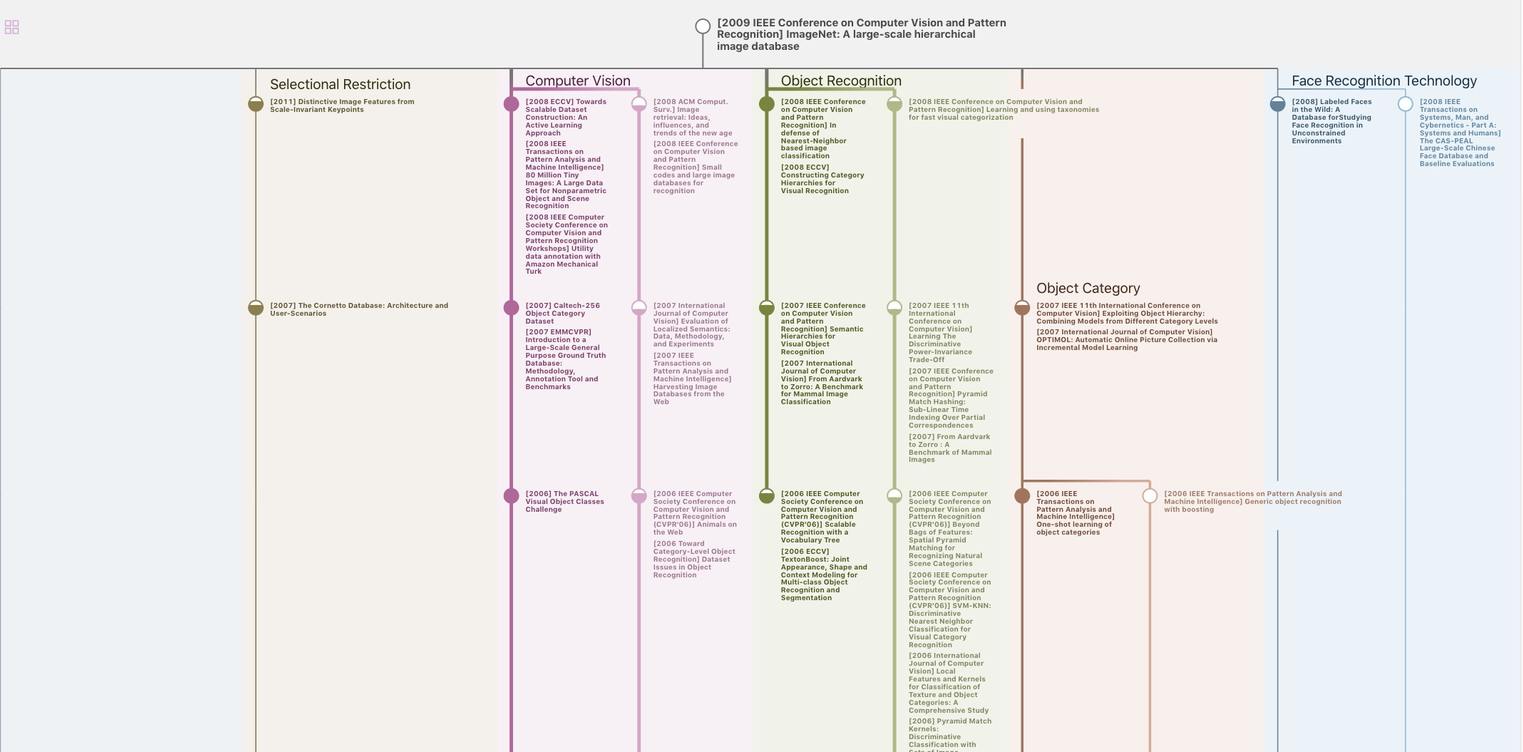
生成溯源树,研究论文发展脉络
Chat Paper
正在生成论文摘要