Margin-based sampling in high dimensions: When being active is less efficient than staying passive
ICML 2023(2023)
摘要
It is widely believed that given the same labeling budget, active learning (AL) algorithms like margin-based active learning achieve better predictive performance than passive learning (PL), albeit at a higher computational cost. Recent empirical evidence suggests that this added cost might be in vain, as margin-based AL can sometimes perform even worse than PL. While existing works offer different explanations in the low-dimensional regime, this paper shows that the underlying mechanism is entirely different in high dimensions: we prove for logistic regression that PL outperforms margin-based AL even for noiseless data and when using the Bayes optimal decision boundary for sampling. Insights from our proof indicate that this high-dimensional phenomenon is exacerbated when the separation between the classes is small. We corroborate this intuition with experiments on 20 high-dimensional datasets spanning a diverse range of applications, from finance and histology to chemistry and computer vision.
更多查看译文
关键词
sampling,margin-based
AI 理解论文
溯源树
样例
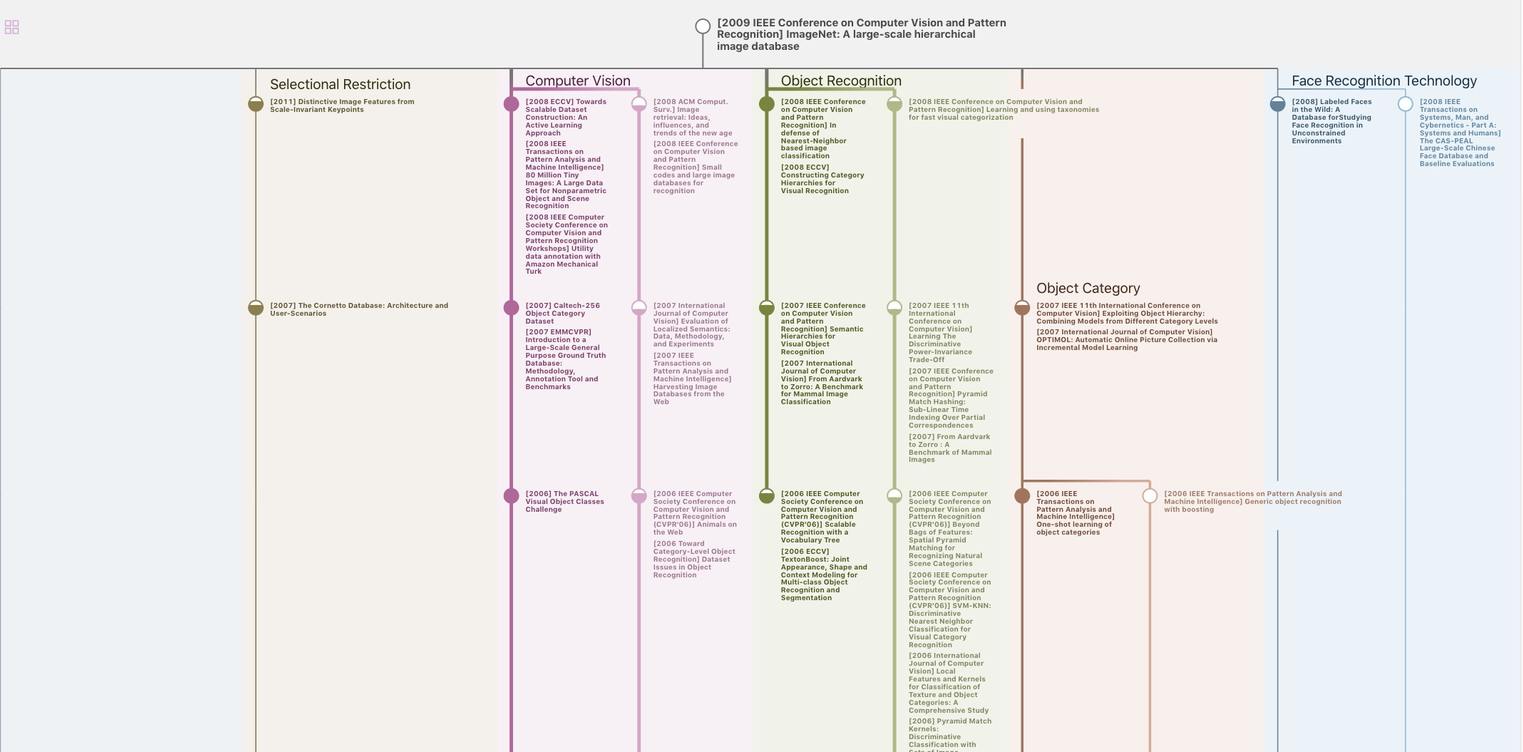
生成溯源树,研究论文发展脉络
Chat Paper
正在生成论文摘要