Differentially Private Heavy Hitter Detection using Federated Analytics
CoRR(2023)
摘要
In this work, we study practical heuristics to improve the performance of prefix-tree based algorithms for differentially private heavy hitter detection. Our model assumes each user has multiple data points and the goal is to learn as many of the most frequent data points as possible across all users' data with aggregate and local differential privacy. We propose an adaptive hyperparameter tuning algorithm that improves the performance of the algorithm while satisfying computational, communication and privacy constraints. We explore the impact of different data-selection schemes as well as the impact of introducing deny lists during multiple runs of the algorithm. We test these improvements using extensive experimentation on the Reddit dataset~\cite{caldas2018leaf} on the task of learning the most frequent words.
更多查看译文
关键词
private heavy hitter detection,federated analytics
AI 理解论文
溯源树
样例
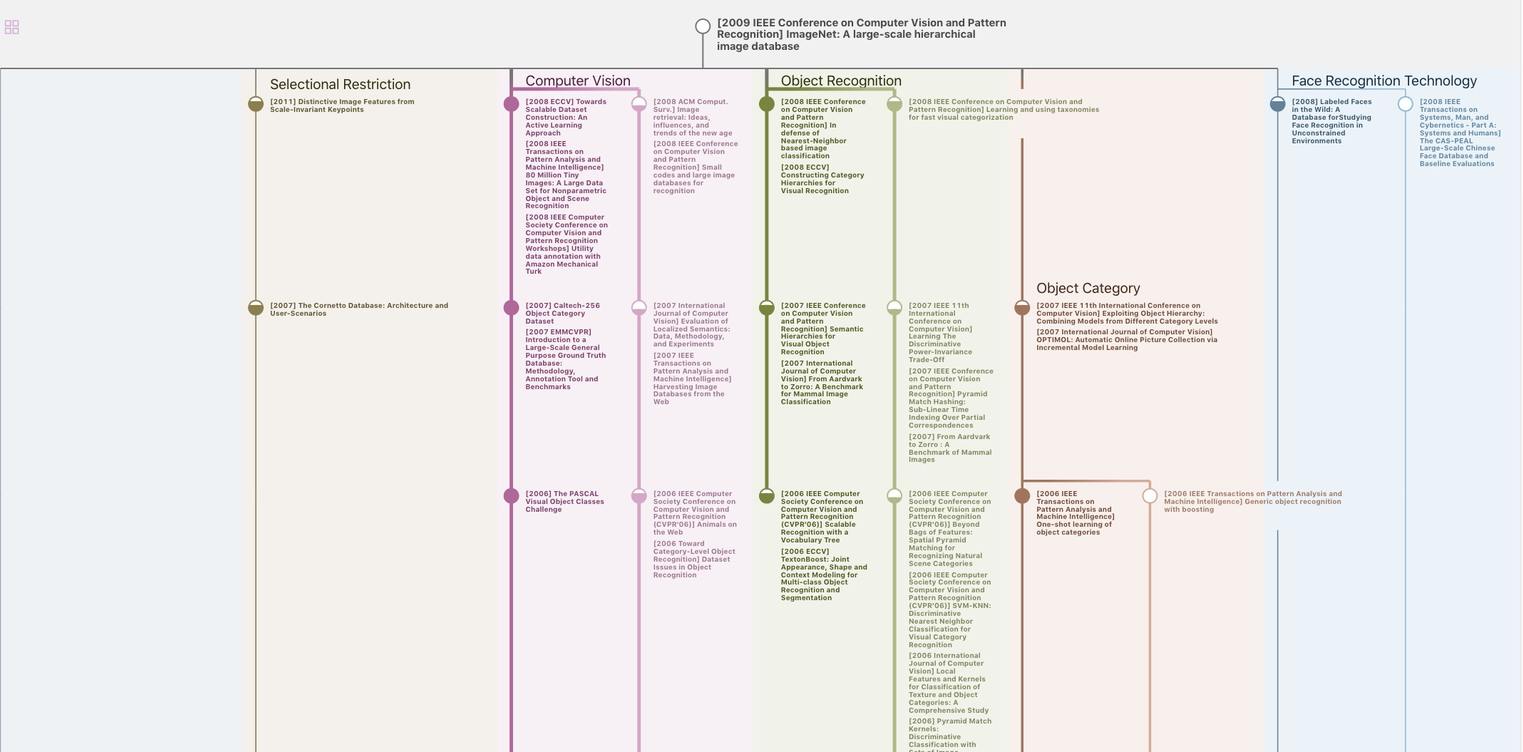
生成溯源树,研究论文发展脉络
Chat Paper
正在生成论文摘要