SACReg: Scene-Agnostic Coordinate Regression for Visual Localization
CoRR(2023)
摘要
Scene coordinates regression (SCR), i.e., predicting 3D coordinates for every pixel of a given image, has recently shown promising potential. However, existing methods remain mostly scene-specific or limited to small scenes and thus hardly scale to realistic datasets. In this paper, we propose a new paradigm where a single generic SCR model is trained once to be then deployed to new test scenes, regardless of their scale and without further finetuning. For a given query image, it collects inputs from off-the-shelf image retrieval techniques and Structure-from-Motion databases: a list of relevant database images with sparse pointwise 2D-3D annotations. The model is based on the transformer architecture and can take a variable number of images and sparse 2D-3D annotations as input. It is trained on a few diverse datasets and significantly outperforms other scene regression approaches on several benchmarks, including scene-specific models, for visual localization. In particular, we set a new state of the art on the Cambridge localization benchmark, even outperforming feature-matching-based approaches.
更多查看译文
关键词
visual localization,sacreg,scene-agnostic
AI 理解论文
溯源树
样例
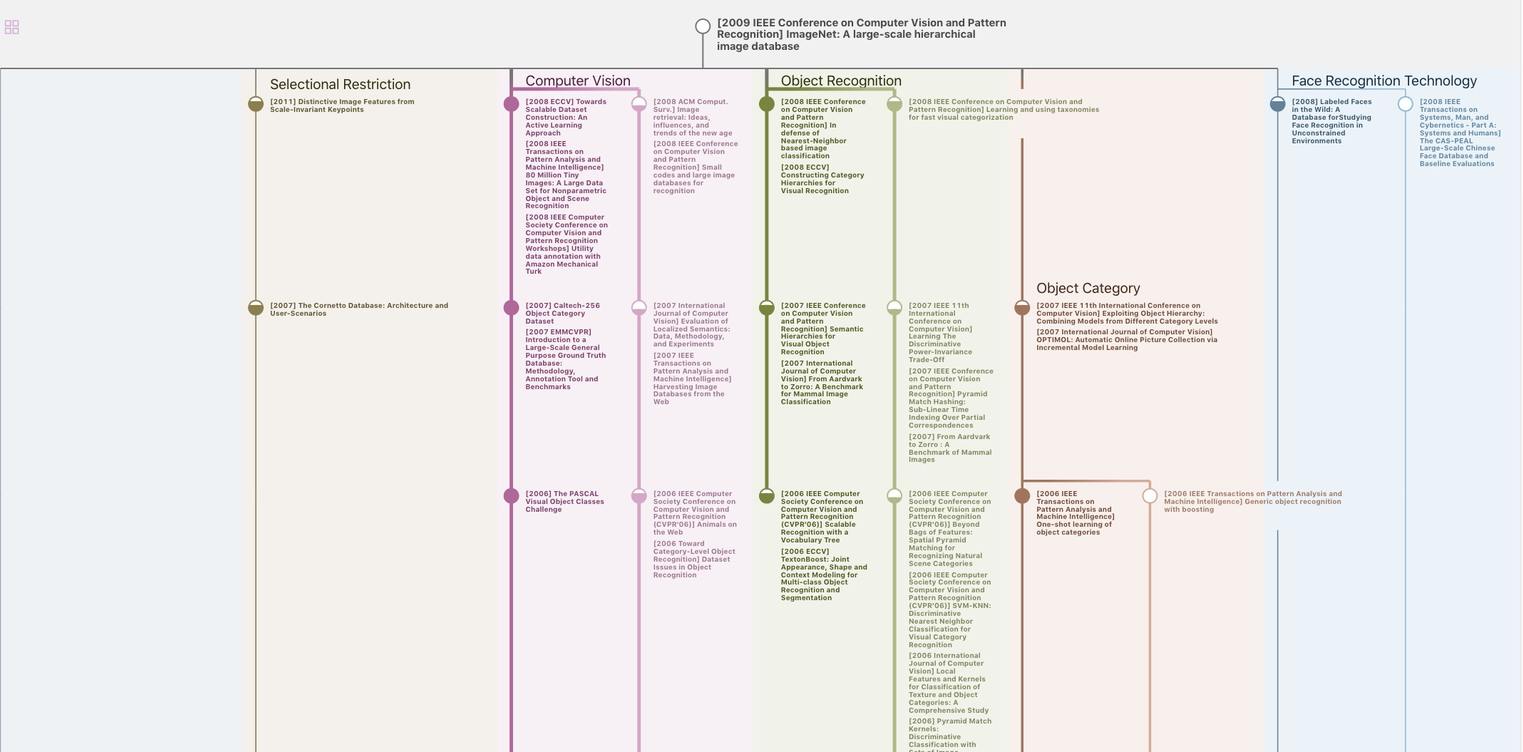
生成溯源树,研究论文发展脉络
Chat Paper
正在生成论文摘要