Fast Adaptive Test-Time Defense with Robust Features
CoRR(2023)
摘要
Adaptive test-time defenses are used to improve the robustness of deep neural networks to adversarial examples. However, existing methods significantly increase the inference time due to additional optimization on the model parameters or the input at test time. In this work, we propose a novel adaptive test-time defense strategy that is easy to integrate with any existing (robust) training procedure without additional test-time computation. Based on the notion of robustness of features that we present, the key idea is to project the trained models to the most robust feature space, thereby reducing the vulnerability to adversarial attacks in non-robust directions. We theoretically show that the top eigenspace of the feature matrix are more robust for a generalized additive model and support our argument for a large width neural network with the Neural Tangent Kernel (NTK) equivalence. We conduct extensive experiments on CIFAR-10 and CIFAR-100 datasets for several robustness benchmarks, including the state-of-the-art methods in RobustBench, and observe that the proposed method outperforms existing adaptive test-time defenses at much lower computation costs.
更多查看译文
关键词
defense,adaptive,test-time
AI 理解论文
溯源树
样例
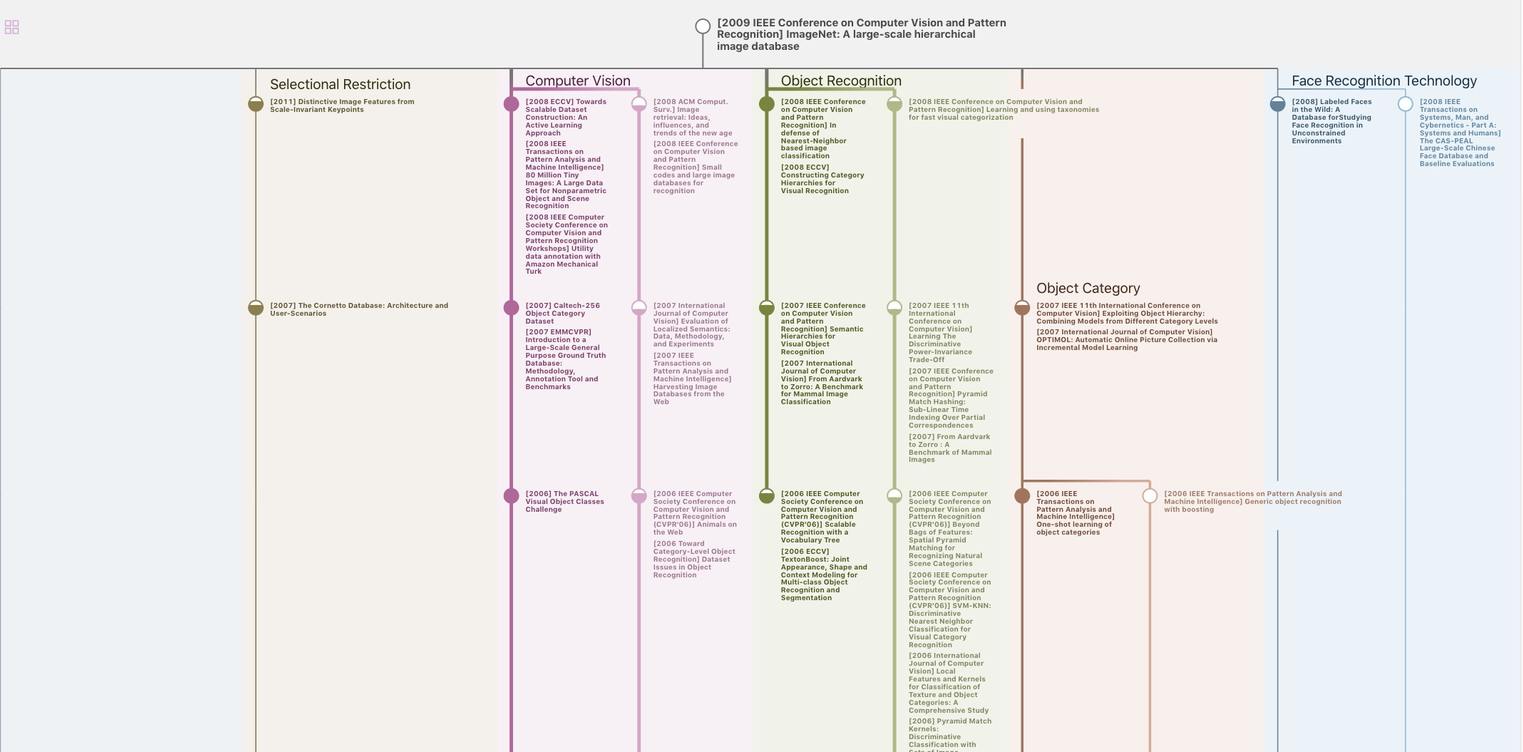
生成溯源树,研究论文发展脉络
Chat Paper
正在生成论文摘要