Discovering Active Subspaces for High-Dimensional Computer Models
JOURNAL OF COMPUTATIONAL AND GRAPHICAL STATISTICS(2023)
Abstract
Dimension reduction techniques have long been an important topic in statistics, and active subspaces (AS) have received much attention this past decade in the computer experiments literature. The most common approach toward estimating the AS is to use Monte Carlo with numerical gradient evaluation. While sensible in some settings, this approach has obvious drawbacks. Recent research has demonstrated that active subspace calculations can be obtained in closed form, conditional on a Gaussian process (GP) surrogate, which can be limiting in high-dimensional settings for computational reasons. In this article, we produce the relevant calculations for a more general case when the model of interest is a linear combination of tensor products. These general equations can be applied to the GP, recovering previous results as a special case, or applied to the models constructed by other regression techniques including multivariate adaptive regression splines (MARS). Using a MARS surrogate has many advantages including improved scaling, better estimation of active subspaces in high dimensions and the ability to handle a large number of prior distributions in closed form. In one real-world example, we obtain the active subspace of a radiation-transport code with 240 inputs and 9372 model runs in under half an hour. Supplementary materials for this article are available online.
MoreTranslated text
Key words
Bayesian MARS,Computer experiments,Dimension reduction,Nonparametric regression,Sensitivity analysis
AI Read Science
Must-Reading Tree
Example
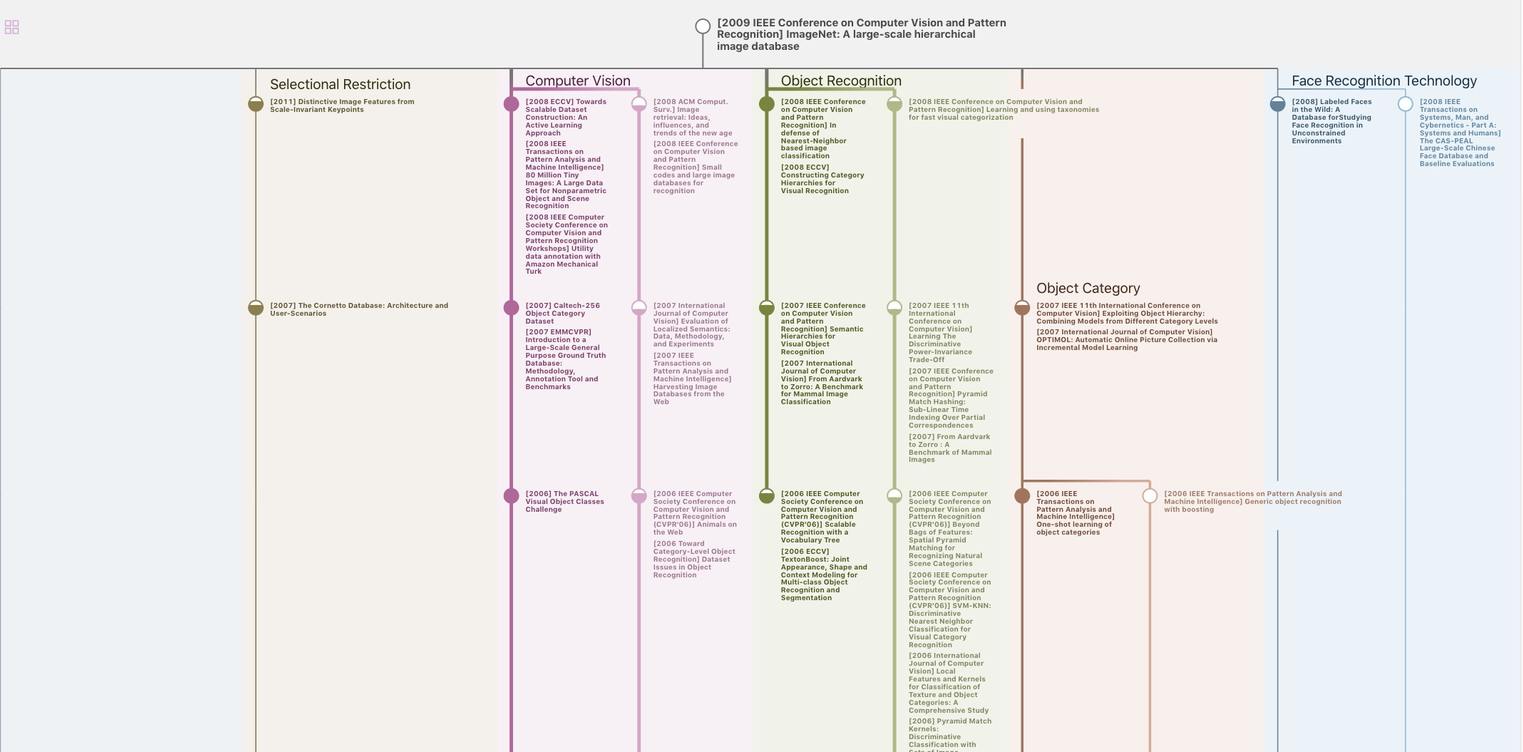
Generate MRT to find the research sequence of this paper
Chat Paper
Summary is being generated by the instructions you defined