A heuristic explicit model predictive control framework for Eco-trajectory planning: Theoretical analysis and case study
arxiv(2023)
摘要
The trajectory planning problem (TPP) has become increasingly crucial in the research of next-generation transportation systems, but it presents challenges due to the non-linearity of its constraints. One specific case within TPP, namely the Eco-trajectory Planning Problem (EPP), poses even greater computational difficulties due to its nonlinear, high-order, and non-convex objective function. This paper proposes a heuristic explicit predictive model control (heMPC) framework to address the eco-trajectory planning problem in scenarios without lane-changing behavior. The heMPC framework consists of an offline module and an online module. In the offline module, we build an optimal eco-trajectory batch by optimizing a series of simplified EPPs considering different system initial states and terminal states, which is equivalent to the lookup table in the general eMPC framework. The core idea of the offline module is to finish all potential optimization and computing in advance to avoid any form of online optimization in the online module. In the online module, we provide static and dynamic trajectory planning algorithms. Both algorithms greatly improve the computational efficiency of planning and only suffer from a limited extent of optimality losses through a batch-based selection process because any optimization and calculation are pre-computed in the offline module. The latter algorithm is also able to face possible emergencies and prediction errors. Both theoretical analysis and numerical are shown and discussed to test the computational quality and efficiency of the heMPC framework under a mixed-traffic flow environment that incorporates human-driving vehicles (HDV) and connected and automated vehicles (CAV) with different market penetration rates (MPR).
更多查看译文
AI 理解论文
溯源树
样例
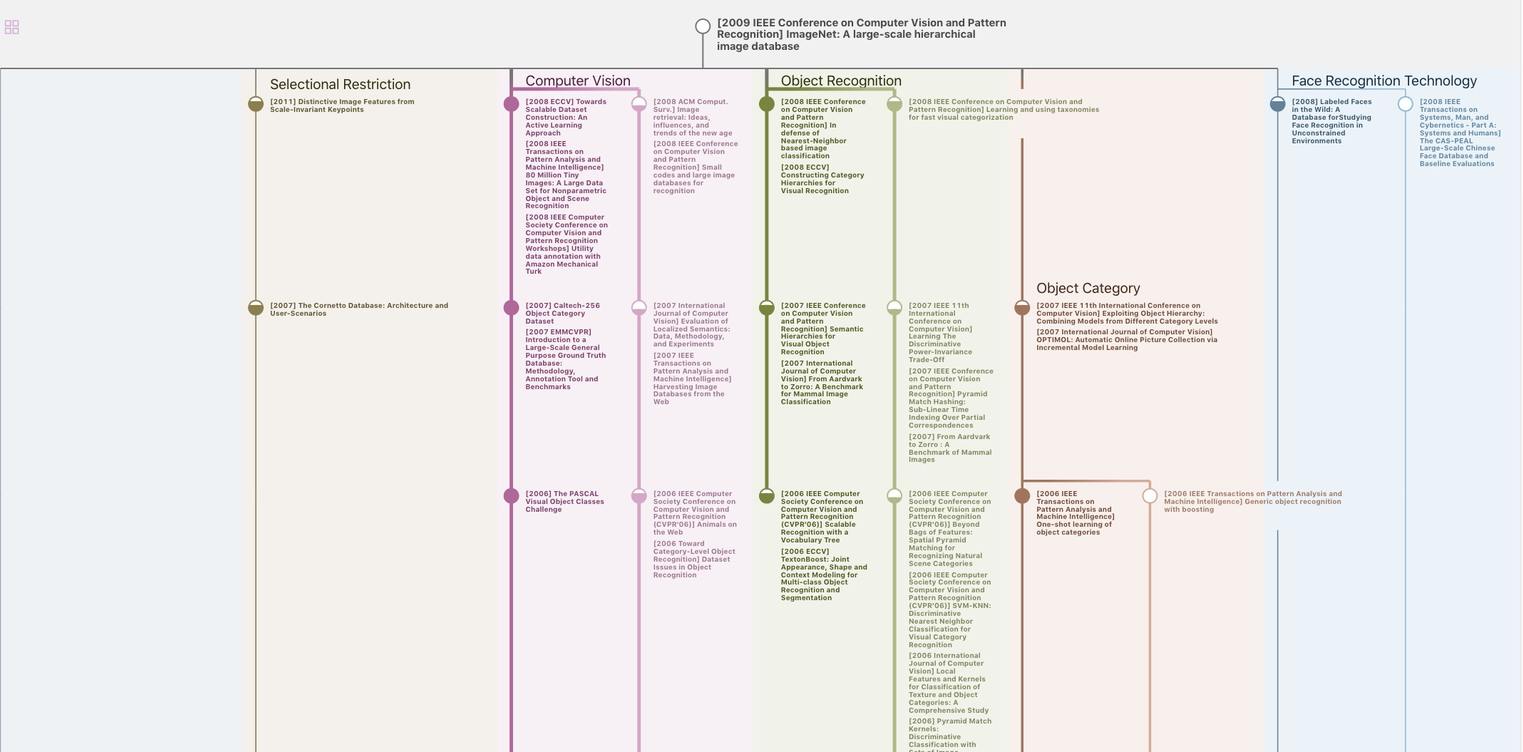
生成溯源树,研究论文发展脉络
Chat Paper
正在生成论文摘要